In 80% of ovarian cancer patients, the therapy outcome (measured by volumetric decrease of tumor lesions) can be predicted using an artificial intelligence model. With an accuracy of 80%, the AI-based model outperforms existing clinical techniques by a large margin.
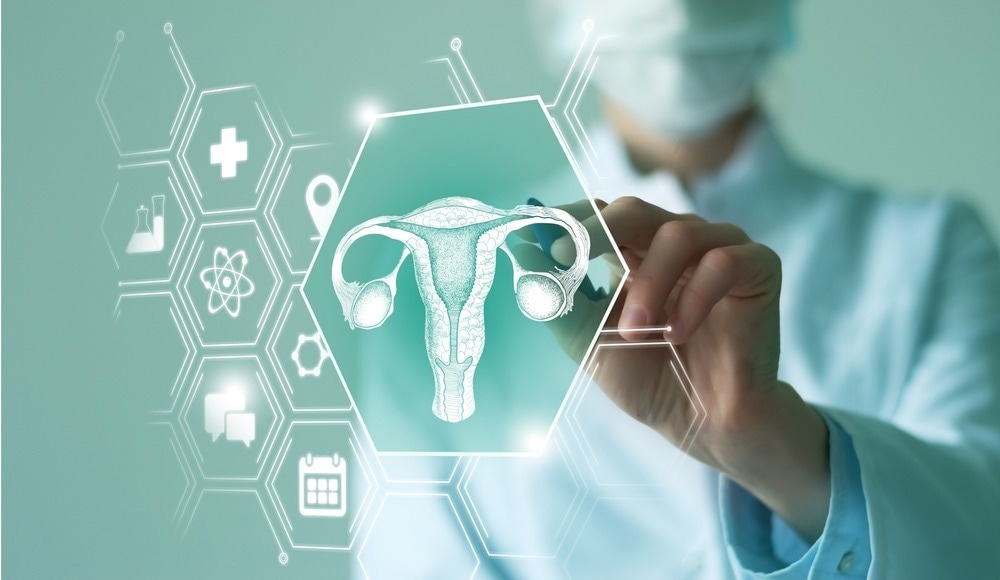
Image Credit: mi_viri/Shutterstock.com
IRON (Integrated Radiogenomics for Ovarian Neoadjuvant treatment) is a tool that evaluates a range of clinical features of patients, including disease images from CT scans, circulating tumor DNA in the blood (liquid biopsy), general characteristics (age, health status, etc.), and tumor markers. It offers an estimate of the likelihood that the therapy will be successful based on this analysis.
This accomplishment is the result of a recent study on 134 individuals with high-grade ovarian cancer that was published in Nature Communications. Professor Evis Sala, Chair of Diagnostic Imaging and Radiotherapy at the Catholic University’s Faculty of Medicine and Surgery and Director of the Advanced Radiology Center at the Policlinico Universitario A. Gemelli IRCCS, oversaw the study. The University of Cambridge team, led by Professor Sala, originally built the AI model.
Background
In Italy, ovarian cancer strikes more than 5,000 women each year, on top of the thirty thousand patients who have already been diagnosed with the disease. As the condition lacks distinct early signs, diagnosis happens when the disease has progressed.
Seventy to eighty percent of ovarian cancers are high-grade serous ovarian carcinoma, which is especially aggressive and often resistant to chemotherapy. For this type of tumor, the prognosis of therapy response is currently just 50% accurate.
Furthermore, due to the great heterogeneity of this type of cancer, which varies significantly from patient to patient, there are few clinically meaningful indicators. As a result, an artificial intelligence-based technique capable of properly predicting chemotherapy responders was developed.
The Study
Professor Sala and Dr. Mireia Crispin Ortuzar from Cambridge added, “We compiled two independent datasets with a total of 134 patients (92 cases in the first dataset, 42 in the second independent test set)."
Clinicians collected clinical data on all patients, including demographic information and treatment details, as well as blood biomarkers such as CA-125 and circulating tumor DNA (ctDNA). Quantitative tumor features were also acquired from CT scan images of all primary and metastatic tumor sites.
Initially, the majority of disease burden was concentrated in the omental and pelvic/ovarian regions (typical for ovarian cancer spread). When compared to pelvic disease, omental deposits responded much better to neoadjuvant therapy. Tumor mutations (for example, TP53 MAF measured on circulating DNA) and the marker CA-125 were linked to the overall disease burden before treatment and therapy response.
Furthermore, improved CT scan image processing identified six patient subgroups with distinct biological and clinical characteristics, indicating therapy responsiveness. All of these tumor characteristics were employed as input data for the artificial intelligence algorithms that comprise the tool. After that, the constructed model was trained, and its efficacy was validated on an independent patient sample.
From a clinical perspective, the proposed framework addresses the unmet need to early identify patients unlikely to respond to neoadjuvant therapy and may be directed to immediate surgical intervention. The tool could be applied to stratify the risk of each individual patient in future clinical research conducted at Policlinico Gemelli in collaboration with Professor Giovanni Scambia's team, Chair of Gynecology and Obstetrics at the Faculty of Medicine and Surgery of the Catholic University and Scientific Director of the Policlinico Universitario Agostino Gemelli IRCCS Foundation.
Evis Sala, Chair of Diagnostic Imaging and Radiotherapy, Faculty of Medicine and Surgery, Università Cattolica
Journal Reference
Crispin-Ortuzar, M., et al. (2023) Integrated radiogenomics models predict response to neoadjuvant chemotherapy in high grade serous ovarian cancer. Nature Communications. doi:10.1038/s41467-023-41820-7