Photos are two-dimensional (2D), but autonomous vehicles and other technologies have to navigate the three-dimensional (3D) world.
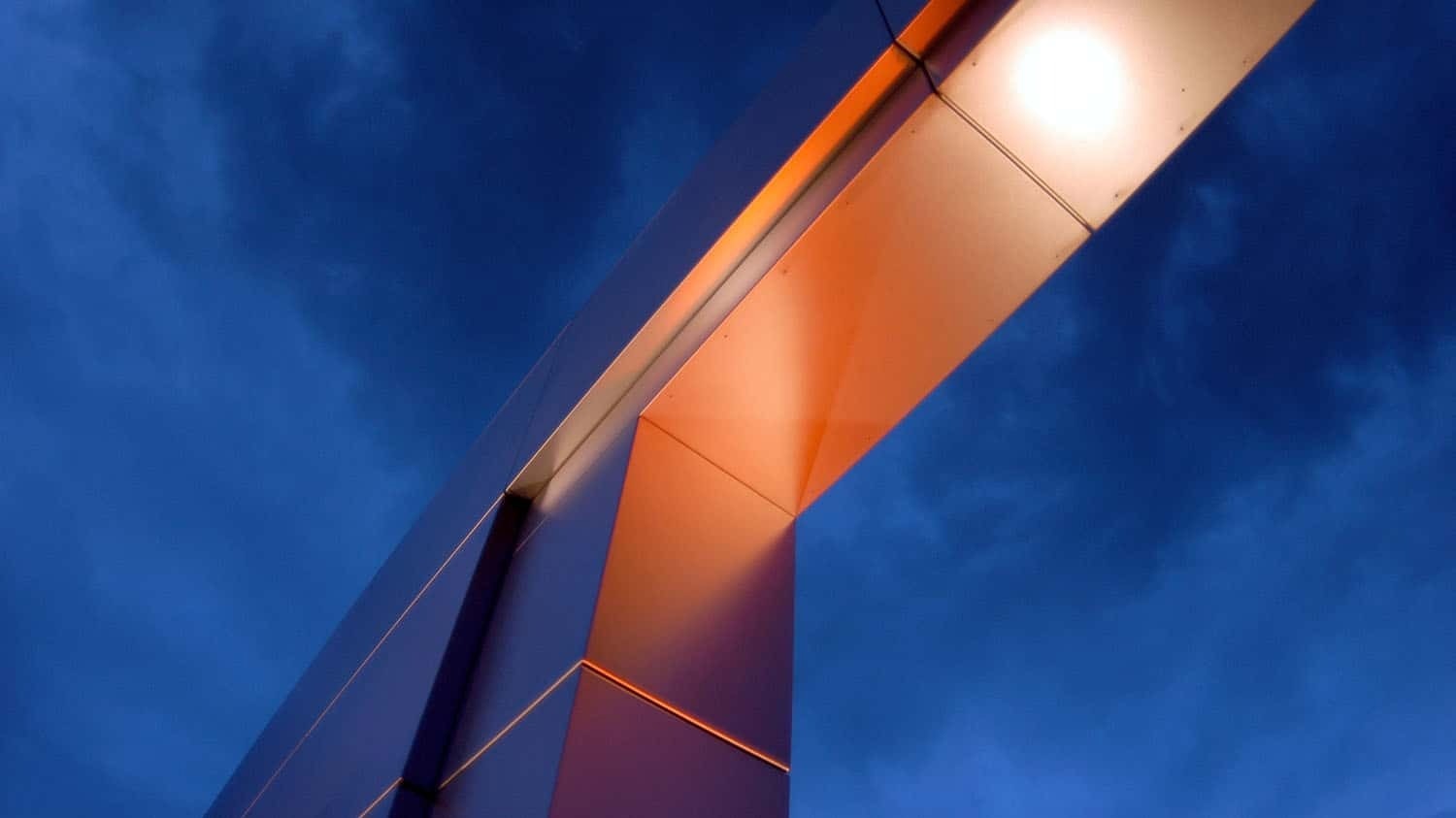
Image Credit: North Carolina State University
Scientists have come up with a new technique to aid artificial intelligence (AI) in extracting 3D information from 2D images, making cameras highly beneficial tools for such emerging technologies.
