According to a new study performed at UTHealth Houston, the implementation of artificial intelligence-powered large vessel occlusion (LVO) detection software available for acute stroke triage could help enhance endovascular thrombectomy treatment times.
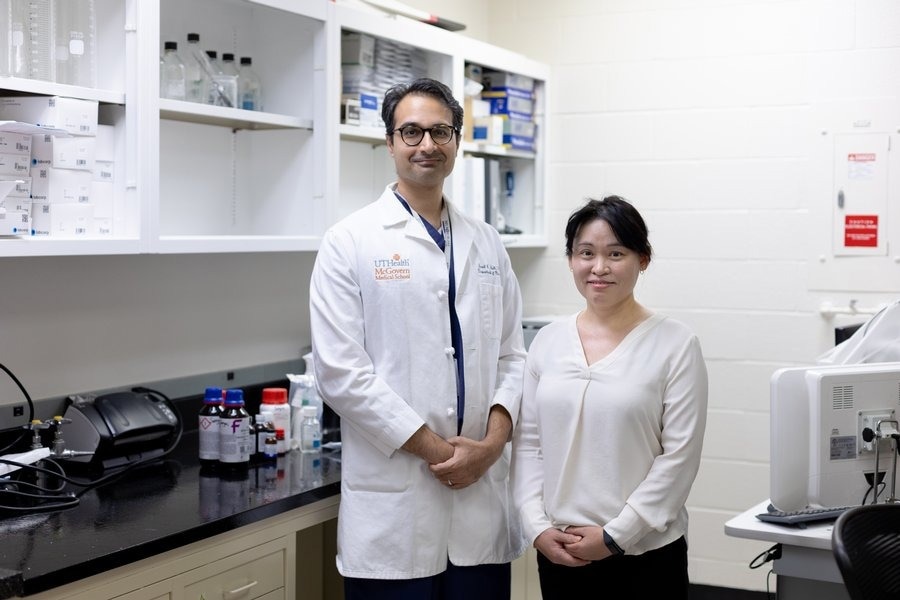
Study authors Sunil A. Sheth, MD, with McGovern Medical School at UTHealth Houston, and Youngran Kim, PhD, with UTHealth Houston School of Public Health, in the lab. Image Credit: Rogelio Castro/UTHealth Houston
The study was recently reported in JAMA Neurology and was headed by co-first authors Youngran Kim, Ph.D., Assistant Professor of Management, Policy, and Community Health with UTHealth Houston School of Public Health, and Juan Carlos Martinez-Gutierrez, MD, a former surgery fellow in the Vivian L. Smith Department of Neurosurgery with McGovern Medical School at UTHealth Houston.
Co-senior authors Sunil A. Sheth MD, Associate Professor of Neurology and Director of the Vascular Neurology Program with McGovern Medical School, and Luca Giancardo, Ph.D., Associate Professor at McWilliams School of Biomedical Informatics at UTHealth Houston, also involved in the study.
LVO happens when there is a blockage in a major artery in the brain. Regarded as one of the most severe kinds of strokes, an LVO accounts for an evaluated 24% to 46% of acute ischemic strokes.
Prompt endovascular thrombectomy, known to be a minimally invasive surgical procedure involving the elimination of a blood clot from a blocked artery in the brain, could considerably enhance outcomes in patients with LVO acute ischemic stroke, but its efficacy is highly time-sensitive.
The benefit of endovascular thrombectomy on functional recovery is time-sensitive, so early identification of patients with strokes with large vessel occlusions, and process improvements to accelerate in-hospital care, are critical. Our study has shown that the implementation of AI software has improved workflows within the comprehensive stroke centers.
Youngran Kim Ph.D., Houston School of Public Health
To check how to enhance in-hospital endovascular therapy workflows, scientists performed a cluster-randomized, stepped-wedge clinical trial from January 1st, 2021, to Feb. 27th, 2022, examining 243 patients with LVO stroke who presented at four comprehensive stroke centers in the Greater Houston area.
A system called Viz LVO, which utilizes artificial intelligence to automatically detect Large Vessel Occlusion (LVO) from computed tomography (CT) angiograms, was implemented at four different sites in a randomized manner.
When activated, this system sent immediate alerts to clinicians and radiologists via their mobile phones, notifying them about potential LVO cases within minutes of completing the CT imaging. Additionally, the patient's treatment team could share information on cases in real time using this system.
Crucially, the implementation of automated LVO detection software resulted in patients experiencing, on average, a statistically considerable decrease of 11 minutes in time to thrombectomy initiation. Also, the time taken from CT scan initiation to the start of endovascular therapy fell by almost 10 minutes.
We are just at the beginning of automated machine-learning algorithms to benefit acute stroke care. Many other applications are being developed, such as using CT angiograms to detect infarcted areas of the brain without advanced imaging, an NIH-funded effort by our research group which we recently published, or even the use of retina imaging as a proxy for brain imaging.
Luca Giancardo, Associate Professor, McWilliams School of Biomedical Informatics, University of Texas Health Science Center at Houston
The study outcomes come shortly following the publication of another study headed by Kim, Sheth, and other UTHealth Houston scientists, which discovered that, despite having worse stroke symptoms and living within equivalent distances to extensive stroke centers, women with LVO acute ischemic stroke were less probable to be routed to the centers than to men.
Both studies are considered to be a part of an extensive measure to find ways to enhance stroke outcomes.
Nearly 2 million brain cells die every minute the blockage remains, so speeding up treatments by 10 to 15 minutes can result in substantial improvements. Our study is the most rigorous of its kind to address the question of whether machine-learning software can result in a clinically meaningful improvement for patients with acute stroke, and here, we see that the answer is ‘yes.’
Sunil A. Sheth MD, Study Author, University of Texas Health Science Center at Houston
The other co-authors of the study with McGovern Medical School included Sergio Salazar-Marioni, MD; Muhammad Bilal Tariq, MD; Rania Abdelkhaleq, MPH; Arash Niktabe, BS; Anjan N. Ballekere, MS; Ananya Iyyangar, BS; Mai Le, BS; Hussain Azeem, BS; Charles Miller, PhD; Jon Tyson, MD, MPH; Sandi Shaw, RN; Peri Smith, RN; Mallory Cowan, RN; Isabel Gonzales, RN;
The additional authors are Louise McCullough, MD, Ph.D., and Andrew Barreto, MD. Abdelkhaleq and Le are also with UTHealth Houston School of Public Health, and McCullough and Giancardo are also with The University of Texas MD Anderson Cancer Center UTHealth Houston Graduate School of Biomedical Sciences.
Journal Reference
Martinez-Gutierrez, J. C., et al. (2023) Automated Large Vessel Occlusion Detection Software and Thrombectomy Treatment Times. JAMA Neurology. doi.org/10.1001/jamaneurol.2023.3206.