The effort of several years is required in the pharmaceutical industry to make medicines that have the potential to treat or cure human disease. But a new study performed has indicated that making use of generative artificial intelligence could immensely expedite the process of drug development.
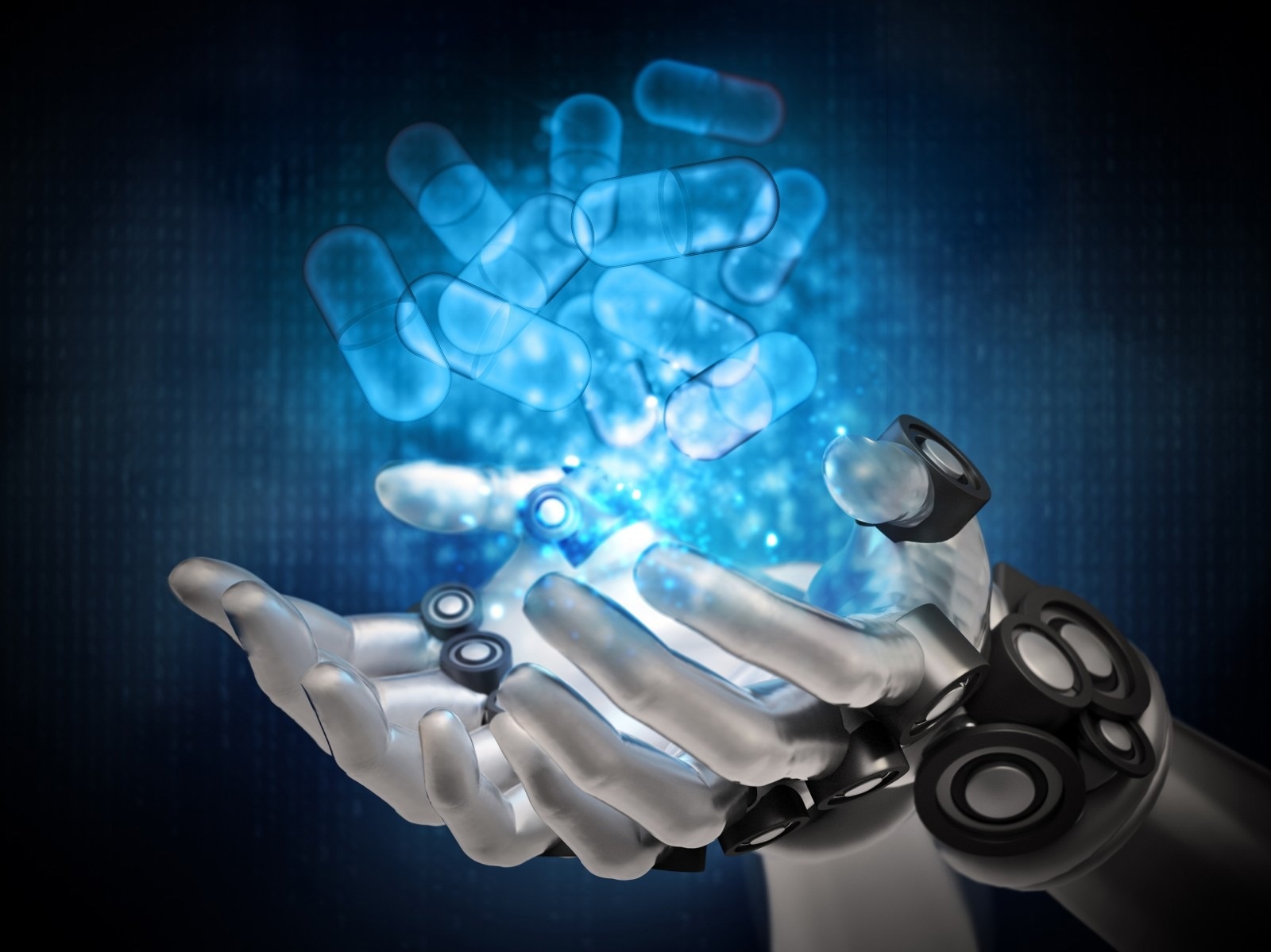
With the help of AI, drugs could be produced significantly faster and for much cheaper. Image Credit: Getty Images
At present, the majority of drug discovery is performed by human chemists who depend on their knowledge and experience to choose and synthesize the right molecules required to become the safe and effective medicines one relies on.