Existing techniques for extracting 3D information from 2D images are good, but not good enough. Our new method, called MonoXiver, can be used in conjunction with existing techniques – and makes them significantly more accurate.
Tianfu Wu, Study Co-Author and Associate Professor, Electrical and Computer Engineering, North Carolina State University
This work holds significant value for various applications, particularly in the context of autonomous vehicles. Cameras are a more cost-effective option compared to other tools like LIDAR, which relies on lasers to measure distances, making them an attractive choice for designers of autonomous vehicles who can incorporate multiple cameras to enhance system redundancy.
However, the effectiveness of this redundancy depends on the AI within the autonomous vehicle's ability to extract 3D navigational information from the 2D images captured by these cameras. MonoXiver plays a crucial role in addressing this challenge by enabling the extraction of 3D information from 2D images, thereby enhancing the capabilities of autonomous vehicles.
Existing methods that aim to extract 3D information from 2D images, like the MonoCon technique developed by Wu and his collaborators, rely on the use of "bounding boxes." In these approaches, AI systems are trained to analyze a 2D image and place 3D bounding boxes around objects within that image, such as individual cars on a street.
These bounding boxes take the form of cuboids, which are three-dimensional rectangular boxes with eight points, similar to the corners of a shoebox. The role of these bounding boxes is to assist the AI in estimating the dimensions of objects within the image and determining their spatial relationships.
Essentially, bounding boxes help the AI ascertain the size of a car and its position relative to other vehicles on the road.
Unlike existing programs where bounding boxes can be imperfect and may not encompass all parts of a vehicle or object present in a 2D image, the MonoXiver approach takes a different approach.
It utilizes each bounding box as a starting point, serving as an anchor for the AI's analysis. Subsequently, the AI conducts a second examination of the region surrounding each anchor bounding box. This secondary analysis results in the generation of multiple additional bounding boxes encompassing the anchor.
To determine which of these secondary boxes most effectively captures any "missing" portions of the object, the AI performs two key comparisons. The first comparison assesses the "geometry" of each secondary box to determine if it contains shapes consistent with those within the anchor box.
The second comparison evaluates the "appearance" of each secondary box to assess whether it exhibits colors or other visual characteristics that closely resemble those found within the anchor box. This comprehensive approach helps MonoXiver enhance the accuracy of object detection in 2D images and more effectively estimates object dimensions and positions.
One significant advance here is that MonoXiver allows us to run this top-down sampling technique – creating and analyzing the secondary bounding boxes–very efficiently.
Tianfu Wu, Study Co-Author and Associate Professor, Electrical and Computer Engineering, North Carolina State University
To quantify the precision of the MonoXiver method, the scientists tested it with the help of two datasets of 2D images: the well-established KITTI dataset and the highly challenging, large-scale Waymo dataset.
We used the MonoXiver method in conjunction with MonoCon and two other existing programs that are designed to extract 3D data from 2D images, and MonoXiver significantly improved the performance of all three programs. We got the best performance when using MonoXiver in conjunction with MonoCon.
Tianfu Wu, Study Co-Author and Associate Professor, Electrical and Computer Engineering, North Carolina State University
Wu added, “It’s also important to note that this improvement comes with relatively minor computational overhead. For example, MonoCon, by itself, can run at 55 frames per second. That slows down to 40 frames per second when you incorporate the MonoXiver method – which is still fast enough for practical utility.”
“We are excited about this work, and will continue to evaluate and fine-tune it for use in autonomous vehicles and other applications,” continued Wu.
The research received support from various organizations, including the US Army Research Office through grants W911NF1810295 and W911NF2210010, as well as the National Science Foundation through grants 1909644, 1822477, 2024688, and 2013451.