According to a study published in Radiology, researchers have succeeded in combining imaging information with clinical patient data to improve diagnostic performance on chest X-Rays.
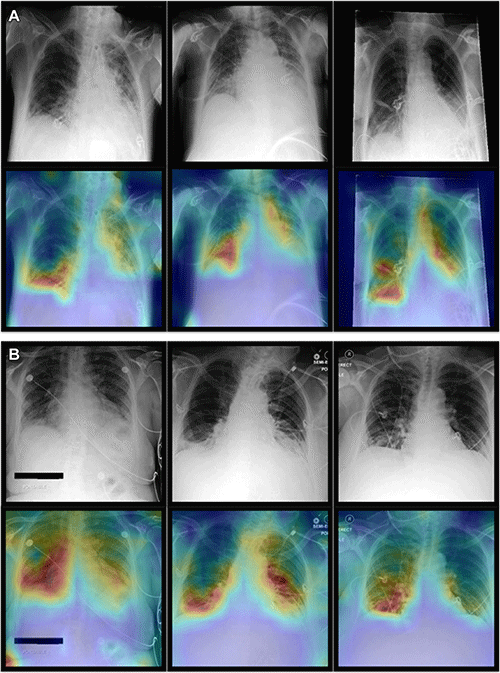
Representative radiographs (top), acquired in anteroposterior projection in the supine position, and corresponding attention maps (bottom). (A) Images show main diagnostic findings of the internal data set in a 49-year-old male patient with congestion, pneumonic infiltrates, and effusion (left); a 64-year-old male patient with congestion, pneumonic infiltrates, and effusion (middle); and a 69-year-old female patient with effusion (right). (B) Images show main diagnostic findings of the Medical Information Mart for Intensive Care data set in a 79-year-old male patient with cardiomegaly and pneumonic infiltrates in the right lower lung (left); a 58-year-old female patient with bilateral atelectasis and effusion in the lower lungs (middle); and a 48-year-old female patient with pneumonic infiltrates in the lower right lung (right). Note that the attention maps consistently focus on the most relevant image regions (e.g., pneumonic opacities are indicated by opaque image regions of the lung). Image Credit: Radiological Society of North America
While physicians use both imaging and non-imaging data to diagnose diseases, current AI-based techniques are designed to solve tasks using just one form of data at a time.
Transformer-based neural networks, an emerging type of AI model, can combine imaging and non-imaging data to provide a more accurate diagnosis. These transformer models were originally designed for computer-assisted human language processing. They have now been used to power huge language models such as ChatGPT and Google’s AI chat service, Bard.
Unlike convolutional neural networks, which are tuned to process imaging data, transformer models form a more general type of neural network. They rely on a so-called attention mechanism, which allows the neural network to learn about relationships in its input.
Firas Khader, Study Lead Author and PhD student in the Department of Diagnostic and Interventional Radiology at University Hospital Aachen
This capacity is particularly useful in medicine, as various factors such as patient data and imaging findings are frequently included in the diagnosis.
Model Trained on Imaging and Non-Imaging Data from 82K Patients
Khader and colleagues created a transformer model specifically for medical applications. They trained it using imaging and non-imaging patient data from two databases, including information from over 82,000 patients in total.
The model was trained to identify up to 25 disorders utilizing non-imaging data, imaging data, or a mix of the two, known as multimodal data.
The multimodal model outperformed the other models in terms of diagnostic performance for all conditions.
In an era of increasing workloads, the approach has the potential to assist clinicians.
Khader added, “With patient data volumes increasing steadily over the years and time that the doctors can spend per patient being limited, it might become increasingly challenging for clinicians to interpret all available information effectively. Multimodal models hold the promise to assist clinicians in their diagnosis by facilitating the aggregation of the available data into an accurate diagnosis.”
According to Khader, the proposed design might serve as a roadmap for effectively integrating enormous data quantities.
AI Combines Chest X-rays with Patient Data to Improve Diagnosis
Vidoe Credit: Radiological Society of North America
Journal Reference:
Khader, F., et al. (2023) Multimodal Deep Learning for Integrating Chest Radiographs and Clinical Parameters: A Case for Transformers. Radiology. doi:10.1148/radiol.230806.