By Samudrapom DamReviewed by Susha Cheriyedath, M.Sc.Updated on Oct 9 2024
Swarm robots are an emerging field of robotics technology that involves coordinating multiple robots as a larger system to carry out critical tasks. Robot swarms are finding potential applications in multiple industries.
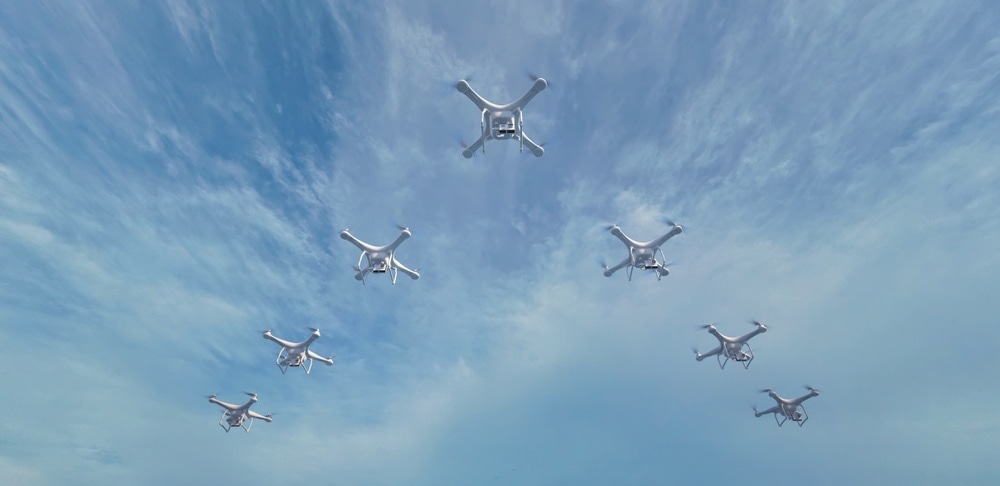
Image Credit: Orange Dragon Studio/Shutterstock.com
Swarm Robots: Definition
Swarm robots are systems comprised of multiple relatively simple physical robots that collaboratively execute tasks. The collective behavior of these robots emerges from local interactions among the robots themselves and their environment, enabling coordinated action.
The concept of swarm robotics is inspired by the natural behaviors observed in social organisms, such as insects, ants, and flocks of birds. This phenomenon, referred to as swarm intelligence, has garnered significant attention in recent years as researchers are focusing on the design of robots and the development of physical properties and behaviors that effectively emulate these natural swarm dynamics.
In a swarm, the individual robots' straightforward behaviors contribute to a broader set of complex group actions, resulting in capabilities that exceed those of any single robot. The collaboration and adaptability of individual robots, along with the dynamics of the group, are crucial for the emergence of sophisticated collective behaviors.
Swarm robots differ from other areas of robotics research, such as general distributed robotic systems, in their emphasis on the contributions of numerous individual robots. These robots utilize local communication through wireless technologies, such as infrared and radio, to facilitate effective coordination. By leveraging distributed and decentralized coordination among groups of simple, homogeneous robots, swarm robots are capable of performing complex tasks that would otherwise be beyond the reach of individual robots.1
Exploring Cutting-Edge Drone Engineering Techniques
Swarm Robots: Applications
Robot swarms have a variety of practical applications across multiple industries, leveraging their collective capabilities to enhance efficiency and effectiveness.
1. Defense and Surveillance
Swarm robotics is increasingly utilized in military applications for reconnaissance, surveillance, and tactical operations. For instance, drone swarms can be deployed for target detection and monitoring, providing enhanced situational awareness in complex environments. These coordinated efforts allow military forces to gather intelligence over large areas, improving decision-making and strategic planning while minimizing risk to personnel.
2. Medicine
Ongoing research indicates that swarm robots have significant potential for precise medical applications, including targeted drug delivery and internal surgical procedures. Small robotic units can collaborate effectively within the human body, working together to deliver medication to specific sites or assist in minimally invasive surgeries. This approach not only improves patient outcomes but also reduces recovery times and the likelihood of complications associated with traditional surgical methods.
3. Space Exploration
Swarm robots are particularly well-suited for planetary surface exploration and the construction of space infrastructure. Their robustness and fault tolerance make them ideal for navigating unpredictable and harsh environments, such as the surfaces of other planets or asteroids. By working in concert, these robots can efficiently perform tasks such as habitat construction, resource extraction, and scientific data collection, thereby enhancing the feasibility and success rates of space missions.
