A fresh method for looking more closely at the intricate behavior of materials has been presented by researchers at the SLAC National Accelerator Laboratory of the Department of Energy. The researchers used machine learning to analyze coherent excitations, which are system-wide swings of atomic spins.
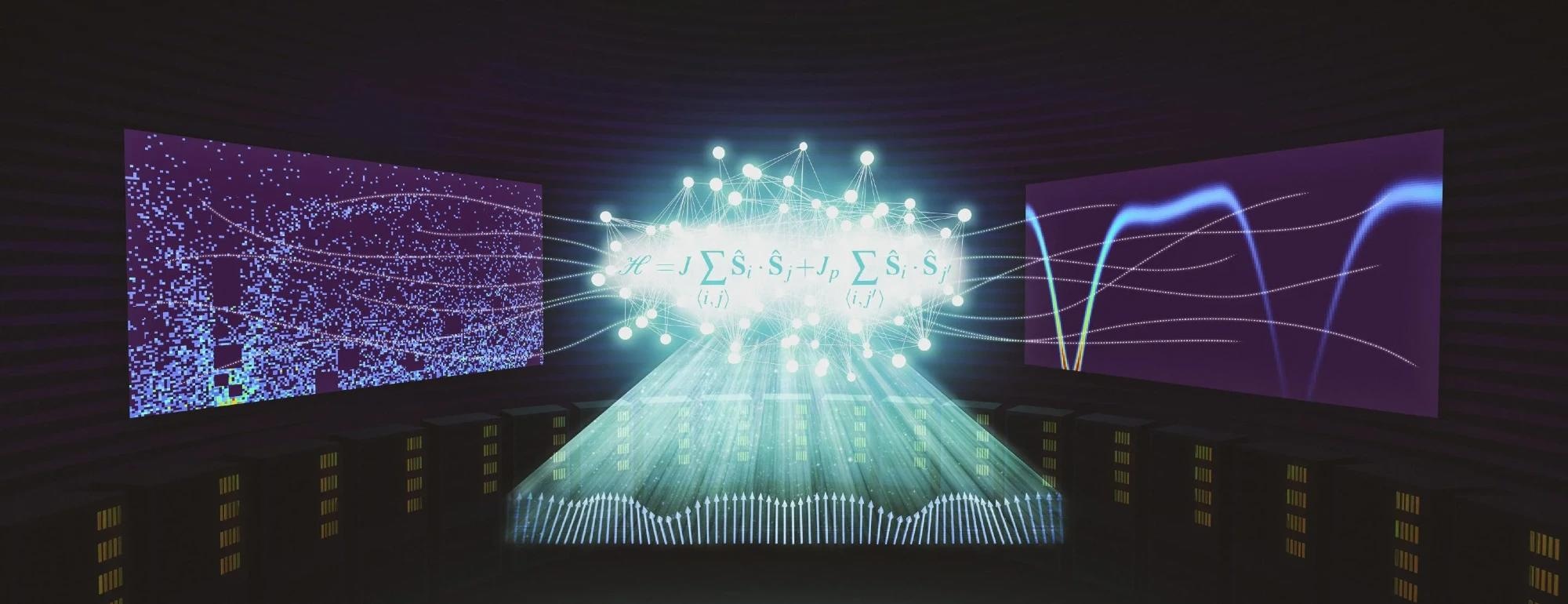
The research reveals the potential for machine learning in understanding the complex behavior of quantum materials. Image Credit: Greg Stewart/SLAC National Accelerator Laboratory
This ground-breaking study, which was just published in Nature Communications, is a component of a DOE-funded project headed by Howard University and involving researchers from SLAC and Northeastern University to use machine learning to speed up research in materials. It could improve experiments by giving researchers real-time guidance while they collect data.
The researchers employed “neural implicit representations,” a machine learning advancement used in computer vision in addition to other scientific domains, including medical imaging, particle physics, and cryo-electron microscopy, to construct this new data-driven tool. This application automates a process that, up until now, required a lot of manual labor by quickly and precisely deriving unknown parameters from experimental data.
