Increasingly powerful and accurate artificial intelligence (AI) technologies like machine learning and neural networks are becoming a staple of modern industry. However, as AI grows in reach and function, so does its carbon footprint.
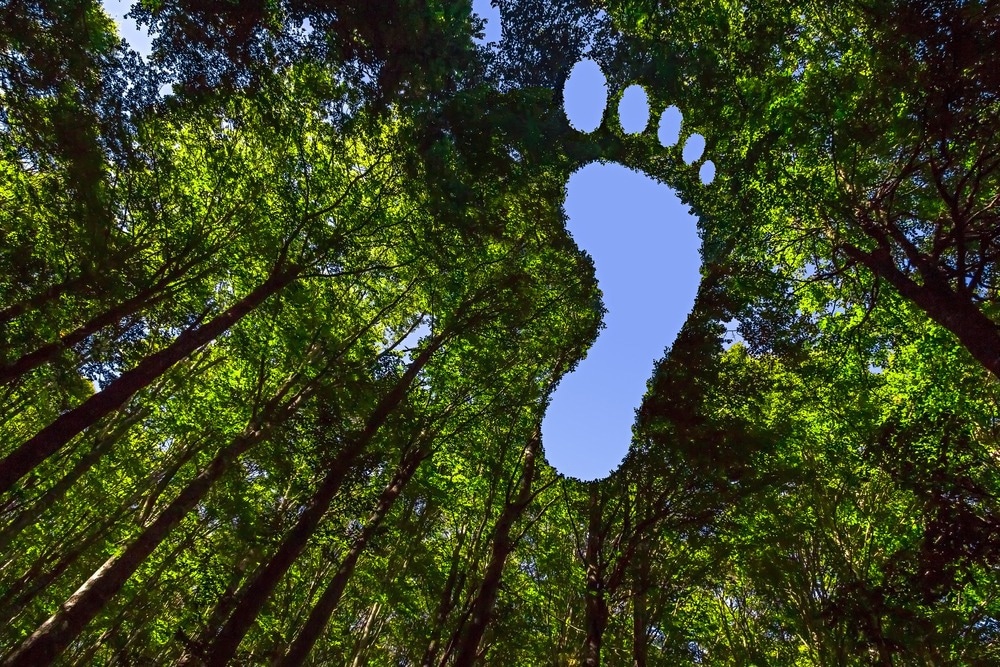
Image Credit: J.M. Image Factory/Shutterstock.com
What is a Digital Carbon Footprint?
A digital carbon footprint is a measure of the carbon emissions involved in the execution of digital activities and the production of digital entities (data). Carbon emissions come primarily from the energy used by computers to generate, transmit, process, and store data. Digitization worldwide generated a reported 4% of the world’s total greenhouse gas emissions in 2020.
Digital data production is going through a rapid growth period as numerous new technologies and business processes are beginning to mature and achieve widespread adoption.
IoT-enabled passive sensing, cloud computing, 5G mobile connectivity, remote satellite internet service, and an ever-increasing number of digital technology users are all contributing to a surge in data. By 2025, data production is predicted to soar to 181 zettabytes (181 trillion gigabytes) annually.
This data storage requirement alone is having a significant impact on the environment and the world’s collectively agreed net zero carbon emissions targets. Data centers’ energy consumption leads to 2.5% of all carbon dioxide emissions caused by humans – aviation only contributes 2.1%.
AI’s Contribution to Digital Carbon Footprints
To make sense – and use – of all of this data, we employ the services of AI Consumers use AI to find another TV show or album from the vast stores of media data they access in the cloud through streaming services.
Financial institutions use AI to make market forecasts based on a sea of microtransactions.
Pharmaceutical manufacturers use AI to develop new medical compounds by continually reevaluating the data in its self-generated models.
Today, AI applications are available and adopted in every industry and activity interacting with big data. As computer hardware, data processing and storage capacity, and algorithmic IP have plummeted in price in the last few years, AI has become widespread in industry, research, policy, and our private lives.
Still, this also has a significant impact on emissions. As well as influencing the growth of data production and requiring large datasets to be effective, AI also consumes energy to process data.
As AI systems are pushed to solve larger problems, they require more data and more computing power to do so. Both of these requirements have a direct impact on AI’s carbon footprint, which is growing as a consequence of this push to increase AI’s reach.
Machine learning systems, for example, need to simulate millions of scenarios and perform statistical analysis on them. These “training” sessions can go on night and day for weeks and even months, and they consume a lot of computing resources. This translates directly into increased power consumption by that unit (or farm of units).
Climate Change: Your carbon footprint explained - BBC News
Climate Change: Your carbon footprint explained - BBC News. Video Credit: BBC News/YouTube.com
Researching AI’s Carbon Footprint
In the last few years, there has been an emerging academic interest in digital carbon footprints and the carbon footprint of AI, specifically. This interest is related to other technology-critical areas of AI research, for example, in AI ethics. This has resulted in a series of efforts to quantify the carbon footprint of AI, as well as proposals to reduce it.