4. Environmental Monitoring
In environmental applications, swarm robots are deployed to collect data related to air quality, water quality, and oceanographic observations. Their ability to operate collectively allows them to cover large areas quickly and efficiently, enabling timely assessments of environmental conditions. This data is crucial for monitoring climate change, assessing pollution levels, and supporting conservation efforts, ultimately contributing to more informed environmental policy and management decisions.
5. Agriculture
In the agricultural sector, swarm robots facilitate a range of tasks such as crop monitoring, planting, and precision farming. By deploying fleets of small robots, farmers can gather data on crop health, optimize irrigation, and even automate the planting process. These scalable solutions not only increase productivity but also promote sustainable practices by minimizing resource use and reducing the environmental impact of farming operations.
6. Construction and Manufacturing
Swarm robots are gaining traction in construction and manufacturing settings, where they perform quality control, automate assembly lines, and execute collaborative tasks. By integrating these robots into smart manufacturing processes, companies can enhance operational efficiency, reduce labor costs, and improve product quality. Their ability to work together also allows for flexible reconfiguration of workflows, adapting to changing production needs in real-time.
7. Search and Rescue Operations
In disaster scenarios, swarm robots are invaluable for exploring and mapping hazardous environments, such as collapsed buildings or unstable terrains. They can locate survivors and coordinate rescue efforts by relaying critical information to emergency responders. This capability significantly improves robustness and flexibility in emergency response operations, allowing for a faster and more effective allocation of resources and personnel.
8. Warehouse Automation and Logistics
In large-scale warehouse environments, swarm robots efficiently manage inventory, move goods, and handle materials. Their enhanced speed and coordination streamline logistics operations, particularly in e-commerce and distribution centers, where rapid order fulfillment is essential. By working collaboratively, these robots can optimize storage space, reduce operational costs, and increase the overall efficiency of supply chain management.2,3
Recent Developments
Recent studies have increasingly focused on addressing memory and communication challenges in the coordination of robot swarms.4-6 Notably, a study published in Applied Sciences explored the evolution of various types of situated and abstract communication within collaborative environments.4
In another paper from Applied Sciences, researchers analyzed how individual robots in a swarm lacking memory capabilities could demonstrate collective memory. An aggregation experiment was conducted, allowing the group to encode and recover information based on specific spatial configurations rather than relying on individual memory storage. The findings revealed that the swarm could independently possess memory, as the robots learned to associate various stimuli with spatial information through simple agents and behavioral rules.5
Furthermore, a distributed path planner for multi-robot systems featuring a priority-based mechanism was proposed in a separate research article.6 This path planner integrated the Bresenham algorithm with particle swarm optimization (PSO) to enhance operational efficiency. The PSO generated optimal trajectories by employing a multi-objective fitness function that considered energy consumption and movement risk, while the Bresenham algorithm ensured comprehensive coverage of the operational area. Communication among the robots was conducted in an ad hoc manner, facilitating the sharing of location and positional information.
The design of automatic controllers is crucial for swarm robotic systems, as these require more sophisticated controllers than single-robot systems to achieve desired collective behaviors. Recent advancements in deep reinforcement learning (DRL) have enabled improvements in automatic controller design. However, a significant challenge of DRL-based methods is their reliance on a central training server, which poses difficulties in real-world environments with limited or unstable robot-server communication.
To address this, a novel federated learning (FL) based DRL training strategy was proposed in IEEE Transactions on Cognitive and Developmental Systems for swarm robotics applications. This innovative approach demonstrated higher generalization ability and robustness in diverse environments and with real robots, overcoming the communication bandwidth limitations faced by other baseline methods. This indicates that the FL-based DRL strategy can significantly enhance swarm robotic systems operating in environments with restricted communication bandwidth, such as subterranean, underwater, or high-radiation settings.7
Traditionally, stigmergy-based collective behaviors in swarm robotics have been designed manually, a process that is often costly, time-consuming, and heavily reliant on the designer's expertise. However, a recent study published in Communications Engineering demonstrated that stigmergy-based behaviors for robot swarms could be generated through an automatic design process. This simulation-based optimization approach produced collective behaviors that allowed robots to lay and sense artificial pheromones. Experimental results indicated that the automatically designed collective behaviors were comparable to, or even superior to, those produced manually. By leveraging pheromone-based stigmergy, this automatic design process enabled the generation of collective behaviors that exhibited spatial organization, effective communication, and memory capabilities.8
Enhancing Drone Autonomy Using AI-Powered Algorithms
Future Trends and Commercial Outlook
The swarm robotics landscape is set to evolve significantly, driven by major trends such as hardware miniaturization, system heterogeneity, phase transitions and adaptability, machine learning integration, and enhanced security measures. Among these, security is anticipated to be the most critical challenge, as issues like data integrity, confidentiality, and entity authentication become increasingly complex due to the interactions among hundreds of robots. The risk of malicious robots infiltrating and disrupting swarm operations further underscores the importance of robust security protocols.