Peculiar Behaviors
Collective excitations aid in the understanding of complex systems with numerous moving elements, such as magnetic materials. Some materials exhibit strange characteristics when observed at the smallest sizes, such as minute variations in the patterns of atomic spins. Numerous emerging technologies, including sophisticated spintronics components that could alter how data is transferred and stored, depend on these characteristics.
Scientists utilize methods like inelastic neutron or X-ray scattering to analyze collective excitations. Given, for instance, the scarce supply of neutron sources, these procedures are not only complex but also resource-intensive.
These issues can be addressed by machine learning, but it still has its limitations. The accuracy of X-ray and neutron scattering data interpretation has been improved in previous investigations using machine learning approaches. Traditional image-based data formats were used in these initiatives. The group’s new strategy, which relies on neural implicit representations, takes a different tack.
The inputs for neural implicit representations are coordinates, which are like points on a map. These networks are capable of predicting each individual pixel’s color in image processing depending on its location. By linking the pixel coordinate to its color, the approach develops a formula for how to interpret the image rather than directly storing it.
Because of this, it can accurately forecast events, even between pixels. These models show promise for interpreting quantum materials data since they are good at capturing fine details in images and sceneries.
Our motivation was to understand the underlying physics of the sample we were studying. While neutron scattering can provide invaluable insights, it requires sifting through massive data sets, of which only a fraction is pertinent. By simulating thousands of potential results, we constructed a machine learning model trained to discern nuanced differences in data curves that are virtually indistinguishable to the human eye.
Alexander Petschm, Postdoctoral Research Associate, Linac Coherent Light Source, SLAC National Accelerator Laboratory
Pieces Falling into Place
The goal was to test if measurements made at LCLS could be used to train a machine learning algorithm to understand the microscopic characteristics of the material. In order to learn from all the varied spectra, they conducted hundreds of simulations of what they would measure using a variety of parameters, feeding the results into an algorithm. This allowed them to anticipate the results from theory before they even observed actual spectra.
It turned out that the measurements they actually desired to take were quite similar to inelastic neutron scattering while they were waiting to do this experiment at the LCLS. Petsch discovered that the team’s simulations, which were overseen by Zhurun (Judy) Ji, a Stanford University Science Fellow, completely matched the neutron scattering data from his thesis. The team’s machine learning model proved successful in overcoming obstacles like noise and missing data points when it was applied to this real-world data.
Traditionally, simulations, post-experiment analysis, and intuition have been used by researchers to determine their next moves. The team gave a demonstration of how their method could continually and instantly examine data. This demonstrated the possibility for scientists to decide when they have accumulated enough data to conclude an experiment, greatly simplifying the procedure.
The potential of this method for continuous real-time analysis, which offers insights into when enough data is acquired to end an experiment, is one of the most interesting breakthroughs.
Our machine learning model, trained before the experiment even begins, can rapidly guide the experimental process. It could change the way experiments are conducted at facilities like LCLS.
Josh Turner, Scientist, SLAC National Accelerator Laboratory
Opening Up New Avenues
The design of the model is not restricted to neutron scattering. This system, known as the “coordinate network,” can be used for a variety of scattering measurements that require information as a function of energy and momentum.
Machine learning and artificial intelligence are influencing many different areas of science. Applying new cutting-edge machine learning methods to physics research can enable us to make faster advancements and streamline experiments. It is exciting to consider what we can tackle next based on these foundations. It opens up many new potential avenues of research.
Sathya Chitturi, Study Co-Author and PhD Student, Stanford University
LCLS is a user facility for the DOE Office of Science. The Office of Science (BES) funded this study.
Journal Reference
Chitturi, S. R., et al. (2023) Capturing dynamical correlations using implicit neural representations. Nature Communications. doi:10.1038/s41467-023-41378-4