According to a new study published in Radiology, AI systems for short- and long-term breast cancer risk could result in an improved cancer risk assessment.
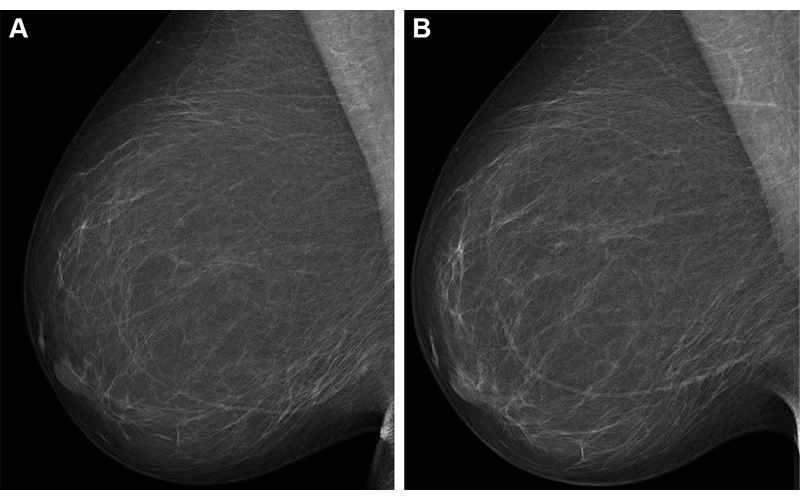
Full-field digital mammograms (right mediolateral oblique view) in a 59-year-old woman show (A) the screening mammogram obtained during the study period and (B) the screening mammogram obtained in the subsequent screening round. The first screening mammogram (A) had a very low combined risk score (lowest 0.1%) as determined by the combination model with texture risk and the examination score. The woman was not recalled and did not receive a breast cancer diagnosis throughout the 5-year follow-up. Image Credit: https://pubs.rsna.org/doi/10.1148/radiol.230227 ©RSNA 2023.
Using mammography-based deep learning models could help to enhance the precision of breast cancer risk assessment and thus result in earlier diagnoses.
About 1 in 10 women develop breast cancer throughout their lifetime. In recent years, AI has been studied for the purpose of diagnosing breast cancer earlier by automatically detecting breast cancers in mammograms and measuring the risk of future breast cancer.
Andreas D Lauritzen, PhD, Study Author, Department of Computer Science, University of Copenhagen
Diagnostic AI models have been trained to detect suspicious-looking lesions on mammograms and are thus adept at estimating short-term breast cancer risk.
More appropriate for long-term breast cancer risk assessment are texture AI models that can accurately identify breast density. Women with dense breast tissue face an elevated risk of developing breast cancer and could potentially gain advantages from additional MRI screening.
It is important to enable reliable and robust assessment of breast cancer risk using information from the screening mammogram.
Andreas D Lauritzen, PhD, Study Author, Department of Computer Science, University of Copenhagen
Risk Assessment Better for Interval and Long-Term Cancer Detection
As part of this study, Dr. Lauritzen and his research group sought to determine if a commercially available AI texture model and a diagnostic AI tool, trained separately and then later integrated, could enhance breast cancer risk assessment.
The researchers used the diagnostic AI tool Transpara and a texture model that was developed by the researchers. A Dutch training set of over 39,000 exams was used to train the models. The short- and long-term risk models were then combined using a three-layer neural network.
The integrated AI model was tested on a study group of over 119,000 women who were involved in a breast cancer screening program in the Capital Region of Denmark between November 2012 and December 2015. The average age of the women was 59 years.
Compared to the diagnostic and texture models, the integrated AI model displayed an overall enhanced risk assessment for interval and long-term cancer detection.
Furthermore, the model allowed for the identification of women at high risk for breast cancer. The integrated model determined women as having the 10% greatest combined risk reported for 33.7% of long-term cancers and 44.1% of interval cancers.
Utilizing AI to determine a woman’s breast cancer risk from a single mammogram will not only lead to earlier cancer detection but could also enhance the strain on the healthcare system as a result of the global shortage of specialized breast radiologists.
Current state-of-the-art clinical risk models require multiple tests such as blood work, genetic testing, mammogram and filling out extensive questionnaires, all of which would substantially increase the workload in the screening clinic.
Andreas D Lauritzen, PhD, Study Author, Department of Computer Science, University of Copenhagen
Lauritzen stated, “Using our model, risk can be assessed with the same performance as the clinical risk models but within seconds from screening and without introducing overhead in the clinic.”
Journal Reference:
Lauritzen, A. D., et al. (2023) Assessing Breast Cancer Risk by Combining AI for Lesion Detection and Mammographic Texture. Radiology. doi.org/10.1148/radiol.230227.