In recent years, there has been significant interest and investment in the potential of artificial intelligence (AI) and machine learning (ML) to significantly impact the field of pharmaceutical research and development (R&D). These technologies are being utilized in the areas of predictive modeling and simulation in drug discovery and delivering positive results.
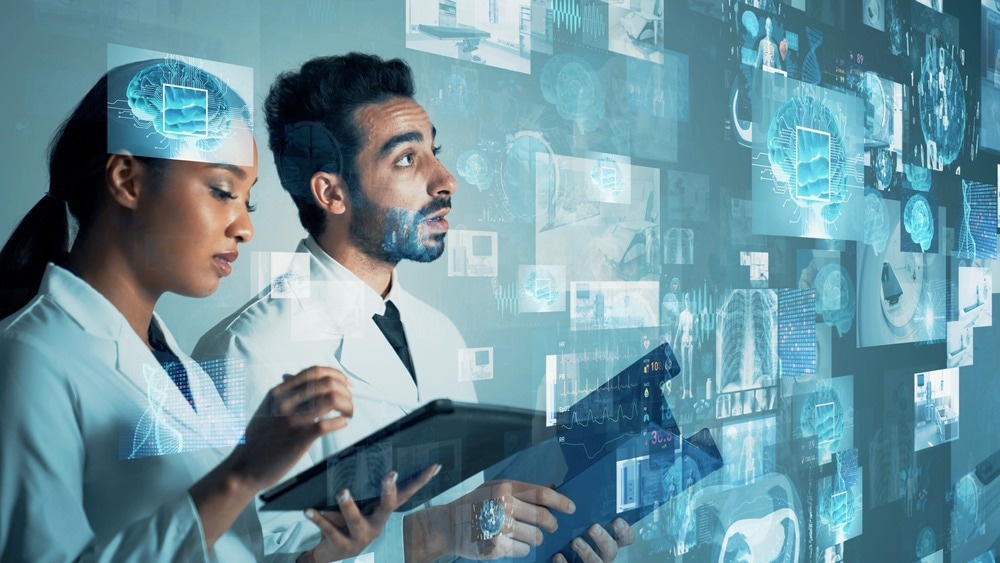
Image Credit: metamorworks/Shutterstock.com
How AI and ML Can Benefit Pre-Clinical-Stage Pharmaceutical R&D
Artificial intelligence (AI) is an algorithmic technique for developing computer processes that emulate human behavior. Machine learning (ML), on the other hand, is a subfield of AI that utilizes algorithms trained with data to achieve intelligent behavior. Deep learning (DL), a type of ML, is modeled on the structure of the human brain. Over the past two decades, ML approaches have been increasingly used in drug discovery.
The COVID-19 pandemic, coupled with broader industrial trends towards automation, digitization, and Industry 4.0, is expected to accelerate the utilization of AI/ML in pharmaceutical R&D. This trend is fueled by significant advances in computational technology and the removal of previous hardware limitations to collecting and processing large volumes of data.
The cost of developing and bringing new drugs to market has become prohibitively expensive, with the average R&D investment per drug estimated at $1.3 billion. The development time for each drug ranges from 6 to 13 years, with an average success rate of 13.8%.
Pharmaceutical companies invest approximately 15% of their revenues in R&D, which is about five-fold more than public expenditure. Despite this investment, the industry has experienced a decline in productivity, with more than an 80% decrease in return on investment during the years 2010-2019.
The complexity of diseases and our lack of understanding of disease biology are major contributors to the high failure rate of clinical trials, which stands at about 90%. Most drugs fail due to low efficacy or high toxicity rather than strategic, commercial, or operative reasons. In some instances, clinical trials have caused serious adverse effects, including death or severe injuries.
Given these challenges facing the pharmaceutical industry, there is a growing interest in leveraging AI/ML techniques due to their ability to automate processes, provide predictive insights, and improve efficiency. These techniques can streamline drug development, reduce costs, accelerate timelines, and increase the likelihood of success.
In pre-clinical R&D, natural language processing (NLP) is being utilized to extract valuable scientific insights from unstructured data sources such as biomedical literature, electronic medical records, and insurance claims. Predictive modeling is also being employed to forecast protein structures and optimize molecular compounds, enabling the identification of drug candidates with higher chances of success.
