Under the guidance of Nagoya University in Japan, a research group has successfully anticipated crystal orientation by teaching artificial intelligence (AI) utilizing optical photographs of polycrystalline materials.
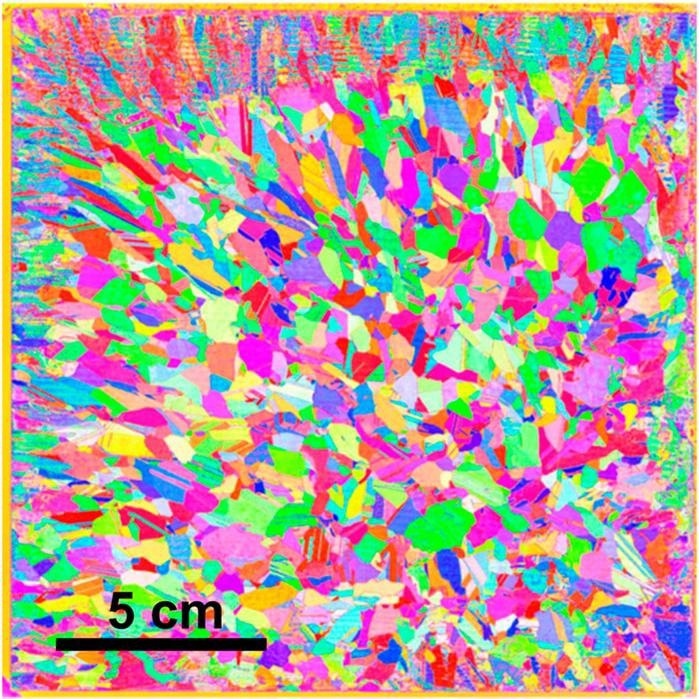
An example of the crystal grain orientations predicted by the AI-based technique. The color represents the orientation of the grain. Image Credit: Dr. Takuto Kojima
The outcomes have been reported in the journal APL Machine Learning.
For various machines, crystals are a crucial component. Familiar materials utilized in industry consist of polycrystalline components, such as ceramics, semiconductors, and metal alloys.
Since polycrystals are made up of several crystals, they have a complicated microstructure, and their properties change considerably based on how the crystal grains have been orientated. Particularly, this is significant for the silicon crystals utilized in computers, smartphones, and solar cells.
To obtain a polycrystalline material that can be used effectively in industry, control and measurement of grain orientation distribution is required. However, this is hindered by the expensive equipment and time current techniques needed to measure large-area samples.
Noritaka Usami, Professor, Nagoya University
A Nagoya University group, including Professor Usami (he, him) from the Graduate School of Engineering and Professor Hiroaki Kudo (he, him) from the Graduate School of Informatics, in partnership with RIKEN, has employed a machine learning model that evaluates photographs that have been taken by illuminating the surface of a polycrystalline silicon material from several directions. They discovered that the AI successfully forecasted the grain orientation distribution.
The time required for this measurement was about 1.5 hours for taking optical photographs, training the machine learning model, and predicting the orientation, which is much faster than conventional techniques, which take about 14 hours. It also enables measurement of large-area materials that were impossible with conventional methods.
Noritaka Usami, Professor, Nagoya University
In the wider industry, Usami has a firm belief in the use of the team’s method.
This is a technology that will revolutionize materials development. This research is intended for all researchers and engineers who develop polycrystalline materials.
Noritaka Usami, Professor, Nagoya University
Usami added, “It would be possible to manufacture an orientation analysis system of polycrystalline materials that packages an image data collection and a crystal orientation prediction model based on machine learning. We expect that many companies dealing with polycrystalline materials would install such equipment.”
Journal Reference
Hara, K., et al. (2023) A machine learning-based prediction of crystal orientations for multicrystalline materials. APL Machine Learning. doi.org/10.1063/5.0138099.