Individualized healthcare could eventually be made possible by artificial intelligence (AI), which recognizes patterns in complicated biological data. An approach based on AI that can be used to address diverse biological and medical problems has been created by LiU researchers. For example, their models can precisely calculate a person’s chronological age and whether or not they have ever smoked.
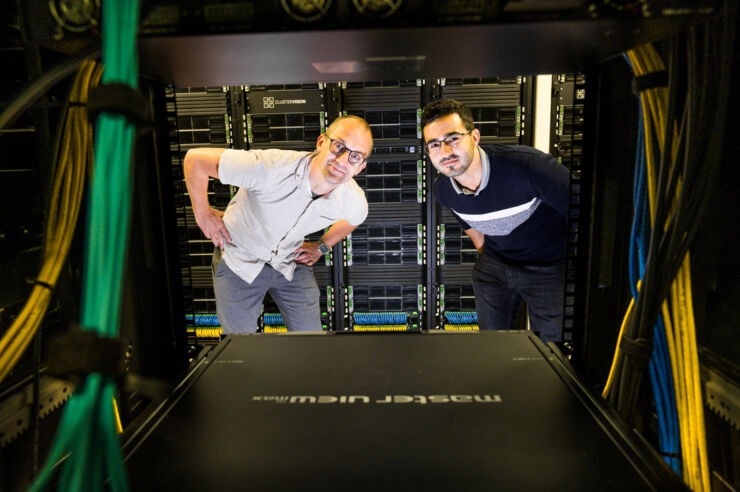
Mika Gustafsson and David Martínez hope that AI-based models could eventually be used in precision medicine to develop treatments and preventive strategies tailored to the individual. Image Credit: Thor Balkhed
There are several factors that might influence which of the genes are utilized at any given moment. Some examples include smoking, poor eating habits, and environmental contamination. This regulation of gene activity, known as epigenetics, can be compared to a power switch that determines which genes are turned on or off without changing the actual genes.
Linköping University (LiU) researchers used data containing epigenetic information from over 75,000 human samples to build a significant number of AI neural network models. They believe that such AI-based models will one day be employed in precision medicine to produce treatments and preventative methods that are specific to the individual. Their models are autoencoders, which self-organize information and identify interrelation patterns in enormous amounts of data.
Smoking Leaves Traces in the DNA
To put their model to the test, the LiU researchers compared it to current models. Existing theories of the effects of smoking on the body are based on the idea that certain epigenetic modifications reflect the effect of smoking on lung function.
These residues stay in a person’s DNA long after they stop smoking, and this type of model could identify if a person is a current, former, or never smoker. Other models, based on epigenetic markers, can estimate an individual’s chronological age or categorize persons depending on whether they have a disease or are healthy.
The LiU researchers trained their autoencoder and then utilized the results to answer three separate questions: age determination, smoker status, and identifying the disease systemic lupus erythematosus, or SLE. Existing models rely on specific epigenetic markers known to be connected with the disease being classified. However, the LiU researchers’ autoencoders performed better or equally well.
Our models not only enable us to classify individuals based on their epigenetic data. We found that our models can identify previously known epigenetic markers used in other models, but also new markers associated with the condition we are examining. One example of this is that our model for smoking identifies markers associated with respiratory diseases, such as lung cancer, and DNA damage.
David Martínez, Ph.D. Student, Linköping University
The goal of autoencoder models is to condense exceedingly complicated biological data into a representation of the most important data characteristics and patterns.
We did not steer the model and had no hypotheses based on existing biological knowledge, but let the data speak for itself. When subsequently looking at what was happening in the autoencoder, we saw that data self-organized in a way similar to how it works in the body.
Mika Gustafsson, Professor, Translational Bioinformatics, Linköping University
The researchers can then use the autoencoder’s most essential attributes to develop models that can categorize for a large number of environment-related, individual-specific aspects where there is insufficient training data to train more complicated AI models.
Interpretable AI Models
Certain forms of AI are frequently compared to a black box that offers answers but cannot be seen by humans. Mika Gustafsson and his colleagues, on the other hand, attempt to develop interpretable AI models that allow researchers to peer under the lid of the “black box” to comprehend what is going on within.
Gustafsson further added, “We want to be able to understand what the model shows us about the biology behind disease and other conditions. Then we’ll see not only whether someone is ill or not, but, by interpreting data, we’ll also have a chance to learn why.”
The Swedish Research Council, the Wallenberg AI, Autonomous Systems and Software Program (WASP), and the SciLifeLab & Wallenberg National Program for Data-Driven Life Science (DDLS) among others, contributed to this study.
Journal Reference
Martínez-Enguita, D., et al. (2023) NCAE: data-driven representations using a deep network-coherent DNA methylation autoencoder identify robust disease and risk factor signatures. Briefings in Bioinformatics. doi:10.1093/bib/bbad293