We speak with Dr. Richard Hawkins about his involvement in the innovative Assuring Autonomy International Programme, an initiative spanning several sectors that aims to improve the safety of machine learning in autonomous systems. The program has recently developed a standardized methodology to help industries implement safer systems.
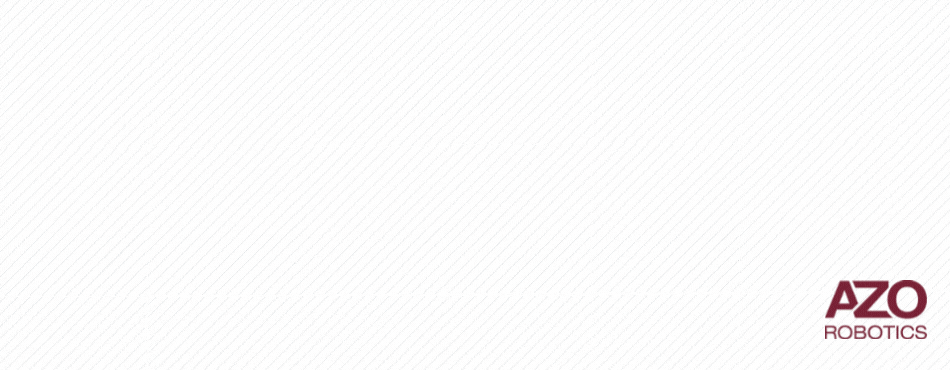
Please could you introduce yourself and your role within the University of York?
My name is Dr. Richard Hawkins and I am a Senior Research Fellow working on the Assuring Autonomy International Programme (AAIP). AAIP is a multi-million-pound research project at the University of York funded by Lloyd’s Register Foundation to find ways to address the challenges of assuring the safety of autonomous systems.
My research, in particular, is focused on how we can demonstrate that autonomous systems are safe enough for operation. We are considering a variety of autonomous systems in many different domains and provide guidance on what needs to be done to give people confidence that they will be safe.
How did you become involved with research into autonomous systems?
I have worked for more than 20 years on the safety of systems that contain a lot of software, like aircraft control systems. Over that time, I have seen software given an increasing level of system control. So in many ways, considering autonomy is a natural progression from that.
Traditionally we would keep a human in the loop even when the software was doing most of the work; someone who was able to take over if needed, like for an auto-pilot on an aircraft. As systems become more autonomous, the human becomes less available to monitor and take over, and in some cases, the human is removed completely. In my opinion, this is the next big challenge for safety assurance.
Could you outline your current research projects and your involvement in AMLAS?
AMLAS is our methodology for machine learning (ML) safety assurance for use in autonomous systems. To develop it, we brought lots of people with different skills together to work out how we could assure the safety of ML software.We are now trying out AMLAS in different domains, including healthcare and automotive.
One particularly interesting test case we have looked at is applying AMLAS to an ML component used on satellites to detect wildfires. The ML can identify wildfires in images taken from space and then send alerts to the ground so that the fire service can determine an appropriate response.
It is crucial that we can provide assurance in the machine learning system as failure to detect the presence of a wildfire or detecting a wildfire in the incorrect location could lead to a response delay. Conversely, raising an alert for a wildfire that does not exist could result in fire responders being misassigned and thus unavailable to respond to legitimate wildfires on time.
The safety of an autonomous system is not just about ML, however. The ML is one component within a much larger, complicated system. As such, we are also researching these wider issues around the safety of the autonomous system as a whole, focusing on how autonomous systems interact with the complex environment they operate in and how we can analyze and control the hazards this can bring about.
