Soil health is the foundation of sustainable agriculture, influencing crop yields, water retention, and overall environmental stability. While effective, traditional soil monitoring methods can be time-consuming and lack real-time insights. AI is changing the game by integrating sensor networks and remote sensing technologies, providing farmers and researchers with real-time, data-driven insights to improve soil management and long-term agricultural sustainability.
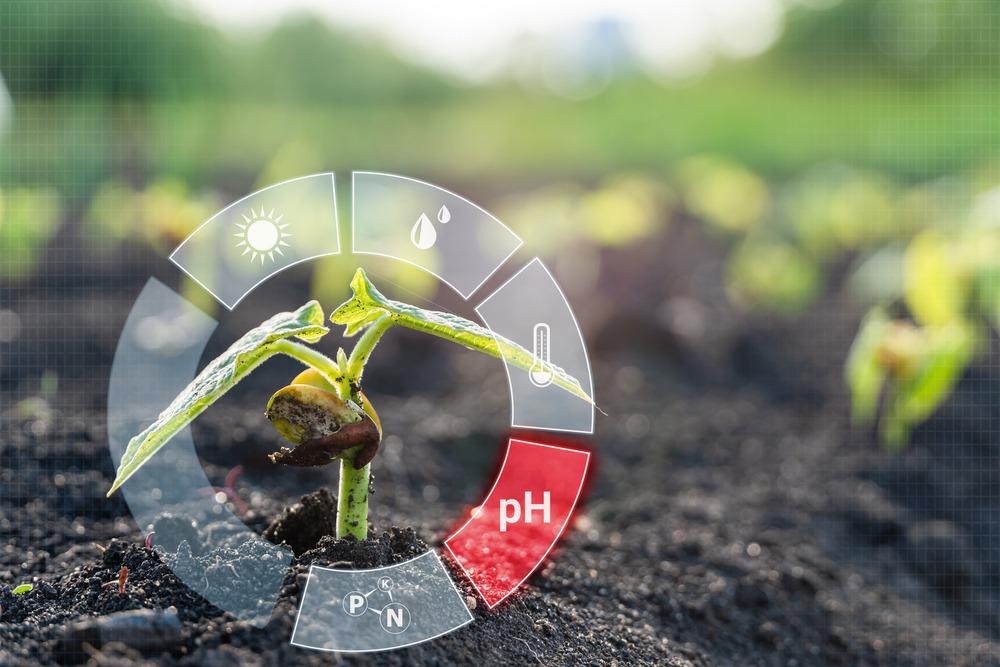
Image Credit: Leonid Sorokin/Shutterstock.com
This article explores why soil quality matters, how AI is enhancing monitoring, the role of remote sensing and predictive management, as well as recent developments in the field.
Want all the details? Grab your free PDF here!
Understanding Soil Quality
Soil quality refers to how well soil can support plant growth, sustain ecosystems, and maintain environmental balance. It’s influenced by a combination of physical, chemical, and biological factors that determine its overall health and functionality.
Key indicators of soil quality include:
- Physical properties – These include texture, bulk density, and aggregate stability, all of which affect water movement, retention, and root growth.
- Chemical factors – Soil pH, nutrient levels (such as nitrogen, phosphorus, and potassium), and cation exchange capacity play a crucial role in plant health and soil fertility.
- Biological components – Organic matter content, microbial activity, and earthworm populations are essential for nutrient cycling and overall soil vitality.
Healthy soil is the foundation of productive agriculture and resilient ecosystems. It provides essential nutrients for plants, improves water absorption and retention, and supports beneficial organisms that enhance soil structure and fertility. High-quality soil also helps prevent erosion, manages pests and diseases naturally, and contributes to carbon sequestration—playing a key role in environmental sustainability.
By monitoring these indicators and implementing good soil management practices, farmers, land managers, and conservationists can maintain and improve soil health, ensuring long-term productivity and environmental stability.1,2
The Role of AI in Soil Quality Monitoring
Traditional soil analysis often requires manually collecting samples and testing them in a lab—a process that can be time-consuming, labor-intensive, and costly. AI is changing this by making soil assessment faster, more accurate, and more comprehensive. By integrating machine learning (ML), sensor technology, and data analytics, AI can process vast amounts of information in real time to evaluate soil health.
For instance, AI-powered sensors continuously monitor key soil metrics such as moisture levels, nutrient content, and pH, providing farmers with instant feedback. ML models analyze historical data, weather patterns, and crop rotation schedules to predict soil fertility trends, helping farmers make proactive, data-driven decisions. AI can also detect early signs of soil contamination and degradation, allowing for timely interventions to maintain soil health.
Enhancing Soil Assessment with AI and Remote Sensing
AI doesn’t just rely on ground sensors—it also works with remote sensing technologies to provide a broader perspective on soil conditions. Satellites, drones, and spectral imaging devices collect large amounts of data on soil composition, moisture distribution, and erosion patterns. AI then processes this data to generate high-resolution soil maps, giving farmers a comprehensive view of their land and guiding more informed decision-making.
Machine learning models trained on spectral reflectance data can classify soil types and identify nutrient deficiencies, reducing the need for manual testing. AI-driven remote sensing also helps detect early signs of soil degradation, allowing farmers to take preventive measures before issues escalate. By integrating AI with remote sensing, soil monitoring becomes more efficient and cost-effective, especially for large agricultural operations.3
Predictive Soil Management with AI
Beyond real-time monitoring, AI also plays a crucial role in predicting future soil conditions. By analyzing historical soil data, climate trends, and cropping patterns, AI can forecast changes in soil health and recommend strategies for maintaining long-term fertility.
