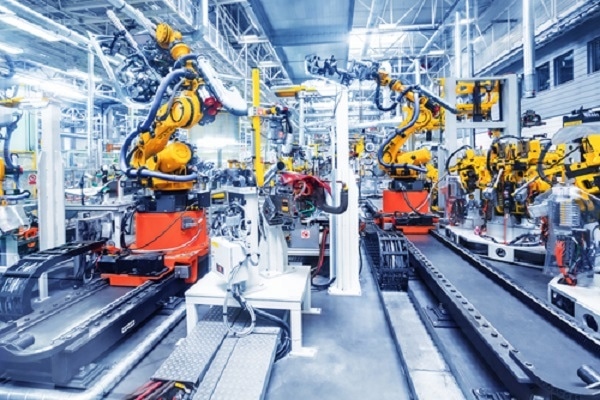
Nataliya Hora / Shutterstock.com
Since the start of the industrial revolution, machines began to perform work that previously required human hands. Throughout the 1950s and 1960s, engineers experimented with robotics as a means of industrial development. The Stanford Arm was created in 1969, a 6-axis robot that could move and assemble parts repeatedly in a continuous pattern. This invention gave robots a practical application in assembly lines and lead to the industrial robotics we have today (Corday, 2014).
Industrial robots are automated, programmable systems used in manufacturing, typically capable of moving on two or more axes (International Organisation for Standardisation (ISO), 2012). Most robots fall into the category of robotic arms, and have at least some degree of autonomy, meaning they are capable of performing tasks without explicit human control. Tasks undertaken by industrial robots include welding, painting, lifting, packaging and inspection.
Industrial robots are most common in automobile manufacture. Within this industry, robots typically undertake the jobs that require the least delicacy such as heavy lifting, painting and welding. Human hands are still relied upon for more intricate work involving small parts or wiring which needs to be guided into place. The robotic arms are also typically large structures which are difficult to move around the factory. However, robots are now being produced with much greater dexterity which will allow them to challenge humans for the more intricate work. Robots manufactured by Universal Robots AS of Denmark are being utilised at the Renault SA plant in Cleon, France, to drive screws into engines, a precise task usually exclusively carried out by a human worker. Furthermore, the robots used at Renault weigh around 30 kg and are easily moved around the workplace. This allows the manufacturer to make shorter runs of custom products without the usual large amount of time and money reconfiguring the factory (Hagerty, 2015).
Collaborative Robots
Typically, industrial robots have been kept fenced away from workers due to the dangerous nature of their operation. Collaborative robots are the new technology to challenge this, which through design allows safe collaboration between humans and robots in the same workstation. The key characteristics required to allow this are as follows:
- Designed to be safe around people, by limiting force to avoid injury if the robot and human interfere or using sensors to prevent touching, or a combination of both.
- Lightweight design to allow the robot to be moved from task to task as required.
- A low skill requirement to use to allow a large range of workers to operate. They are typically operated with a tablet or smartphone (Henry, 2015).
Kawasaki Robotics have recently released the duAro, a first-of-its-kind collaborative robot. It uses low-power motors, soft body, speed and shared work zone monitoring allowing collaborative work with humans. In the unlikely event of a collision, the collision detection function instantaneously stops the robot’s movement. Tasks are taught to the robot by hand guiding its arms, and can complete tasks such as material handling, assembly, machine tending and dispensing using both of its 2kg payload arms in coordinated movements. Installation of duAro is simple, base mounted wheels allow a single user to easily move the robot to any location desired (Robotics.org, 2016).
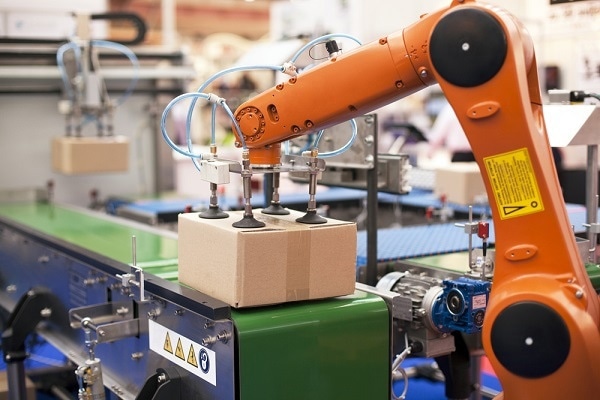
Wellphoto / Shutterstock.com
Artificial Intelligence
Artificial intelligence in robots is a rapidly developing field of research. AI allows tasks to be completed that require dexterity and special awareness by a process of learning, meaning the robot can be introduced to new scenarios and react correctly without pre-programming. UC Berkeley researchers have developed algorithms that “enable robots to learn motor tasks through trial and error using a process that more closely approximates the way humans learn, marking a major milestone in the field of artificial intelligence” (Yang, 2016). This technique was successfully used for a variety of tasks such as assembling a toy plane, screwing a cap on a water bottle and putting clothes hangers on a rack – all without any pre-programmed details on its surroundings.
