In situ transmission electron microscopy (TEM) is an effective tool for studying the development of a material's microstructure, topography, and behavior at the atomic level instantaneously.
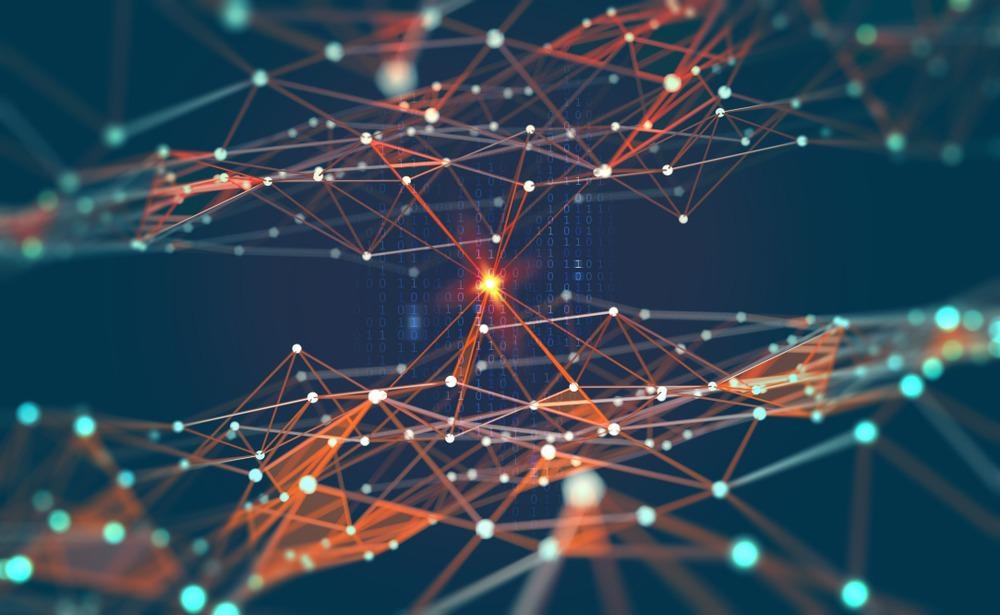
Image Credit: Yurchanka Siarhei/Shutterstock.com
In situ TEM is a technique that requires the use of sophisticated software to analyze big datasets. Because of the rapid growth of artificial intelligence (AI), a variety of machine learning (ML) approaches may now be applied to elucidate the critical data analysis problem for in situ TEM.
Transition Electron Microscopy
Transmission electron microscopy (TEM) is effective equipment for materials analysis that uses ultra-high resolution scanning, scattering, and imaging techniques. When combined with analytical spectrometry, atomic identification, covalent bonds, redox states, and electric configuration can be determined by TEM.
What is In Situ TEM?
The idea behind in situ TEM is to use one or more physical stimuli, such as temperature, pressure, illumination, electronic partiality, magnetization, electromagnetic radiation, or reactive chemical surroundings inside the TEM section to simulate plausible situations where the tested specimen is operated. Doing so enables researchers to monitor the material's spatial, chemical, and operational changes and developments in real-time.
Artificial Intelligence and Machine Learning
Artificial intelligence (AI) is implanted into technologies to enable them to understand and tackle problems in the same way that humans do. Machine learning (ML) is an AI tool that allows device algorithms and techniques to extract knowledge from data and utilize that information to take actions. It has evolved into next-generation deep learning (DL), which uses machine learning techniques to perform cognitive development without the need for human interference.
Utilization of AI in In Situ TEM for Better Analysis
In situ TEM is a data-intensive analysis technique that produces many visual and spectroscopic datasets as a function of frequency, distance, or other experimentation variables. Conventional manual processes for collecting and investigating these huge datasets are laborious and inefficient, and they can also lead to mistakes due to cognitive distortions.
As a result, using AI-enabled techniques to execute automated and accurate data processing for in situ TEM analysis is extremely desirable. ML improved approaches have recently been used for transition electron microscope imaging and spectroscopic studies.