For the synthesis paths to be determined, a method known as retrosynthesis has been employed by scientists—a technique for making potential drugs by working backward from the preferred molecules and looking for chemical reactions to make them.
Moreover, as sifting through millions of potential chemical reactions could be an extremely difficult and tedious endeavor, scientists at The Ohio State University have made an AI framework known as G2Retro to automatically produce reactions for any provided molecule.
The new study has disclosed that compared to present manual-planning methods, the framework had the potential to cover a huge range of possible chemical reactions as well as precisely and rapidly perceive which reactions may work best to make a given drug molecule.
Using AI for things critical to saving human lives, such as medicine, is what we really want to focus on. Our aim was to use AI to accelerate the drug design process, and we found that it not only saves researchers time and money but provides drug candidates that may have much better properties than any molecules that exist in nature.
Xia Ning, Study Lead Author and Associate Professor, Computer Science and Engineering, Ohio State University
This study builds on an earlier study by Ning where her team came up with an approach named Modof that was capable of generating molecule structures that displayed preferred properties compared to any present molecules.
“Now the question becomes how to make such generated molecules, and that is where this new study shines,” stated Ning, also an associate professor of biomedical informatics at the College of Medicine.
The study was reported in the Communications Chemistry journal.
The research group of Ning trained G2Retro on a dataset comprising 40,000 chemical reactions gathered between 1976 and 2016. The framework “learns” from graph-based representations of provided molecules and makes use of deep neural networks to produce possible reactant structures that can be utilized for them to be synthesized.
According to Ning, its generative power is highly impressive that once provided a molecule, G2Retro could arise with hundreds of new reaction predictions in just a few minutes.
Our generative AI method G2Retro is able to supply multiple different synthesis routes and options, as well as a way to rank different options for each molecule. This is not going to replace current lab-based experiments, but it will offer more and better drug options so experiments can be prioritized and focused much faster.
Xia Ning, Study Lead Author and Associate Professor, Computer Science and Engineering, Ohio State University
To additionally test the effectiveness of AI, a case study was performed by Ning’s team to see if G2Retro could precisely forecast four newly released drugs that are already in circulation: Mitapivat, a medication utilized to treat hemolytic anemia; Tapinarof, which is utilized to treat several skin diseases; Mavacamten, a drug to treat systemic heart failure; and Oteseconazole, utilized to treat fungal infections in females.
G2Retro was able to properly produce precisely the same patented synthesis routes for such medicines and offered alternative synthesis routes that are also possible and synthetically useful, stated Ning.
The availability of such a dynamic and effective device at researchers’ disposal could allow the industry to manufacture powerful drugs at a rapid pace—however, despite the edge AI may give researchers within the laboratory, Ning stresses the medicines G2Retro or any generative AI makes still need to be validated. It is a process that includes the created molecules being tested in animal models and later in human trials.
We are very excited about generative AI for medicine, and we are dedicated to using AI responsibly to improve human health.
Xia Ning, Study Lead Author and Associate Professor, Computer Science and Engineering, Ohio State University
This study was financially supported by Ohio State’s President’s Research Excellence Program and the National Science Foundation. Other co-authors of the Ohio State were Ziqi Chen, Oluwatosin Ayinde, James Fuchs, and Huan Sun.
Journal Reference
Chen, Z., et al. (2023) G2Retro as a two-step graph generative models for retrosynthesis prediction. Communications Chemistry. https://doi.org/10.1038/s42004-023-00897-3.