With the ever-increasing volume of complex data from genomics, imaging, and digital wearable devices, ML methods have emerged as a powerful tool for handling the "Large p, Small n" problem where the number of variables ("p") is high but the number of samples ("n") is low.
Additionally, these methods hold promise for post-marketing research by utilizing real-world data to enrich the understanding of a drug's benefit-risk profile, identify treatment sequence patterns, and pinpoint patient subgroups that may benefit more from one treatment over another, thereby enabling precision medicine.
AlphaFold Predicts Virus’ Protein Structures
AlphaFold, an AI network developed by DeepMind (a Google division), is designed to determine the 3D shape of a protein based on its amino acid sequence. By leveraging a DL approach, AlphaFold predicts the protein's structure using its sequence
This addresses the problem of protein misfolding, which has been linked to several diseases, including type II diabetes and neurodegenerative disorders such as Alzheimer's, Parkinson's, Huntington's, and amyotrophic lateral sclerosis.
Since a protein's biological mechanism is defined by its 3D structure, encoded in its 1D amino acid sequence, accurately predicting 3D protein structures can significantly benefit new drug discovery and advance our understanding of protein-folding diseases.
Knowledge about protein structures is also applied to determine their biological mechanisms and facilitate the discovery of new therapies that can either inhibit or activate specific proteins to treat target diseases.
At the heart of AlphaFold lies a convolutional neural network (a DL model) trained on Protein Data Bank structures to predict the distances between each pair of residues in a protein sequence. These regions are then tiled together to generate distance predictions for the entire protein, which in turn, produces a protein structure that conforms to the distance predictions.
In 2020, AlphaFold made headlines by releasing the structure predictions of five understudied SARS-CoV-2 targets, including SARS-CoV-2 membrane protein, Nsp2, Nsp4, Nsp6, and Papain-like proteinase (C-terminal domain), which is expected to deepen the understanding of these under-studied biological systems.
BenevolentAI
The translation of basic research into safe and effective therapies is challenging due to the complexity of human disease. To address this challenge and facilitate data-driven discoveries, UK’s BenevolentAI has developed a comprehensive knowledge graph. This graph builds a detailed mechanistic representation of the unregulated processes that underlie human disease.
To generate hypotheses for novel biological targets with therapeutic potential for any disease of interest, BenevolentAI utilizes AI and ML. These hypotheses are then experimentally validated in physiologically relevant human patient-derived cell-based systems before entering the company's drug discovery portfolio.
BenevolentAI distinguishes itself in the crowded AI-driven drug development sector with its extensive pipeline of drug candidates, substantial resources, and an increasing partnership with AstraZeneca.
AI- and ML-Driven Drug Development
AI-driven drug discovery shows huge potential for growth in the realm of research and development. As is typical with technological advancements, this presents a unique set of challenges as well as opportunities for progress.
With the advent of modern-day data collection techniques, the sheer magnitude (number of entries, or size) and dimensionality (number of parameters, or complexity) of data will inevitably increase significantly through the use of digital technology.
This, in turn, will create new opportunities for AI and ML techniques to enhance the understanding of biological systems, repurpose drugs for new indications, and inform the design and analysis of clinical trials in drug development.
References and Further Reading
DiNuzzo, M. (2022). How artificial intelligence enables modeling and simulation of biological networks to accelerate drug discovery. Frontiers in Drug Discovery. doi.org/10.3389/fddsv.2022.1019706.
Kolluri, S., et al (2022). Machine Learning and Artificial Intelligence in Pharmaceutical Research and Development: a Review. AAPS J. doi.org/10.1208%2Fs12248-021-00644-3.
Machine learning and AI in biological science, drug discovery and medicine. (2023) [Online] The Royal Society. Available at: https://royalsociety.org/science-events-and-lectures/2023/03/ai-ml-in-biology-tof/.
Wild, D. (2023). The Secret Sauce To BenevolentAI’s Ongoing Success. [Online] In Vivo. Available at: https://invivo.pharmaintelligence.informa.com/IV146802/The-Secret-Sauce-to-BenevolentAIs-Ongoing-Success
Disclaimer: The views expressed here are those of the author expressed in their private capacity and do not necessarily represent the views of AZoM.com Limited T/A AZoNetwork the owner and operator of this website. This disclaimer forms part of the Terms and conditions of use of this website.