ML has been widely used to analyze microscope images in the field of physics and biological imaging as a subclass of AI technologies. ML attempts to empower devices to accomplish specified tasks autonomously without any need for explicit instructions. It contains two main techniques, controlled ML and uncontrolled ML, based on whether or not human interaction is required.
AI-Based Microscopy Data Analysis and Applications for In Situ TEM
In the last few years, AI-based technologies have been used for TEM data interpretation and have advanced quickly. Some of the applications of AI-based microscopy data analysis for in situ TEM are:
AI-Based Machine Learning for TEM Image Processing
In principle, in situ TEM scanning creates datasets with images specific to a given specimen in a dynamic state as a function of time. The acquisition of image data must be processed and analyzed efficiently and decisively.
ML has substantially enhanced and simplified imaging analysis thanks to AI to tackle everyday tasks in image classification, integration, positioning, segmentation, reconstruction, depiction, and other areas.
AI-enabled image enhancement has been used to aid TEM analysis in various ways, utilizing the existing techniques and resources.
AI-Based Machine Learning for Investigating Atomic Configurations
Identifying atomic places, differentiating atom types, and determining atomic deficiencies are all significant in investigating atomic-resolution TEM/STEM images. Because of its simple contrasts and interpretability, STEM imaging is better suited for this task.
Machine learning approaches for extracting underlying data from atomic STEM images have been pioneered by researchers. From atomic-scale resolution STEM images, Ziatdinov et al. (2017) employed the machine learning approach in conjunction with the Laplacian of Gaussian (LoG) blob sensing to inaugurate the locations of atoms and impurities, such as silicon in graphene.
AI-Based Machine Learning for Identifying Nanoscale Microstructures
To analyze vast quantities of data produced by in situ TEM investigation, the segmentation procedure is a frequent operation in TEM analysis methods, and it needs precision. Deep machine learning can give effective methodologies for the dissection of TEM and STEM images at the scale of individual nanoparticles and nanostructures, in addition to image segmentation of specific atom columns.
Stach et al. (2020) published a case study on conceptual nanoparticle categorization using the convolution neural machine learning method. The research team focused on a gold nanoparticle precursor model system that changed dimensions and structures throughout the Ostwald ripening period, which they examined in situ using TEM.
Current Challenges and Perspectives Regarding AI-Based Analysis in In Situ TEM
For independent and adaptable imaging, in situ TEM needs higher AI engagement, to improve ML's applicability among materials, geometries, and TEM procedures.
Pre-trained ML algorithms should be able to complete new tasks in various material systems for accurate modeling and prediction. Despite early tests, task-specific machine learning algorithms and designs lack resilience and applicability.
Adding domain-specific data and employing physics-informed machine learning are also exciting possibilities. The objective is to provide real-time microscopy data analysis and evaluation, predicting the best operational characteristics to lead the microscope for more data collection. In this difficult endeavor, TEM operators and data science teams must work together.
Continue reading: Training Artificial Intelligence in Industry through Machine Learning Algorithms
References and Further Reading
Zheng, H., Lu, X., & He, K. (2021). In situ transmission electron microscopy and artificial intelligence-enabled data analytics for energy materials. Journal of Energy Chemistry. https://doi.org/10.1016/j.jechem.2021.12.001
Ziatdinov, M., Dyck, O., Maksov, A., Li, X., Sang, X., Xiao, K., ... & Kalinin, S. V. (2017). Deep learning of atomically resolved scanning transmission electron microscopy images: chemical identification and tracking local transformations. ACS Nano, 11(12), 12742-12752. https://doi.org/10.1021/acsnano.7b07504
Horwath, J. P., Zakharov, D. N., Mégret, R., & Stach, E. A. (2020). Understanding important features of deep learning models for segmentation of high-resolution transmission electron microscopy images. npj Computational Materials, 6(1), 1-9. https://doi.org/10.1038/s41524-020-00363-x
Disclaimer: The views expressed here are those of the author expressed in their private capacity and do not necessarily represent the views of AZoM.com Limited T/A AZoNetwork the owner and operator of this website. This disclaimer forms part of the Terms and conditions of use of this website.