Hardware miniaturization will play a key role in expanding the scope of swarm robotics by enabling more compact and versatile robots that can operate in constrained or delicate environments, such as within the human body or underground. Heterogeneity within robot swarms—where robots with diverse capabilities work in unison—will enhance flexibility and open new possibilities for tackling multifaceted tasks. Additionally, phase transitions and adaptability will allow swarms to adjust their behaviors dynamically, optimizing performance based on environmental conditions.9
The integration of machine learning into swarm robotics will further drive innovation, enhancing the decision-making abilities of individual robots and the swarm as a whole. These intelligent capabilities will enable swarms to autonomously adapt to new environments, learn from previous experiences, and become more efficient in complex scenarios.
Key players within the swarm robotics market, including KION Group AG, Sentien Robotics, Hydromea, and FARobot Inc., are spearheading advancements in the industry. These companies bring an expansive geographic footprint and diverse product offerings, focusing on autonomous swarm robots for warehouse material handling, underwater exploration, and smart factory automation. Their efforts are aimed at creating flexible manufacturing systems that capitalize on the collective intelligence of swarm robots.
In summary, swarm robotics is a rapidly evolving field with immense potential across various industries. Future advancements in hardware miniaturization, machine learning, heterogeneity, and security will further expand the capabilities of swarm robotics, positioning them as a pivotal technology for addressing complex challenges in fields ranging from healthcare to manufacturing. The continued development of these technologies will not only enhance swarm capabilities but also make swarm robotics a key driver of innovation and efficiency in the years to come.
References and Further Reading
- Talamali, M. S., Saha, A., Marshall, J. A., Reina, A. (2021). When less is more: Robot swarms adapt better to changes with constrained communication. Science Robotics, 6(56). DOI: 10.1126/scirobotics.abf1416, https://www.science.org/doi/full/10.1126/scirobotics.abf1416
- Altshuler, Y. Recent Developments in the Theory and Applicability of Swarm Search. Entropy 2023, 25, 710. DOI: 10.3390/e25050710, https://www.mdpi.com/1099-4300/25/5/710
- Schranz Melanie , Umlauft Martina , Sende Micha , Elmenreich Wilfried, 2020. Swarm Robotic Behaviors and Current Applications, Frontiers in Robotics and AI. 7. DOI: 10.3389/frobt.2020.00036, https://www.frontiersin.org/journals/robotics-and-ai/articles/10.3389/frobt.2020.00036
- Gutiérrez, Á. (2021). Evolution of Situated and Abstract Communication in Leader Selection and Borderline Identification Swarm Robotics Problems. Applied Sciences, 11(8), 3516. DOI: 10.3390/app11083516, https://www.mdpi.com/2076-3417/11/8/3516
- Campo, A., Nicolis, S. C., Deneubourg, J. (2021). Collective Memory: Transposing Pavlov’s Experiment to Robot Swarms. Applied Sciences, 11(6), 2632. DOI: 10.3390/app11062632, https://www.mdpi.com/2076-3417/11/6/2632
- Ahmed, N., Pawase, C. J., Chang, K. (2021). Distributed 3-D Path Planning for Multi-UAVs with Full Area Surveillance Based on Particle Swarm Optimization. Applied Sciences, 11(8), 3417. DOI: 10.3390/app11083417, https://www.mdpi.com/2076-3417/11/8/3417
- Na, S. et al. (2023). Federated reinforcement learning for collective navigation of robotic swarms. IEEE Transactions on Cognitive and Developmental Systems, 15(4), 2122-2131. DOI: 10.1109/TCDS.2023.3239815, https://ieeexplore.ieee.org/abstract/document/10025836
- Salman, M., Garzón Ramos, D., & Birattari, M. (2024). Automatic design of stigmergy-based behaviours for robot swarms. Communications Engineering, 3(1), 1-13. DOI: 10.1038/s44172-024-00175-7, https://www.nature.com/articles/s44172-024-00175-7
- Dorigo, M., Theraulaz, G., Trianni, V. (2021). Swarm robotics: Past, present, and future. Proceedings of the IEEE, 109(7), 1152-1165, https://www.researchgate.net/publication/352762371_Swarm_Robotics_Past_Present_and_Future
Disclaimer: The views expressed here are those of the author expressed in their private capacity and do not necessarily represent the views of AZoM.com Limited T/A AZoNetwork the owner and operator of this website. This disclaimer forms part of the Terms and conditions of use of this website.