At the University of Toronto, scientists have come up with an artificial intelligence system that has the potential to make proteins not discovered in nature with the help of generative diffusion, the same technology behind famous image-creation platforms like Midjourney and DALL-E.
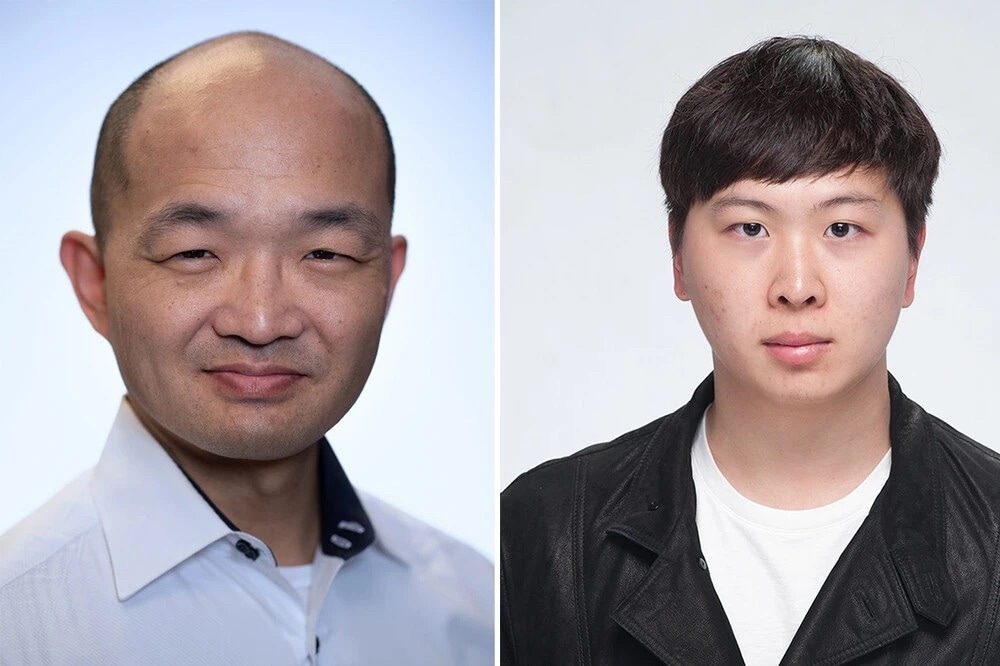
Professor Philip Kim and doctoral student Jin Sub (Michael) Lee. Image Credit: University of Toronto
The system will help progress the field of generative biology, which promises to expedite drug development by making the design and testing of completely new therapeutic proteins highly flexible and effective.
Our model learns from image representations to generate fully new proteins, at a very high rate. All our proteins appear to be biophysically real, meaning they fold into configurations that enable them to carry out specific functions within cells.
Philip M. Kim, Professor, Donnelly Center for Cellular and Biomolecular Research, Temerty Faculty of Medicine, University of Toronto
The journal Nature Computational Science reported the findings, the first of their kind in a peer-reviewed journal. Also, Kim’s lab published a pre-print on the model last summer via the open-access server bioRxiv, ahead of two identical pre-prints from last December, RF Diffusion by the University of Washington and Chroma by Generate Biomedicines.
Proteins are created from chains of amino acids that fold into three-dimensional shapes, which in return dictate the function of a protein. Those shapes developed over billions of years and are changed and complicated but also restricted in number. Having a better insight into how to present proteins fold, scientists have started to design folding patterns not generated in nature.
Kim states, however, a major difficulty has been envisioning folds that are both feasible and functional.
It’s been very hard to predict which folds will be real and work in a protein structure. By combining biophysics-based representations of protein structure with diffusion methods from the image generation space, we can begin to address this problem.
Philip M. Kim, Professor, Donnelly Center for Cellular and Biomolecular Research, Temerty Faculty of Medicine, University of Toronto
Kim is also a professor in the departments of molecular genetics and computer science at U of T.
The new system, which the scientists name ProteinSGM, withdraws from a large set of image-like representations of present proteins that encode their structure precisely. These images were fed by the scientists into a generative diffusion model, which slowly adds noise until every image turns out to be all noise.
The model tracks how the images turn out to be noisier and further runs the process in reverse. This teaches how to convert random pixels into clear images that match completely novel proteins.
Jin Sub (Michael) Lee, a doctoral student in the Kim lab and the first author of the paper, states that improving the early stage of this image generation process was considered to be one of the huge difficulties in making ProteinSGM.
A key idea was the proper image-like representation of protein structure, such that the diffusion model can learn how to generate novel proteins accurately.
Jin Sub (Michael) Lee, Study First Author and Doctoral Student in the Kim Lab, University of Toronto
Lee is from Vancouver but did his undergraduate degree in South Korea and master’s in Switzerland before selecting U of T for his doctorate.
Moreover, it was hard to perform the validation of the proteins produced by ProteinSGM. The system produces several structures, often dissimilar to anything found in nature. Nearly all of them look real as the standard metrics, states Lee, but the scientists required additional proof.
For their new proteins to be tested, Lee and his collaborators first turned to OmegaFold, an enhanced version of DeepMind’s software AlphaFold 2. However, both platforms made use of AI to forecast the structure of proteins depending on amino acid sequences.
With OmegaFold, the research group verified that nearly all their novel sequences fold into the preferred and also novel protein structures. Further, they selected a smaller number to make physically in test tubes, to verify the structures were proteins and not just stray strings of chemical compounds.
Lee stated, “With matches in OmegaFold and experimental testing in the lab, we could be confident these were properly folded proteins. It was amazing to see validation of these fully new protein folds that don’t exist anywhere in nature.”
Additional steps depending on this work include additional development of ProteinSGM for antibodies and other proteins with the majority of the therapeutic potential.
Kim stated. “This will be a very exciting area for research and entrepreneurship.”
Lee states that he would like to view generative biology move toward the collaborative design of protein sequences and structures, such as protein side-chain conformations. So far, the majority of the research has concentrated on the generation of backbones, the main chemical structures that hold proteins collectively.
Lee stated, “Side-chain configurations ultimately determine protein function, and although designing them means an exponential increase in complexity, it may be possible with proper engineering. We hope to find out.”
This study was financially supported by the Canadian Institutes of Health Research.
Journal Reference:
Lee, J. S., et al. (2023) Score-based generative modeling for de novo protein design. Nature Computational Science. doi.org/10.1038/s43588-023-00440-3.