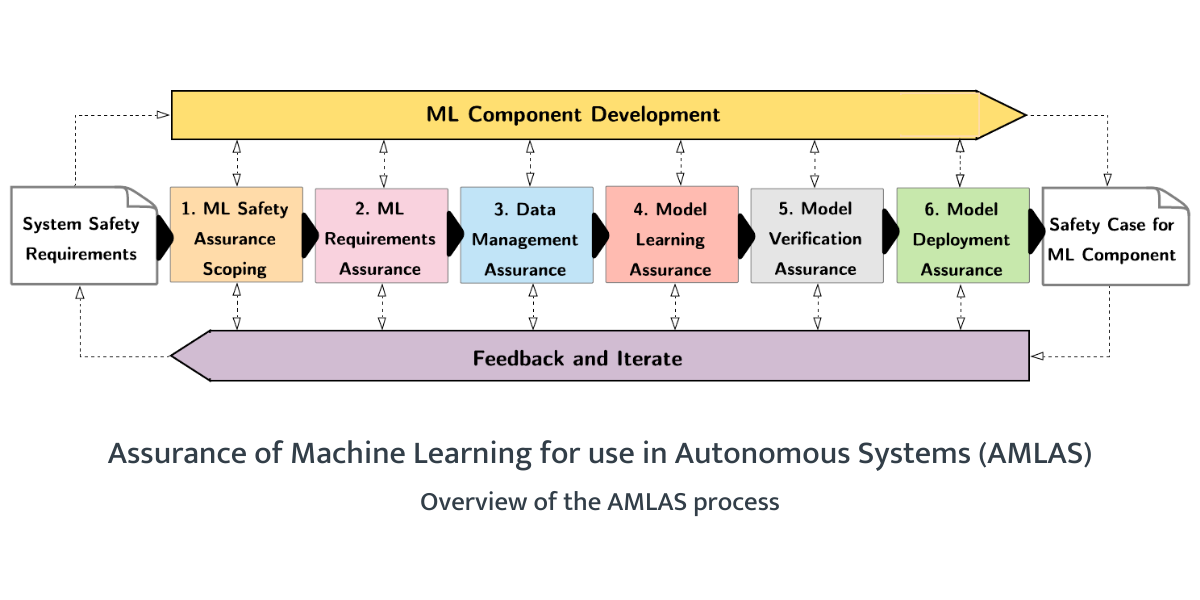
Overview diagram of the AMLAS methodology for assuring the safety of machine learned components. © Assuring Autonomy International Programme/University of York
Although autonomous systems have the power to optimize a myriad of technologies, many people are apprehensive of their use due to risk concerns. How can researchers work with industrial sectors to help reassure individuals of their safety?
Autonomous systems have the potential to bring significant benefits to people's lives, and could also be safer than the systems that they replace. It is also true that people need to have confidence that autonomous systems are sufficiently safe before operation. With the kind of complex technologies we have in autonomous systems, a detailed safety case is needed that explicitly sets out why the system is safe and provides rigorous evidence.
One of the things that AMLAS does is to lay out very clearly what the safety case needs to look like for ML systems so that we can demonstrate that they are safe.
By working closely with industry, researchers can better understand the sort of evidence that can be generated for ML systems that can then feed into that safety case and evaluate which approaches are most effective at demonstrating the safety of the system.
How would you define a “safe” artificial intelligence or machine learning system? Are there any parameters that are critical to the design?
You cannot say whether ML is safe or not without considering the overall system - what it is doing, where it is doing it, and what it needs to interact with in order to do it. Think about ML that classifies objects in images. We cannot say anything about how safe that is unless we know what it is going to be used for - is it going to be used to check medical scans for the presence of tumors? Or will it be used on a self-driving car to identify pedestrians?
We need to be very explicit about what the actual safety requirements are. An ML system is considered safe only once we demonstrate that these safety requirements are met.
It is essential to realize that this is not just about how accurate the ML is. There are vast amounts of research currently looking into ML techniques, and much of this focuses on improving the performance or accuracy of the ML algorithms.
For the kinds of safety-related applications that we are interested in, small improvements in accuracy are not necessarily the most important thing. We are often more concerned about how they perform under many different conditions that the system might encounter during operation, which can be very hard to predict.
For ML, the data is absolutely crucial to this as the system uses the data to learn; if it is not good enough to address the safety requirements, the learned model will not be safe.
