According to a new study performed by the National Institutes of Health, artificial intelligence (AI) and machine learning (ML) have the potential to effectively detect and diagnose Polycystic Ovary Syndrome (PCOS). This is known to be the most common hormone disorder among women, normally between ages 15 and 45.
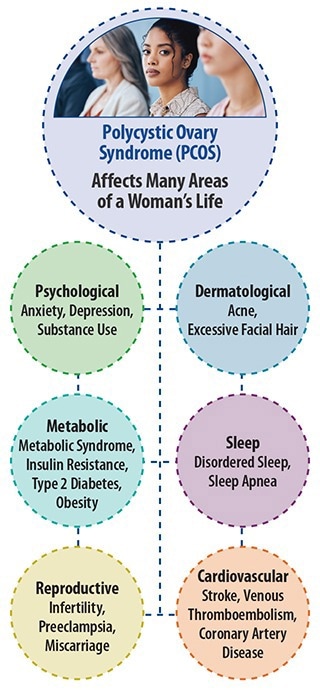
Image Credit: National Institutes of Health
Scientists systematically reviewed published scientific studies that made use of AI or ML to examine data to diagnose and categorize PCOS and found that AI/ML-based programs were able to detect PCOS successfully.
Given the large burden of under- and mis-diagnosed PCOS in the community and its potentially serious outcomes, we wanted to identify the utility of AI/ML in the identification of patients that may be at risk for PCOS. The effectiveness of AI and machine learning in detecting PCOS was even more impressive than we had thought.
Janet Hall MD, Study Co-Author, Senior Investigator and Endocrinologist, National Institute of Environmental Health Sciences
PCOS happens when the ovaries do not function properly and in several cases, is accompanied by high levels of testosterone. The disorder could result in extra facial hair, irregular periods, acne, or hair loss from the head.
Women with PCOS are frequently at an increased risk for developing type 2 diabetes, as well as sleep, cardiovascular, psychological, and other reproductive disorders like infertility and uterine cancer.
PCOS can be challenging to diagnose given its overlap with other conditions. These data reflect the untapped potential of incorporating AI/ML in electronic health records and other clinical settings to improve the diagnosis and care of women with PCOS.
Skand Shekhar MD, Study Senior Author, Assistant Research Physician and Endocrinologist, National Institute of Environmental Health Sciences
Study authors recommended combining huge population-based studies along with electronic health datasets and examining common laboratory tests to determine sensitive diagnostic biomarkers that could streamline the diagnosis of PCOS.
For the past few years, diagnosis has been based on extensively accepted standardized criteria that have developed but normally include clinical features (for example, excess hair growth, acne, and irregular periods) accompanied by radiological (for example, multiple small cysts and high ovarian volume on ovarian ultrasound) and laboratory findings (for example, high blood testosterone).
However, since few of the features of PCOS could co-occur with other disorders like diabetes, obesity, and cardiometabolic disorders, it always goes unidentified.
AI refers to the use of computer-based systems or tools to imitate human intelligence and to help make predictions or decisions. ML is known as a subdivision of AI focused on learning from earlier events and employing this insight in future decision-making.
AI could process huge amounts of diverse data, like that derived from electronic health records, thereby making it perfect assistance in the diagnosis of hard-to-diagnose disorders like PCOS.
The scientists performed a systematic review of all peer-reviewed studies reported on this topic for the past 25 years (1997-2022) that utilized AI or ML to detect PCOS. Using an experienced NIH librarian, the scientists determine possibly eligible studies.
On the whole, the scientists screened 135 studies and included 31 in this investigation. All studies were observational and evaluated the use of AI/ML technologies on patient diagnosis. Ultrasound images were included in around half the studies. The average age of the participants was 29.
Among the ten studies that made use of standardized diagnostic criteria for PCOS diagnosis, the precision of detection ranged from 80% to 90%.
Across a range of diagnostic and classification modalities, there was an extremely high performance of AI/ML in detecting PCOS, which is the most important takeaway of our study.
Skand Shekhar MD, Study Senior Author and Assistant Research Physician and Endocrinologist, National Institute of Environmental Health Sciences
The authors observe that AI or ML-based programs have the ability to considerably improve the capability to determine women with PCOS early, with associated cost savings and a decreased burden of PCOS on patients and also on the health system.
Follow-up studies with robust validation and testing practices will allow for the smooth integration of AI/ML for chronic health conditions.
Several NIEHS clinical studies focus on understanding and detecting PCOS.
This study was financially supported by the Intramural Research Program of the NIH/National Institute of Environmental Health Sciences (ZIDES102465 and ZIDES103323).
Journal Reference
Barrera, F. J., et al. (2023) Application of machine learning and artificial intelligence in the diagnosis and classification of polycystic ovarian syndrome: a systematic review. Frontiers in Endocrinology. doi.org/10.3389/fendo.2023.1106625.