In a landmark for artificial intelligence (AI), the AI system “Swift,” developed by University of Zurich (UZH) scientists, has beaten the world champions in drone racing—an outcome that seemed impossible just a few years ago.
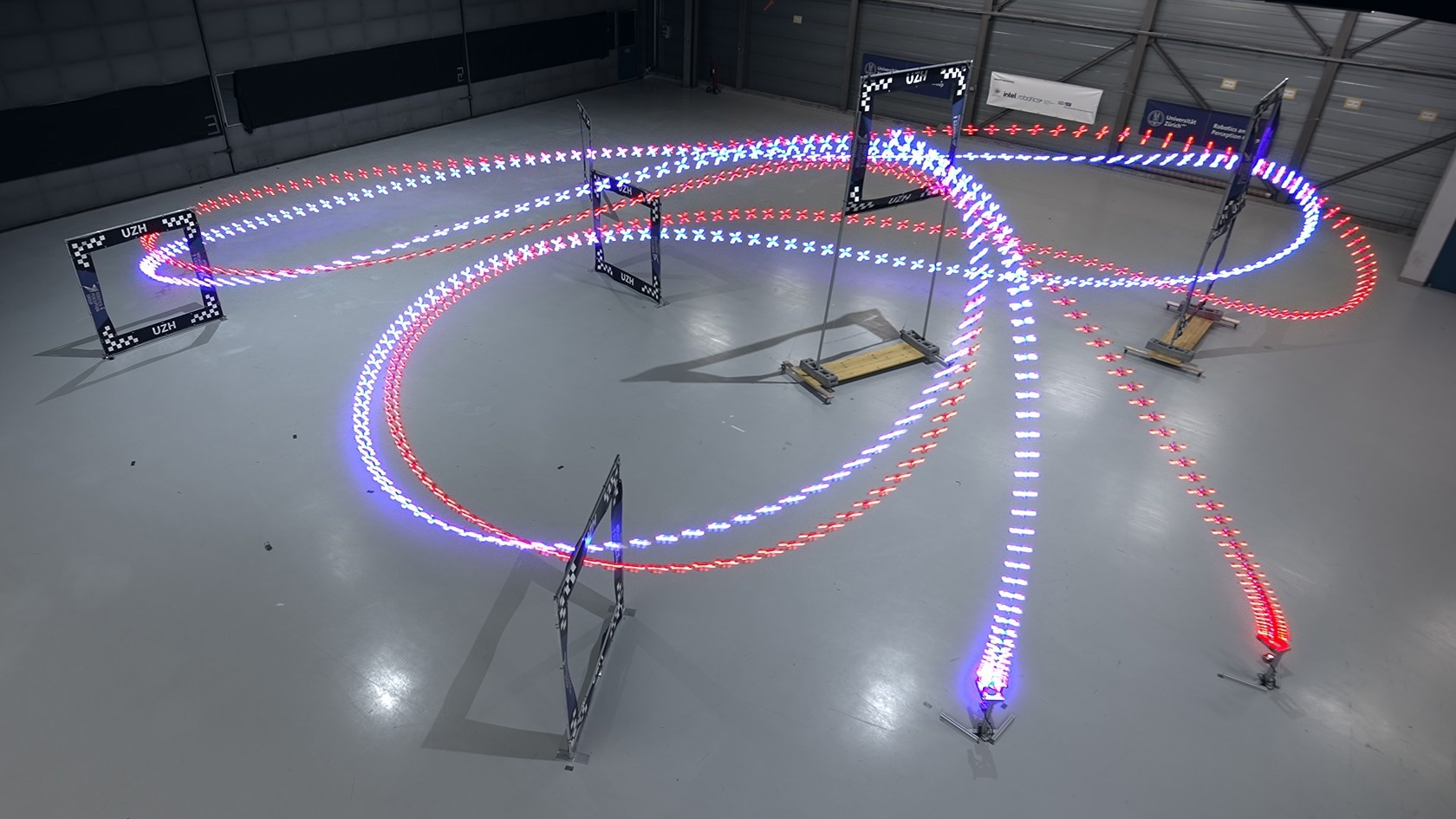
The AI-trained autonomous drone (in blue) managed the fastest lap overall, half a second ahead of the best time of a human pilot. Image Credit: UZH / Leonard Bauersfeld
The AI-piloted drone was trained in simulated surroundings. Real-world applications consist of disaster response or environmental monitoring.