A new branch of artificial intelligence known as deep learning was used. Deep learning is another name for artificial neural networks (ANNs), which are a family of models inspired by biological neural networks (the central nervous systems of animals, particularly within the brain) and are used to estimate functions that depend on many inputs and are generally unknown. ANNs are designed as systems of interconnected “neurons” which can exchange messages to each other. The connections have numeric weights that can be tuned based on experience, making neural networks adaptive to inputs and thus capable of learning. ‘Deep learning’ is called deep due to the structure of ANNs, with layers of neurons stacked on top of each other. The lowest layer takes the raw data such as images, text or sound, and each neuron stores some information about the data they encounter. Each neuron in the layer sends information up to the next layer of neurons which learn a more abstract version of the date below it (Tyagi, 2016).
This technology has already been successfully implemented in speech and vision recognition in Apple’s Siri and Google Street View. However, these applications can make use of labelled directions where they have examples of how to solve the problem in advance, such as stored data on different existing words. Moving in an unstructured 3D environment does not have these directions and thus is a much greater challenge. The robot currently being used in experimentation by UC Berkley uses an algorithm with a reward function that provides a score based on how well the robot is doing the set task. A camera tracks the position of its arms and legs and analyses its surroundings, and a real time score is fed back to the robot with respect to its movement. Optimal movement with respect to the goal can be learnt on its own through repetition – however, it currently calculates ‘good’ values for around 92,000 parameters and thus the time to optimise is limited by data processing hardware. At present, with no prior data on object location the learning process takes approximately 3 hours. There is a long way before this technology will be commercially viable for production lines, but the revolutionary effect of this technology in the future will be huge in enabling robots to learn complex tasks from scratch.
BRETT the Robot learns to put things together on his own
References
Corday, R., 2014. The evolution of assembly lines: A brief history. [Online]
Available at: http://robohub.org/the-evolution-of-assembly-lines-a-brief-history/
[Accessed 9 September 2016].
Hagerty, J. R., 2015. Meet the New Generation of Robots for Manufacturing. [Online]
Available at: https://www.wsj.com/articles/meet-the-new-generation-of-robots-for-manufacturing-1433300884
[Accessed 9 September 2016].
Henry, J. R., 2015. What are collaborative robots and why should you care?. [Online]
Available at: http://www.packagingdigest.com/robotics/what-are-collaborative-robots-and-why-should-you-care1505
[Accessed 9` September 2016].
International Organisation for Standardisation (ISO), 2012. ISO 8373:2012 - Robotics and robotic devices. [Online]
Available at: https://www.iso.org/obp/ui/#iso:std:iso:8373:ed-2:v1:en
[Accessed 9 September 2016].
automate.org, 2016. Kawasaki Robotics Releases the “duAro”, a First-of-its-Kind Collaborative Robot, for North American Market. [Online]
Available at: https://www.automate.org/news/kawasaki-robotics-releases-the-duaro-a-first-of-its-kind-collaborative-robot-for-north-american-market
[Accessed 9 September 2016].
Tyagi, V., 2016. What is deep learning. [Online]
Available at: https://www.quora.com/What-is-deep-learning
[Accessed 21 September 2016].
Yang, S., 2016. New ‘deep learning’ technique enables robot mastery of skills via trial and error. [Online]
Available at: http://news.berkeley.edu/2015/05/21/deep-learning-robot-masters-skills-via-trial-and-error/
[Accessed 21 September 2016].
Disclaimer: The views expressed here are those of the author expressed in their private capacity and do not necessarily represent the views of AZoM.com Limited T/A AZoNetwork the owner and operator of this website. This disclaimer forms part of the Terms and conditions of use of this website.