A groundbreaking AI model has the potential to assist policymakers in the efficient identification and prioritization of homes for retrofitting and various decarbonization initiatives.
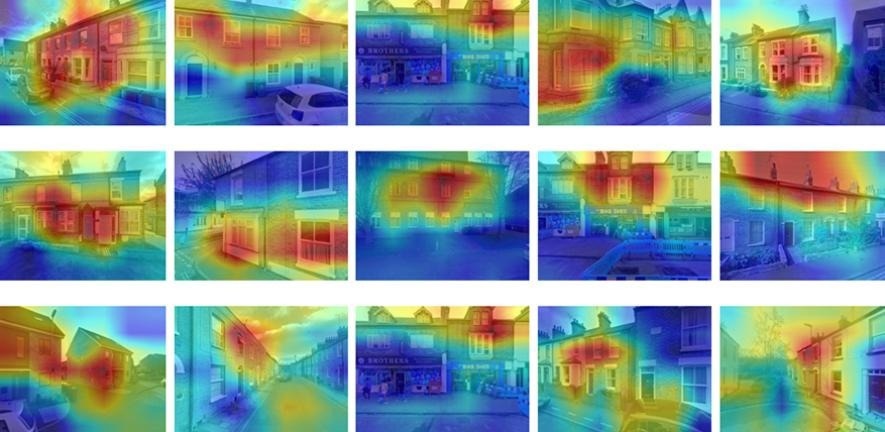
Street view images of houses in Cambridge, UK, identifying building features. Red represents the region contributing most to the “Hard-to-decarbonize” identification. Blue represents low contribution. Image Credit: Ronita Bardhan
“Hard-to-decarbonize” (HtD) residences, accounting for more than a quarter of all direct housing emissions and serving as a significant impediment to achieving net zero emissions, have typically eluded identification and targeted enhancement efforts.
However, a novel “deep learning” model developed by researchers from the Department of Architecture at the University of Cambridge holds the promise of simplifying, expediting, and reducing the cost of recognizing these crucial problem properties and devising strategies to enhance their environmental sustainability.
Various factors can render houses “hard to decarbonize,” including their age, structural characteristics, geographical location, socio-economic obstacles, and the availability of pertinent data. Policymakers have historically concentrated on conventional structures and specific technologies that are challenging to decarbonize.
However, the study published in the Sustainable Cities and Society journal could potentially alter this approach.
Urban researcher and Data Scientist Maoran Sun, along with his Ph.D. Advisor Dr. Ronita Bardhan from Selwyn College, who heads the Sustainable Design Group at Cambridge, has demonstrated that their AI model can achieve a classification accuracy of 90% for challenging-to-decarbonize (CtD) houses.
They anticipate that this precision will further improve as they continue to augment their dataset, a task already in progress.
This is the first time that AI has been trained to identify hard-to-decarbonize buildings using open source data to achieve this.
Dr Ronita Bardhan, PhD Supervisor, University of Cambridge
Bardhan added, “Policymakers need to know how many houses they have to decarbonize, but they often lack the resources to perform detail audits on every house. Our model can direct them to high priority houses, saving them precious time and resources.”
Additionally, the model aids authorities in comprehending the geographic dispersion of challenging-to-decarbonize (CtD) houses, facilitating the precise targeting and efficient deployment of interventions.
The researchers calibrated their AI model using data specific to their hometown, Cambridge, located in the United Kingdom.
They integrated information from Energy Performance Certificates (EPCs), alongside data derived from street view images, aerial view images, land surface temperature, and building stock. In its entirety, their model successfully distinguished 700 CtD houses from 635 non-CtD houses, with all the data sourced from publicly available open-source datasets.
Maoran Sun stated, “We trained our model using the limited EPC data which was available. Now the model can predict for the city’s other houses without the need for any EPC data.”
This data is available freely and our model can even be used in countries where datasets are very patchy. The framework enables users to feed in multi-source datasets for identification of HtD houses.
Dr Ronita Bardhan, PhD Supervisor, University of Cambridge
Sun and Bardhan are currently engaged in the development of an even more sophisticated framework, which will encompass additional layers of data encompassing factors like energy consumption, poverty indicators, and thermal imagery of building exteriors. Their expectation is that this expanded dataset will not only enhance the model's accuracy but also offer a deeper level of information.
The current model already possesses the capability to pinpoint specific building elements, such as roofs and windows, that are experiencing the greatest heat loss, and to discern between older and more modern structures. However, the researchers are confident that they can significantly heighten the level of detail and precision.
They are already in the process of training AI models based on thermal building images from other cities in the UK and are collaborating with a space-oriented organization to leverage higher-resolution thermal images obtained from new satellites.
Notably, Bardhan has played a role in the NSIP – UK Space Agency program, where she worked in conjunction with the Department of Astronomy and Cambridge Zero to employ high-resolution thermal infrared space telescopes for the global monitoring of building energy efficiency.
Sun stated, “Our models will increasingly help residents and authorities to target retrofitting interventions to particular building features like walls, windows, and other elements.”
Bardhan elucidates that hitherto, policy decisions pertaining to decarbonization have relied on information sourced from restricted datasets. However, she holds a positive outlook regarding AI’s potential to transform this situation.
Sun added, “We can now deal with far larger datasets. Moving forward with climate change, we need adaptation strategies based on evidence of the kind provided by our model. Even very simple street view photographs can offer a wealth of information without putting anyone at risk.”
The researchers contend that increasing the visibility and accessibility of data to the public will significantly facilitate the process of garnering consensus for endeavors aimed at reaching a net-zero goal.
Empowering people with their own data makes it much easier for them to negotiate for support.
Dr Ronita Bardhan, PhD Supervisor, University of Cambridge
Bardhan added, “There is a lot of talk about the need for specialized skills to achieve decarbonization but these are simple data sets and we can make this model very user friendly and accessible for the authorities and individual residents.”
Cambridge as a Study Site
Cambridge serves as a unique yet instructive foundation for the initial model. Bardhan points out that Cambridge’s relatively higher affluence translates to a greater inclination and financial capacity for household decarbonization.
Bardhan stated, “Cambridge isn’t ‘hard to reach’ for decarbonization in that sense. But the city’s housing stock is quite old and building bylaws prevent retrofitting and the use of modern materials in some of the more historically important properties. So it faces interesting challenges.”
The researchers plan to share their discoveries with the Cambridge City Council. Bardhan has previously collaborated with the Council on assessing heat loss in council houses. Additionally, they will maintain their collaboration with colleagues at Cambridge Zero and the University’s Decarbonisation Network.
Journal Reference
Sun, M & Bardhan, R (2023) Identifying Hard-to-Decarbonize houses from multi-source data in Cambridge, UK. Sustainable Cities and Society. doi.org/10.1016/j.scs.2023.105015