Researchers from Karolinska Institute and SciLifeLab have integrated artificial intelligence (AI) techniques commonly used in satellite imaging and community ecology to analyze extensive data from tumor tissue.
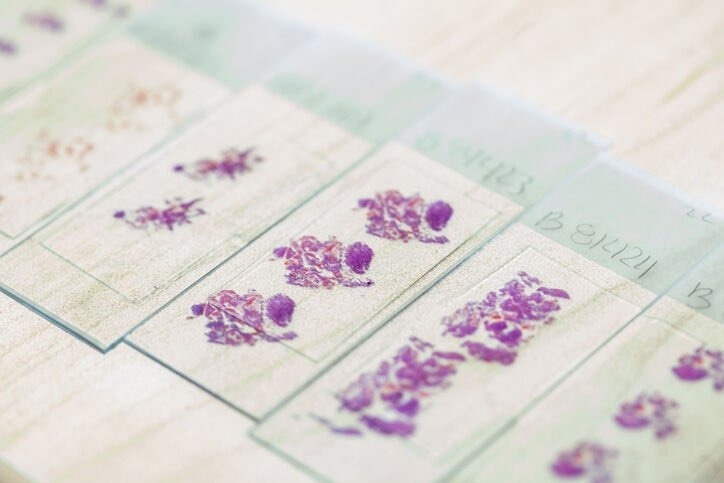
AI methods used in satellite imaging can help researchers analyze tumor images. Image Credit: Getty Images
This innovative method, outlined in the journal Nature Communications, has the potential to enhance the personalization of cancer treatment for patients.
Despite significant progress in tumor imaging that offers detailed insights into the microscopic realm of tumors, the key challenge lies in interpreting the vast amount of generated data. With the simultaneous measurement of hundreds of molecules in tens or hundreds of thousands of cells, researchers face the difficulty of determining which molecules and cells to prioritize for further analysis.
While AI methods have the potential to assist researchers in analyzing this overwhelming volume of data and identifying key areas of focus, traditional AI, like deep neural networks, often operates as a black box.
These systems perform tasks without providing transparent explanations that are easily comprehensible to humans. The details of the process are either hidden or challenging to access. Acknowledging the limitations of such methods, the research team at Karolinska Institute and SciLifeLab turned to inspiration from other fields. They identified well-established analysis techniques in satellite imaging and ecology, dating back to the 2000s and 1950s, respectively.
Similar To Interpreting Satellite Images
Evolving AI methods are being developed to interpret data from satellite images continuously, enabling tasks such as the automatic identification of cities, lakes, forests, and deserts within extensive satellite images.
In ecology, sophisticated techniques are employed to unveil the dynamics of how various species of plants, animals, and microorganisms coexist within a specific geographical area, forming communities.
We realized that the interpretation of tumor images is similar to the interpretation of satellite images and that the relationships between cells in a tissue are similar to the relationships between species in ecology. By combining techniques used in satellite imaging and ecology and adapting them for the analysis of tumor tissue, we have now been able to turn complex data into new insights into how cancer works.
Jean Hausser, Senior Researcher and Author, Department of Cell and Molecular Biology, Karolinska Institute
Tailor Cancer Treatments
The subsequent phase involves implementing the newly developed method in clinical trials. The researchers are engaged in collaborations with a prominent cancer hospital in Lyon, France, to explore reasons behind the varying responses of patients to cancer immunotherapy. Another collaboration with the Mayo Clinic in the US is focused on understanding why certain breast cancer patients do not require chemotherapy.
With our new method, we can reveal important details in tumor tissue that can determine whether a cancer treatment works or not. The long-term goal is to be able to tailor cancer treatments to individual needs and avoid unnecessary side effects.
Jean Hausser, Senior Researcher, Author, Department of Cell and Molecular Biology, Karolinska Institute
The research is funded by the Swedish Cancer Society, the Swedish Research Council, and SciLifeLab. There are no reported conflicts of interest.
Journal Reference
Marrahi, E, A., et al. (2023). NIPMAP: niche-phenotype mapping of multiplex histology data by community ecology. Nature Communications. doi.org/10.1038/s41467-023-42878-z.