Aarne Talman explores the meaning of language understanding, how it is measured, and how the current benchmarks for measurement are flawed.
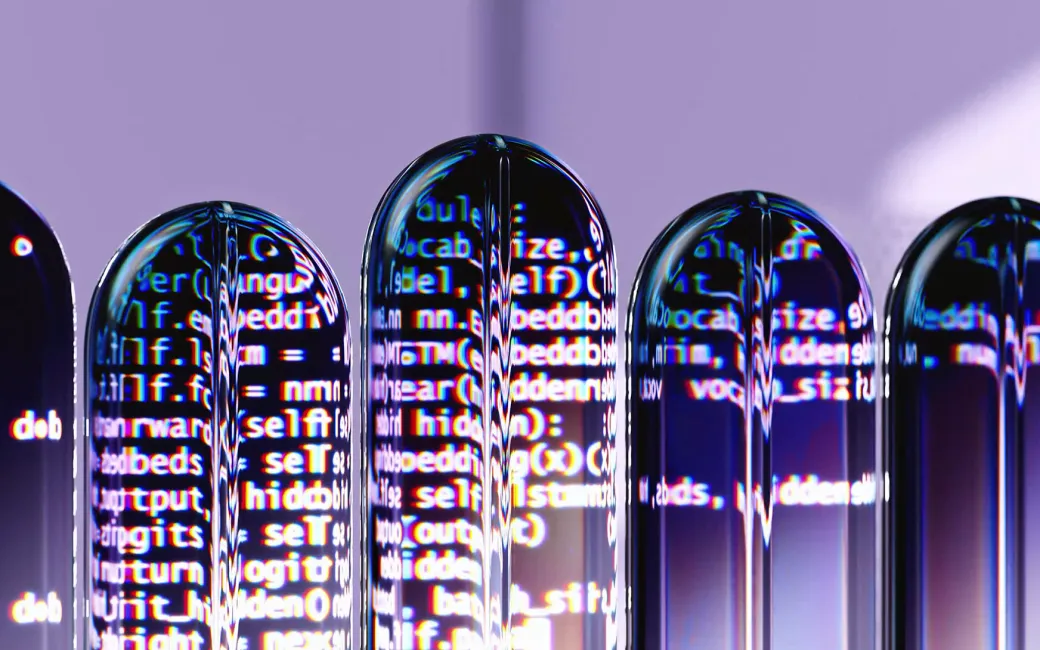
Wes Cockx & Google DeepMind/Better Images of AI/AI large language models. Image Credit: University of Helsinki
Aarne Talman's contributions aid in refining benchmarks, consequently enhancing AI models and facilitating the development of new AI models that more accurately emulate human language comprehension.
According to Talman, the benchmarks used to assess AI models’ proficiency in language understanding do not fully capture their capabilities because the models can accomplish any given task by leveraging patterns found in other datasets.
Talman's research included the development of techniques to improve the language understanding of AI models as well as the evaluation of language understanding benchmarks.
To the best of my knowledge, I was the first to apply the Stochastic Weight Averaging Gaussian (SWAG) method in the context of language understanding. This method enables the development of AI models with language understanding capabilities that better capture the uncertainty involved in human language understanding.
Aarne Talman, University of Helsinki
In research, Talman also elucidates language understanding concepts and initiates a discourse on the conditions that AI models must satisfy to declare them to be capable of comprehending natural language.
The language understanding abilities of AI models have been compared using current benchmarks.
In addition, Talman explores the nature of language understanding in general and the degree to which AI models can comprehend language.
Talman asks, “Can we say that an AI model actually understands the language it reads?”
AI is already and will continue to play a significant role in the society. Understanding language is one of the fundamental components of intelligence.
Talman concludes, “It’s important that we’re able to develop better AI models that more closely match human language understanding. To do so, we must grasp what language understanding means and how it can be measured.”