To measure an AI system’s carbon footprint, one first has to determine its power usage. This is not a simple matter. Computers are often used to train several AI models at once, so the researchers behind a tool for measuring AI’s emissions had to first unpick discrete training sessions from one another.
Then, the team from Stanford, McGill University, and Facebook AI Research had to properly allocate each session’s share of overhead functions like cooling.
Once the power consumption of a discrete AI model can be pinned down, one next has to determine the carbon emissions that that energy use generated. This depends on local electric grid infrastructure and the presence or absence of renewable energy sources.
Carbon intensity is also impacted by the time of day, as locations with solar power provide energy for fewer carbon emissions in the middle of the day when the sun is actively powering the grid.
The researchers found the consumption to emissions rate by searching public data sources for energy supply. For example, California ISO provided real-time data for the experimental AI emission tracker developed in this research.
Another study, led by University of Massachusetts researchers, found that neural networks create 284 tons of carbon dioxide emissions. The team looked at four neural networks used to understand written text: GPT-2, BERT, ELMo, and Transformer. They measured each AI model for one day, sampling the model’s energy consumption. They then built up a total emissions figure by multiplying this by the number of days developers said it took to train each AI.
Is AI’s Carbon Footprint Still Growing?
AI is likely to continue to grow in terms of both capacity and reach. More computing power, more storage capacity and cloud access, and faster connections such as 5G are on the horizon. All of these advances would lead to more applications for AI, which would likely lead to a larger carbon footprint for all AI systems in use.
Still, researchers are combating this future. A so-called “green AI” movement is gaining recognition and support. Some AI conferences now ask for carbon emissions information to be included with submitted papers. Attempts to reduce AI’s carbon footprint include developing more energy-efficient systems and moving training sessions to off-grid, renewable energy powered locations.
Improving our capacity to measure AI’s carbon footprint will underpin efforts to reduce it. This is why tools like those mentioned above are important, as they will give organizations the ability to properly account for and reduce their digital carbon footprint. Impactful decisions like finding more efficient AI systems and relocating carbon-intensive processes are easier to make corrections when the underlying evidence is clear.
References and Further Reading
Andrews, E.L. (2020). AI’s Carbon Footprint Problem. [Online] Stanford University. Available at: https://hai.stanford.edu/news/ais-carbon-footprint-problem
Dhar, P. (2020). The carbon impact of artificial intelligence. Nature. doi.org/10.1038/s42256-020-0219-9.
Dodge, J., et al (2022). Measuring the Carbon Intensity of AI in Cloud Instances. FAccT. doi.org/10.1145/3531146.3533234.
Lu, D. (2019). Creating an AI can be five times worse for the planet than a car. [Online] New Scientist. Available at: https://www.newscientist.com/article/2205779-creating-an-ai-can-be-five-times-worse-for-the-planet-than-a-car
Henderson, P., et al (2020). Towards the Systematic Reporting of the Energy and Carbon Footprints of Machine Learning. arXiv. doi.org/10.48550/arXiv.2002.05651.
Hutson, M. (2022). Measuring AI’s Carbon Footprint. [Online] IEEE Spectrum. Available at: https://spectrum.ieee.org/ai-carbon-footprint
Jackson, T.W. and IR Hodgkinson (2022). ‘Dark data’ is killing the planet – we need digital decarbonisation. [Online] The Conversation. Available at: https://theconversation.com/dark-data-is-killing-the-planet-we-need-digital-decarbonisation-190423 (
---. Keeping a lower profile: how firms can reduce their digital carbon footprints. Journal of Business Strategy. doi.org/10.1108/JBS-03-2022-0048.
Jones, N. (2018). How to stop data centres from gobbling up the world’s electricity. Nature. doi.org/10.1038/d41586-018-06610-y.
Kettle, J. (2021). The internet consumes extraordinary amounts of energy. Here’s how we can make it more sustainable. [Online] The Conversation. Available at: https://theconversation.com/the-internet-consumes-extraordinary-amounts-of-energy-heres-how-we-can-make-it-more-sustainable-160639
Strubell, E., A. Ganesh, and A. McCallum (2019). Energy and Policy Considerations for Deep Learning in NLP. arXiv. doi.org/10.48550/arXiv.1906.02243.
Trueman, C. (2019). Why data centres are the new frontier in the fight against climate change. [Online] Computer World. Available at: https://www.computerworld.com/article/3431148/why-data-centres-are-the-new-frontier-in-the-fight-against-climate-change.html
Disclaimer: The views expressed here are those of the author expressed in their private capacity and do not necessarily represent the views of AZoM.com Limited T/A AZoNetwork the owner and operator of this website. This disclaimer forms part of the Terms and conditions of use of this website.