Artificial intelligence can mimic the development and structure of trees, according to research from the Department of Computer Science and Institute for Digital Forestry at Purdue University and Kiel University in Germany. Sören Pirk is a collaborator in this study.
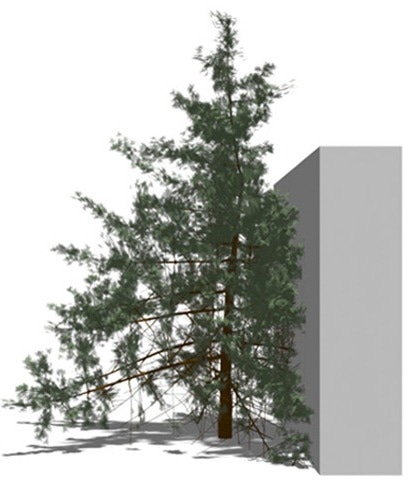
Novel Artificial intelligence-based methods generate tree models that adapt to changing environmental conditions. Image Credit: Bedrich Benes
The DNA molecule encodes both tree structure and environmental response in a small, subcellular bundle. Bedrich Benes, a computer science professor, and his colleagues created unique AI models inspired by DNA that condense the information necessary for encoding tree form into a megabyte-sized neural network.
Following training, the AI models encapsulate the local evolution of trees, which can be utilized to produce massive tree models that provide terabytes of precise geometry as output.
Benes and his colleagues detail their tree-simulation AI models in two publications that were published in the IEEE Transactions on Visualizations and Computer Graphics and ACM Transactions on Graphics of the Association for Computing Machinery.
The AI models learn from large data sets to mimic the intrinsic discovered behavior.
Bedrich Benes, Professor, Purdue University
Digital tree models that are not AI-based are highly intricate, using simulation algorithms that take into account several nonlinear aspects that impact one another. Such models are necessary to make ideas more realistically appealing to their target consumers and audiences in pursuits like architecture and urban planning, as well as in the gaming and entertainment sectors.
Benes anticipated that after over a decade of working with AI models, they would be able to greatly enhance the current techniques for digital tree twins. Still, the models’ stature was unexpected.
He added, “It’s complex behavior, but it has been compressed to rather a small amount of data.”
Computer science graduate students Jae Joong Lee and Bosheng Li from Purdue University were co-authors of the study published in the ACM Transactions on Graphics. Li and Xiaochen Zhou, a graduate student at Purdue University studying computer science, Songlin Fei, the Dean's Chair in Remote Sensing and director of the Institute for Digital Forestry, and Sören Pirk from Kiel University in Germany were co-authors of the study published in the IEEE Transactions on Visualization and Computer Graphics.
The researchers created growth models for maple, oak, pine, walnut, and other tree species with and without leaves using deep learning, a subfield of machine learning within artificial intelligence. Deep learning is the process of creating software that uses connected neural networks to simulate some of the functions of the human brain to teach AI models to carry out certain tasks.
Benes stated, “Although AI has become seemingly pervasive, thus far it has mostly proved highly successful in modeling 3D geometries unrelated to nature.”
These are projects aimed at enhancing algorithms for digital manufacturing and computer-aided design.
The study authors further added, “Getting a 3D geometry vegetation model has been an open problem in computer graphics for decades.”
They pointed out that while certain methods for modeling biological phenomena are becoming better, “simple methods that would quickly provide many 3D models of real trees are not readily available.”
Traditionally, tree-growth models have been produced by experts in biology. They are aware of the interactions between trees and their surroundings. Comprehending these intricate relationships relies on attributes inherited by the tree from its genetic makeup.
Among these are branching angles, which, for example, are substantially greater in pines than in oaks. The same species of tree grown in two separate environments may have radically different forms due to environmental factors dictating other traits.
“Decoupling the tree’s intrinsic properties and its environmental response is extremely complicated. We looked at thousands of trees, and we thought, ‘Hey, let AI learn it.’ And maybe we can then learn the essence of tree form with AI,” Benes added.
Models are usually constructed by scientists from observations and theories about the natural world. They make sense because they are human-made models. The models developed by the researchers extrapolate behavior from thousands of trees’ worth of input data that the AI encoded. Subsequently, the researchers confirm that the models exhibit the same behavior as the input data.
The paucity of training data describing 3D tree geometry found in the actual world is a shortcoming of the AI tree models.
Benes noted, “In our methods, we needed to generate the data. So, our AI models are not simulating nature. They are simulating tree developmental algorithms.”
He wants to rebuild 3D geometry data from real trees within a computer.
He concluded, “You take your cellphone, take a picture of a tree, and you get a 3D geometry inside the computer. It could be rotated. Zoom in. Zoom out. This is next. And it is perfectly aligned with the mission of digital forestry.”
The Department of Computer Science is part of the Purdue Computer initiative.
Journal References
Lee, J. J., et. al. (2024) Latent L-systems: Transformer-based Tree Generator. ACM Transactions on Graphics. doi:10.1145/3627101
Zhou, X., et. al. (2024) DeepTree: Modeling Trees with Situated Latents. IEEE Transactions on Visualizations and Computer Graphics. doi:10.1109/TVCG.2023.3307887