Using reinforcement learning and deep learning techniques, AI can optimize fertilization schedules, irrigation plans, and crop rotations to enhance soil sustainability. AI-driven precision agriculture ensures that resources are applied only where needed, reducing waste and minimizing environmental impact. This data-driven approach not only improves soil health and productivity but also supports long-term food security by promoting more sustainable farming practices.2,4
Challenges in AI-Based Soil Monitoring
While AI has the potential to transform soil quality monitoring, several challenges need to be addressed for its widespread adoption.
One of the biggest hurdles is data availability and quality. AI models rely on large datasets for training, yet in many regions, soil data is either insufficient or inconsistent. Additionally, the lack of standardized data collection methods makes it difficult to develop AI algorithms that can deliver accurate and reliable results across different environments.
Cost is another major factor. Advanced AI-driven sensors and monitoring equipment can be expensive, making them more accessible to large commercial farms while leaving smallholder farmers at a disadvantage. Although efforts are being made to develop more affordable AI solutions, financial barriers still limit widespread adoption.
AI models also require continuous updates to account for changes in climate, soil composition, and evolving agricultural practices. This demands ongoing research, technical expertise, and infrastructure support, which may not always be readily available, particularly in developing regions. Additionally, the successful implementation of AI in agriculture requires a certain level of digital literacy—something that can be a challenge in rural areas with limited access to technology and training.
Addressing these challenges will be key to ensuring that AI-driven soil monitoring is both effective and accessible, allowing farmers of all scales to benefit from its potential.
Recent Research and Developments
AI’s role in soil quality monitoring has been the focus of several recent studies, showcasing its potential to enhance agricultural and environmental research.
A study published in the Journal of Soil Science and Plant Nutrition explored AI models for predicting soil respiration (SR), a key process influenced by soil moisture and temperature. Researchers found that AI-based methods, particularly the adaptive neuro-fuzzy inference system (ANFIS), outperformed traditional regression models. Sensitivity analysis revealed that soil moisture had the most significant impact on SR, and ANFIS proved to be the most efficient and accurate model. These findings highlight AI’s ability to improve soil science research and environmental monitoring.5
Another study in SN Applied Sciences examined how AI can be used to monitor soil health, particularly focusing on the soil microbiome. The research emphasized that AI-powered models can analyze vast datasets from soil samples to identify microbial patterns that contribute to soil fertility and plant health. While research in this area is still developing, the findings suggest that AI has significant potential for precision agriculture—helping to assess soil health, optimize fertilization strategies, and predict disease outbreaks in crops.6
What’s Next for Soil Quality Monitoring?
Soil quality monitoring is advancing rapidly, with AI-driven tools improving precision, scalability, and accessibility. Innovations like hyperspectral imaging, when combined with AI, offer deeper insights into soil composition and health. Meanwhile, advanced machine learning models are integrating climate data, satellite imagery, and genomic information to refine predictions and optimize soil management strategies.
As these technologies become more affordable and user-friendly, their adoption is expected to grow, including among small-scale farmers. But how can we ensure these advancements lead to more sustainable agricultural practices, greater food security, and reduced environmental impact?
Looking ahead, the future of soil management will likely depend on a balance between technology, traditional farming knowledge, and sustainable practices. The challenge now is not just about developing smarter tools but making sure they are accessible, practical, and beneficial for farmers worldwide.
Want to Learn More?
Soil health is a complex puzzle, and AI is just one piece of the equation. If you're curious about other cutting-edge methods and scientific insights, check out these articles:
From microbial ecosystems to precision sensing, these resources explore the many ways we can better understand and care for the ground beneath our feet.
References and Further Reading
- Al-Naji, A., Fakhri, A. B., Gharghan, S. K., & Chahl, J. (2021). Soil color analysis based on an RGB camera and an artificial neural network towards smart irrigation: A pilot study. Heliyon, 7(1), e06078 https://doi.org/10.1016/j.heliyon.2021.e06078
- Dharmaraj, V., & Vijayanand, C. (2018). Artificial intelligence (AI) in agriculture. International Journal of Current Microbiology and Applied Sciences, 7(12), 2122-2128. https://doi.org/10.20546/ijcmas.2018.712.241
- Varatharajalu, K., & Ramprabu, J. (2018). Wireless Irrigation System via Phone Call & SMS. Int. J. Eng. Adv. Technol, 8, 397-401. Retrieved from https://www.ijeat.org/wp-content/uploads/papers/v8i2s/B10821282S18.pdf
- Mishra, S. (2022). Emerging Technologies—Principles and Applications in Precision Agriculture. In Data Science in Agriculture and Natural Resource Management (pp. 31-53). Springer, Singapore. https://doi.org/10.1007/978-981-16-5847-1_2
- 4EI. (2021). Technology Innovation is Transforming Soil Health Monitoring. [Online] 4 Earth Intelligence. Available at: https://www.4earthintelligence.com/insights/technology-innovation-transforming-soil-health-monitoring
- World Bank. (2021). A Roadmap for Building the Digital Future of Food and Agriculture. [Online] The World Bank. Available at: https://www.worldbank.org/en/news/feature/2021/03/16/a-roadmap-for-building-the-digital-future-of-food-and-agriculture
- InCeres. (2018). Artificial Intelligence for Soil Fertility Control. [Online] Available at: https://pesquisaparainovacao.fapesp.br/artificial_intelligence_for_soil_fertility_control/747
Disclaimer: The views expressed here are those of the author expressed in their private capacity and do not necessarily represent the views of AZoM.com Limited T/A AZoNetwork the owner and operator of this website. This disclaimer forms part of the Terms and conditions of use of this website.