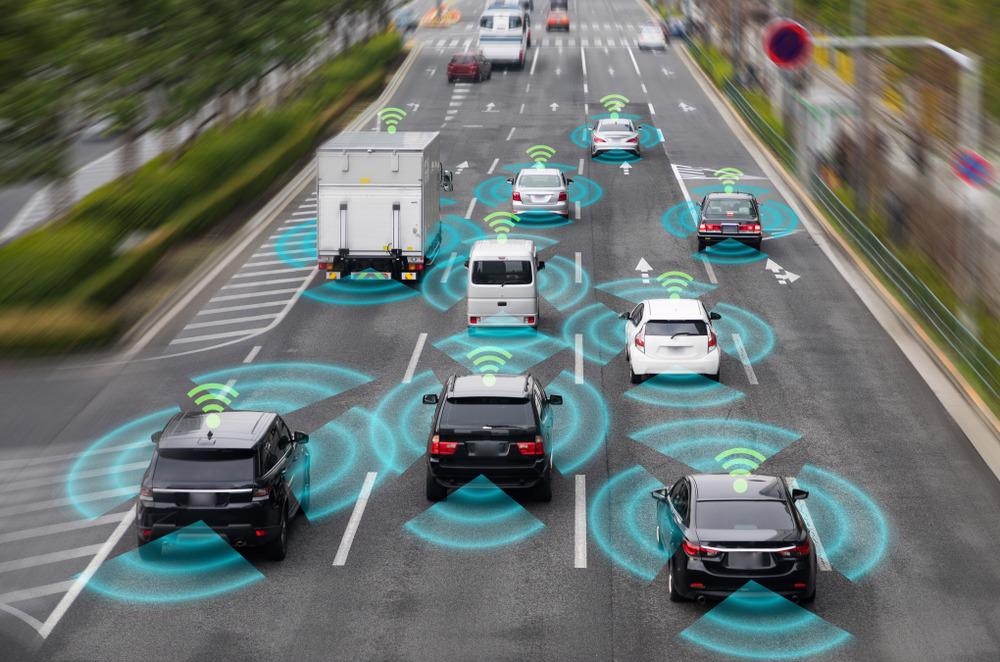
© metamorworks/Shutterstock.com
What aspects of machine learning make it particularly suitable for autonomous systems, especially in sectors like industrial manufacturing?
The real power of machine learning is in its ability to generalize. That means that you can train it to perform a task by using a particular set of training data and it is then able to do the same thing with data that it has not seen before. This makes it particularly powerful for use in the kind of systems that we are interested in that operate in very complex environments where the data that will be encountered during operation can be challenging to predict.
ML can be used for several different tasks in an autonomous system. Generally, it will help the autonomous system understand what is going on in the world around them or where they are in their environment or help the system make decisions on what actions it should perform to safely fulfill its objectives. ML has been shown to be particularly good at tasks such as image classification.
Despite all of the advances that have been made, the artificial intelligence that we have available to us is still relatively narrow. This means that it can do certain specific tasks very well - often much better than a human - but it does not have the general intelligence required to respond to unexpected events.
It is important that we are clear about what the limitations of the technology are.
Where can our readers go to stay up to date with your research activities?
All of our research is freely available for people to make use of.
You can also follow the AAIP on social media to get all of the latest updates.
About Dr. Richard Hawkins
Dr. Richard Hawkins is a Senior Research Fellow at the Assuring Autonomy International Programme within the Department of Computer Science at the University of York. He is undertaking research into the assurance and regulation of autonomous systems, and is also currently involved with the Safer Autonomous System European Training Network and Future Factories in the Cloud research projects.
Previously Dr. Hawkins has worked for BAE Systems as a software safety engineer and was involved in research into modular and incremenatal certification as part of the Industrial Avionics Working Group (IAWG). He has worked as a safety adviser for BNFL at Sellafield, and has undertaken research for companies including Rolls-Royce Submarines and General Dynamics UK.
Disclaimer: The views expressed here are those of the interviewee and do not necessarily represent the views of AZoM.com Limited (T/A) AZoNetwork, the owner and operator of this website. This disclaimer forms part of the Terms and Conditions of use of this website.