Sepsis is a life-threatening condition that occurs when the body responds adversely to infection. It is essential to detect sepsis early and provide effective treatment to prevent extreme outcomes.1 The high heterogeneity of sepsis complicates its prompt and accurate diagnosis. This article explores the effectiveness of artificial intelligence (AI)-based automated systems for the early detection of sepsis.
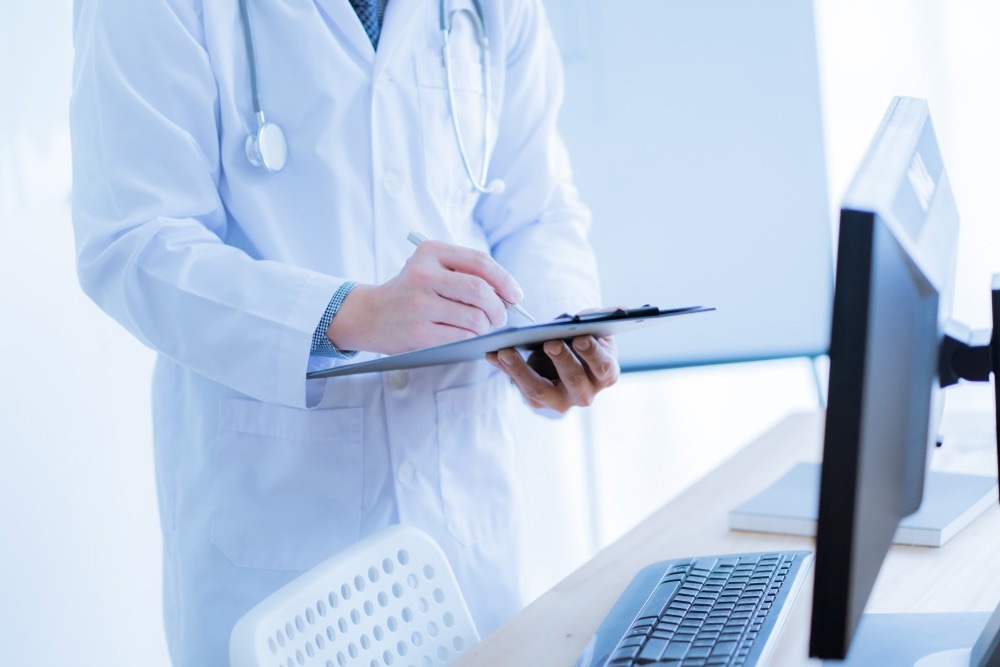
Image Credit: Portrait Image Asia/Shutterstock.com
What Is Sepsis?
Sepsis is associated with the development of systemic inflammatory response syndrome (SIRS) due to infection. It is characterized by abnormal white blood cell counts (leukopenia or leucocytosis), increased heart and respiratory rates, and abnormal body temperatures, such as fever or hypothermia.2
Severe sepsis leads to septic shock, a serious condition marked by prolonged hypotension even after fluid resuscitation. In the case of severe sepsis, patients undergo organ dysfunction.
Sepsis can arise from the improper functioning of the host defense system post-infection. In this context, the Sequential Organ Failure Assessment (SOFA) score is a marker of cardiovascular, respiratory, renal, and nervous system functionality. During infection, the SOFA score increases by two or more points.3
Why Is Early Detection of Sepsis Important?
A recent study revealed that sepsis is one of the leading causes of death worldwide. Given the time-sensitive nature of sepsis management, the early detection of this condition could significantly lower mortality rates.4
According to sepsis management guidelines, early fluid resuscitation is crucial within the first three hours of sepsis. It is also essential to start intravenous antimicrobial treatment as early as possible—within one hour of sepsis.
It has been observed that sepsis mortality rates significantly increase with each hour of delay in administering antimicrobial administration.
Early sepsis identification is challenging due to the variability and ambiguity of early symptoms and operational constraints in healthcare systems, such as delays in communication between nurses and clinicians.
Development of AI-based Tools for Early Sepsis Diagnosis
Current methods for diagnosing and predicting sepsis onset are based on structured data stored in the electronic medical records (EMR) system. However, more than 80 % of clinical data in EMR systems are unstructured, meaning the data is not stored in a specific format. Unstructured clinical data, such as clinical notes and images, provide vital information about the patient.5
Unstructured EMR data is a vital source for developing AI tools to diagnose medical conditions like sepsis. Several studies have shown that clinical note mining improves the accuracy of early sepsis prediction.
In clinical note mining, natural language processing (NLP) has been used to identify and extract relevant medical information. This concept has been implemented to develop text-mining sepsis-predicting algorithms for early sepsis detection with a warning lead time of up to 48 hours.6
Many strategies have been implemented to improve the prediction accuracy of AI-based systems. This includes designing tools that identify common words in the clinical notes that could indicate a risk of sepsis onset. Some researchers have also highlighted that extracting common topics (such as lexicographical) rather than words could improve sepsis prediction accuracy.
