Fingerprints have been the go-to method for connecting offenders to crime detectives, both in real life and in television shows like “Law and Order” and “CSI.” However, it can be exceedingly challenging to connect two crime sites when a criminal leaves prints from various fingers, and the evidence could vanish.
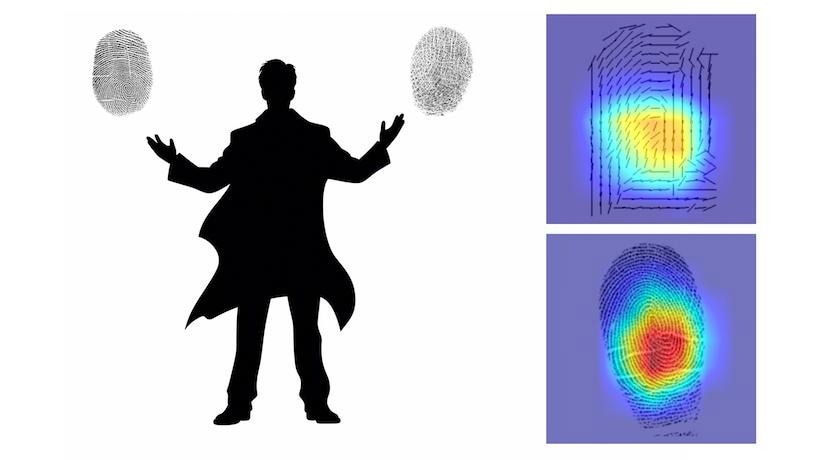
AI discovers a new way to compare fingerprints that seem different, but actually belong to different fingers of the same person. In contrast with traditional forensics, this AI relies mostly on the curvature of the swirls at the center of the fingerprint, as shown by the heatmap. Image Credit: arco-Marcil Montoto, Columbia Engineering, generated with Dall-E
In the field of forensics, it is well acknowledged that fingerprints from various fingers on the same individual—also known as “intra-person fingerprints”—are distinct from one another and cannot be matched.
Research Led by Columbia Engineering Undergraduate
This commonly accepted belief was contested by a group led by senior Gabe Guo, an undergraduate student at Columbia Engineering. Guo, who has no prior experience with forensics, discovered a database of over 60,000 fingerprints kept by the public US government and entered the images in pairs into a deep contrastive network, an artificial intelligence system.
The pairings occasionally belonged to distinct persons, and occasionally they belonged to the same person (but with different fingers).
AI Has Potential to Greatly Improve Forensic Accuracy
The scientists created the AI system by tweaking an innovative framework, and it improved over time in identifying which seemingly distinct fingerprints belonged to the same individual and which ones did not. Just one pair had an accuracy of 77%. The accuracy increased significantly when numerous pairings were shown, which might more than tenfold increase the current level of forensic efficiency.
The study was published in Science Advances and was a partnership between Wenyao Xu’s lab at the University at Buffalo, SUNY, and Hod Lipson’s lab at Columbia Engineering’s Creative Machines lab.
Study Findings Challenge–and Surprise–Forensics Community
The team promptly forwarded the data to a reputable forensics publication after verifying its findings, but a few months later, they were rejected.
The anonymous expert reviewer and editor added, “It is well known that every fingerprint is unique.”
Therefore, even if the fingerprints were from the same individual, it would not be feasible to identify any similarities.
The group persevered. They intensified their efforts, providing their AI system with additional data, and watched as it continued to advance. Knowing that the forensics community would not be buying into their story, the team decided to send their work to a wider audience.
Lipson, the co-director of the Makerspace Facility and the James and Sally Scapa Professor of Innovation in the Department of Mechanical Engineering, filed an appeal when the study was turned down once again.
I don’t normally argue editorial decisions, but this finding was too important to ignore. If this information tips the balance, then I imagine that cold cases could be revived, and even that innocent people could be acquitted.
Hod Lipson, Sally Scapa Professor of Innovation, Department of Mechanical Engineering, Columbia University in the City of New York
Although the accuracy of the system is insufficient to formally determine a case, it can assist in prioritizing leads in instances where there is uncertainty. The work was eventually approved for publication by Science Advances following more back and forth.
Unveiled: A New Kind of Forensic Marker to Precisely Capture Fingerprints
The topic of what alternative information the AI was really using—which had eluded decades of forensic analysis—was one of the main grounds of contention. The team deduced that the AI system was using a novel form of forensic marker following meticulous visualizations of its decision-making process.
The AI was not using ‘minutiae,’ which are the branchings and endpoints in fingerprint ridges – the patterns used in traditional fingerprint comparison. Instead, it was using something else, related to the angles and curvatures of the swirls and loops in the center of the fingerprint.
Gabe Guo, Head Teaching Assistant, Columbia University in the City of New York
Ph.D. candidate Judah Goldfeder and senior engineer Aniv Ray of Columbia Engineering, who assisted with the data analysis, pointed out that their findings are preliminary.
Just imagine how well this will perform once it’s trained on millions, instead of thousands of fingerprints.
Aniv Ray, Senior Engineer, Columbia University in the City of New York
A Need for Broader Datasets
The group is conscious of possible biases in the information. When samples were available, the authors’ evidence showed that the AI functions equally for both genders and ethnicities. However, they point out that if this method is to be applied in real-world scenarios, more meticulous validation must be carried out using datasets with wider coverage.
Transformative Potential of AI in a Well-Established Field
According to Lipson, there will be more unexpected discoveries from AI, like this one.
Lipson noted, “Many people think that AI cannot really make new discoveries–that it just regurgitates knowledge. But this research is an example of how even a fairly simple AI, given a fairly plain dataset that the research community has had lying around for years, can provide insights that have eluded experts for decades.”
He concluded, “Even more exciting is the fact that an undergraduate student, with no background in forensics whatsoever, can use AI to successfully challenge a widely held belief of an entire field. We are about to experience an explosion of AI-led scientific discovery by non-experts, and the expert community, including academia, needs to get ready.”
Journal Reference:
Guo, G., et. al. (2023) Unveiling Intra-Person Fingerprint Similarity via Deep Contrastive Learning. Science Advances. doi:10.1126/sciadv.adi0329