Remember when IBM’s Deep Blue won against Gary Kasparov at chess in 1996, or Google’s AlphaGo crushed the top champion, Lee Sedol, at Go, a highly complicated game, in 2016?
In the history of artificial intelligence, such competitions where machines triumphed over human champions are the main milestones. Now, a research group from the University of Zurich and Intel has set a new milestone with the first autonomous system that has the potential to beat human champions in a physical sport: drone racing.
The AI system, known as Swift, won multiple races against three world-class champions in first-person view (FPV) drone racing. In this context, pilots fly quadcopters at speeds surpassing 100 km/hour, thereby regulating them remotely while wearing a headset that has been linked to an onboard camera.
Learning by Interacting With the Physical World
Physical sports are more challenging for AI because they are less predictable than board or video games. We don’t have a perfect knowledge of the drone and environment models, so the AI needs to learn them by interacting with the physical world.
Davide Scaramuzza, Head of the Robotics and Perception Group, University of Zurich
Scaramuzza is the newly minted drone racing team captain.
Recently, autonomous drones took around twice as long as those piloted by humans to fly through a racetrack as they depended on an external position-tracking system to regulate their trajectories.
But Swift reacts in real-time to the data gathered by an onboard camera, like the one utilized by human racers. Its combined inertial measurement unit quantifies speed and acceleration while an artificial neural network makes use of data from the camera to localize the drone in space and then detect the gates together with the racetrack.
This data has been fed to a control unit, also based on a deep neural network that selects the best action to complete the circuit as quickly as possible.
Training in an Optimized Simulation Environment
Swift was trained in simulated surroundings where it taught itself to fly by trial and error, using a type of machine learning called reinforcement learning. The usage of simulation helped avoid destroying multiple drones in the early stages of learning when the system often crashes.
To make sure that the consequences of actions in the simulator were as close as possible to the ones in the real world, we designed a method to optimize the simulator with real data.
Elia Kaufmann, Study First Author, University of Zurich
In this phase, the drone flew autonomously, thanks to very precise positions provided by an external position-tracking system, while also recording data from its camera. This approach was able to autocorrect errors it made, rendering data from the onboard sensors.
Human Pilots Still Adapt Better to Changing Conditions
Following a month of simulated flight time, Swift was all set to challenge its human competitors: the 2019 Drone Racing League champion Alex Vanover, the 2019 MultiGP Drone Racing champion Thomas Bitmatta, and three-time Swiss champion Marvin Schaepper.
The races happen between 5 and 13 June 2022 on a purpose-built track in a hangar of the Dübendorf Airport, next to Zurich.
The track spanned an area of 25 by 25 m, along with seven square gates that had to be passed in the correct order to finish a lap, including difficult maneuvers such as a Split-S, an acrobatic feature that includes half-rolling the drone and performing a descending half-loop at complete speed.
On the whole, Swift obtained the quickest lap, with a half-second lead over the best lap by a human pilot. On the contrary, human pilots proved more adaptable compared to the autonomous drone, which failed when the conditions were distinct from what it was trained for, for example, if there was excessive light in the room.
Drones have a limited battery capacity; they need most of their energy just to stay airborne. Thus, by flying faster we increase their utility.
Davide Scaramuzza, Head of the Robotics and Perception Group, University of Zurich
In applications like space exploration or forest monitoring, for instance, flying fast is important to cover large spaces in a limited time. As far as the film industry is concerned, quick autonomous drones can be utilized for shooting action scenes. Also, the potential to fly at high speeds could make a huge change for rescue drones that have been sent inside a building on fire.
Journal Reference
Kaufmann, E., et al. (2023) Champion-level drone racing using deep reinforcement learning. Nature. doi.org/10.1038/s41586-023-06419-4.