A newly developed NLP-enabled AI algorithm has been developed that extracts, analyzes, and summarizes physicians’ clinical notes to predict sepsis risks. This model combines summarized clinical information with structured EMR clinical variables to determine whether a patient has developed sepsis.
This model surpasses previous NLP sepsis predictive models because it operates successfully in conditions where sepsis prevalence is low. The main advantage of this model is that it uses clinical notes over structural variables to predict sepsis. Implementation of this AI-based tool in hospital settings could significantly reduce overall mortality due to sepsis.
Machine Learning Tools for Early Sepsis Identification
Machine learning (ML) methods, a subset of AI, have significantly contributed to the detection and prediction of sepsis.
ML approaches used for sepsis prediction include support vector machines (SVMs), logistic regression, gradient boosting, and random forests. These algorithms utilize vital signs, such as blood pressure and heart rate, laboratory results, and clinical factors (for example, comorbidities and age) to predict the risk of sepsis onset.5
The prediction accuracy and sensitivity of these models have been demonstrated through Area Under the Receiver Operating Characteristic Curve (AUC-ROC) values.
Novel ML-based sepsis screening tools have shown superior prediction capacity compared to traditional methods, utilizing retrospective Electronic Health Record (EHR) data from emergency department visits for prediction analysis.
Deep Learning (DL), a subset of ML, has been used to develop models for the early detection of sepsis. DL models, such as recurrent neural networks (RNNs) and convolutional neural networks (CNNs), have exhibited encouraging results in sepsis prediction.7
A combination of CNN and Long Short-Term Memory (LSTM) network models has demonstrated better efficacy in detecting sepsis using EHR data than traditional ML models.5
Real-World Use and Future Directions in Sepsis Prediction Using AI Models
It is extremely important to validate sepsis prediction models in hospital settings.
Hundreds of hospitals use the Epic Sepsis Model (ESM) to predict sepsis.8 Although its prediction score was below the initial projected score upon validation, without the model, physicians had to evaluate 109 patients to detect one case of sepsis.
The use of the Targeted Real-time Early Warning System (TREWS) in hospitals has significantly improved patient outcomes. Scientists have continually focussed on improving TREWS. Scientists are continuously working on enhancing TREWS, which currently flags 82 % of sepsis cases successfully.9
However, it is recommended that TREWS undergo further validation through a randomized controlled trial (RCT) to ascertain its potential benefits.
Although AI-based tools provide high-precision alerts for sepsis detection, their actual impact on patient outcomes needs further investigation. Future research must focus on the challenges associated with proprietary intellectual property, regulation, and generation of RCT-level evidence for widespread use of AI-based tools in hospital settings.
More from AZoRobotics: Can a Robotic Right Ventricle Transform Cardiac Device Testing?
References and Further Reading
- Duncan, CF., Youngstein, T., Kirrane, MD., Lonsdale DO. (2021). Diagnostic Challenges in Sepsis. Curr Infect Dis Rep. doi.org/10.1007/s11908-021-00765-y
- Hotchkiss, RS., Moldawer, LL., Opal, SM., Reinhart, K., Turnbull, IR., Vincent, JL. (2016). Sepsis and septic shock. Nat Rev Dis Primers. doi.org/10.1038/nrdp.2016.45
- Singer, M., et al. (2016). The Third International Consensus Definitions for Sepsis and Septic Shock (Sepsis-3). JAMA. doi.org/10.1001/jama.2016.0287
- Kim, HI., Park S. (2019). Sepsis: Early Recognition and Optimized Treatment. Tuberc Respir Dis (Seoul). doi.org/10.4046/trd.2018.0041
- Islam, KR., et al. (2023). Machine Learning-Based Early Prediction of Sepsis Using Electronic Health Records: A Systematic Review. J Clin Med. doi.org/10.3390/jcm12175658
- Komorowski, M., Celi, LA., Badawi, O., Gordon, AC. Faisal, AA. (2018). The artificial intelligence clinician learns optimal treatment strategies for sepsis in intensive care. Nat. Med. doi.org/10.1038/s41591-018-0213-5
- Deng, HF., et al. (2021). Evaluating machine learning models for sepsis prediction: A systematic review of methodologies. iScience. doi.org/10.1016/j.isci.2021.103651
- Wong, A., et al. (2021). External Validation of a Widely Implemented Proprietary Sepsis Prediction Model in Hospitalized Patients JAMA Intern Med. doi.org/10.1001/jamainternmed.2021.2626
- Schinkel, M., van der Poll, T., Wiersinga, WJ. (2023). Artificial Intelligence for Early Sepsis Detection: A Word of Caution. Am J Respir Crit Care Med. doi.org/10.1164/rccm.202212-2284VP
Disclaimer: The views expressed here are those of the author expressed in their private capacity and do not necessarily represent the views of AZoM.com Limited T/A AZoNetwork the owner and operator of this website. This disclaimer forms part of the Terms and conditions of use of this website.