Scientists from Harvard and EPFL have created a ground-breaking new method for tracking neurons in moving and deforming animals.
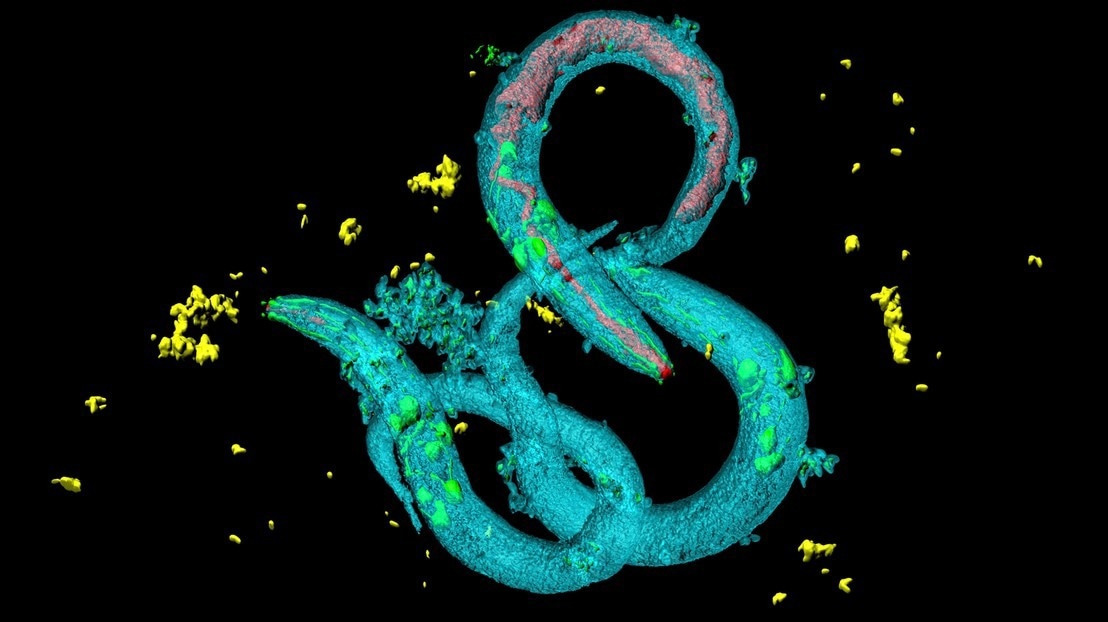
Caenorhabditis elegans. Image Credit: iStock photos (HeitiPaves)
The novel technique combines deep learning with a new methodology known as “targeted augmentation.”
Neurons within freely moving animals can now be imaged, thanks to recent advancements. Nevertheless, these observed neurons need to be computationally recognized and tracked in order to decipher circuit activity. This is extremely difficult when, as in the case of a worm, the brain itself moves and deforms inside the flexible body of the organism. The scientific community lacked the resources to deal with the issue up until now.
A team of researchers from EPFL and Harvard have established a pioneering AI method to track neurons inside moving and deforming animals. The research, now published in Nature Methods, is headed by Sahand Jamal Rahi at EPFL’s School of Basic Sciences.
Convolutional neural networks (CNNs), a subset of artificial intelligence (AI) that have been trained to identify and comprehend patterns in images, are the foundation of the new technique. To make sense of the image and identify objects or patterns, a technique known as “convolution” is used. It takes a look at small portions of the image, such as edges, colors, or forms, at a time.
Identifying and tracking neurons in an animal's brain during a movie poses a challenge due to the varied appearances caused by frequent body deformations. This diversity in the animal's postures necessitates numerous manual image labels to effectively train a Convolutional Neural Network (CNN). However, this task can be daunting due to the sheer volume of annotations required to cover the extensive range of the animal's poses.
The scientists established an enhanced CNN featuring ‘targeted augmentation’ to address this. Using only a small number of manual annotations, the novel method automatically creates trustworthy annotations for reference. As a result, the CNN adeptly grasps the internal deformations within the brain, utilizing this learned information to generate annotations for new postures. This significantly diminishes the necessity for extensive manual annotation and redundant cross-verification.
The novel approach is adaptable; it can recognize neurons in images that show them as discrete spots or as three-dimensional volumes. They conducted tests using the roundworm Caenorhabditis elegans, a widely favored model organism in neuroscience due to its precisely mapped 302 neurons.
The scientists monitored activity in a few of the worm’s interneurons - neurons that transmit messages between neurons - using the improved CNN. Their observations revealed intricate behaviors among these neurons. For instance, these neurons showcased adaptable response patterns when exposed to varying stimuli, such as periodic bursts of odors, showcasing their ability to adapt to different environmental cues.
The group has provided access to their CNN, offering a graphical user interface that is easy to use and incorporates targeted augmentation, simplifying the process into a seamless pipeline that includes manual annotation through final proofreading.
By significantly reducing the manual effort required for neuron segmentation and tracking, the new method increases analysis throughput three times compared to full manual annotation. The breakthrough has the potential to accelerate research in brain imaging and deepen our understanding of neural circuits and behaviors.
Sahand Jamal Rahi, Researcher, Laboratory of the Physics of Biological Systems, Institute of Physics, École Polytechnique Fédérale de Lausanne
Two-dimensional projection of 3D volumetric brain activity recordings in C. elegans.
Video Credit: École Polytechnique Fédérale de Lausanne
Journal Reference
Park, F, C., et al. (2023). Automated neuron tracking inside moving and deforming C. elegans using deep learning and targeted augmentation. Nature Methods. doi/s41592-023-02096-3