Automated robot-guided surgery and minimally invasive surgery are possible to execute under extreme conditions, which need new requirements on intraoperative information acquisition abilities.
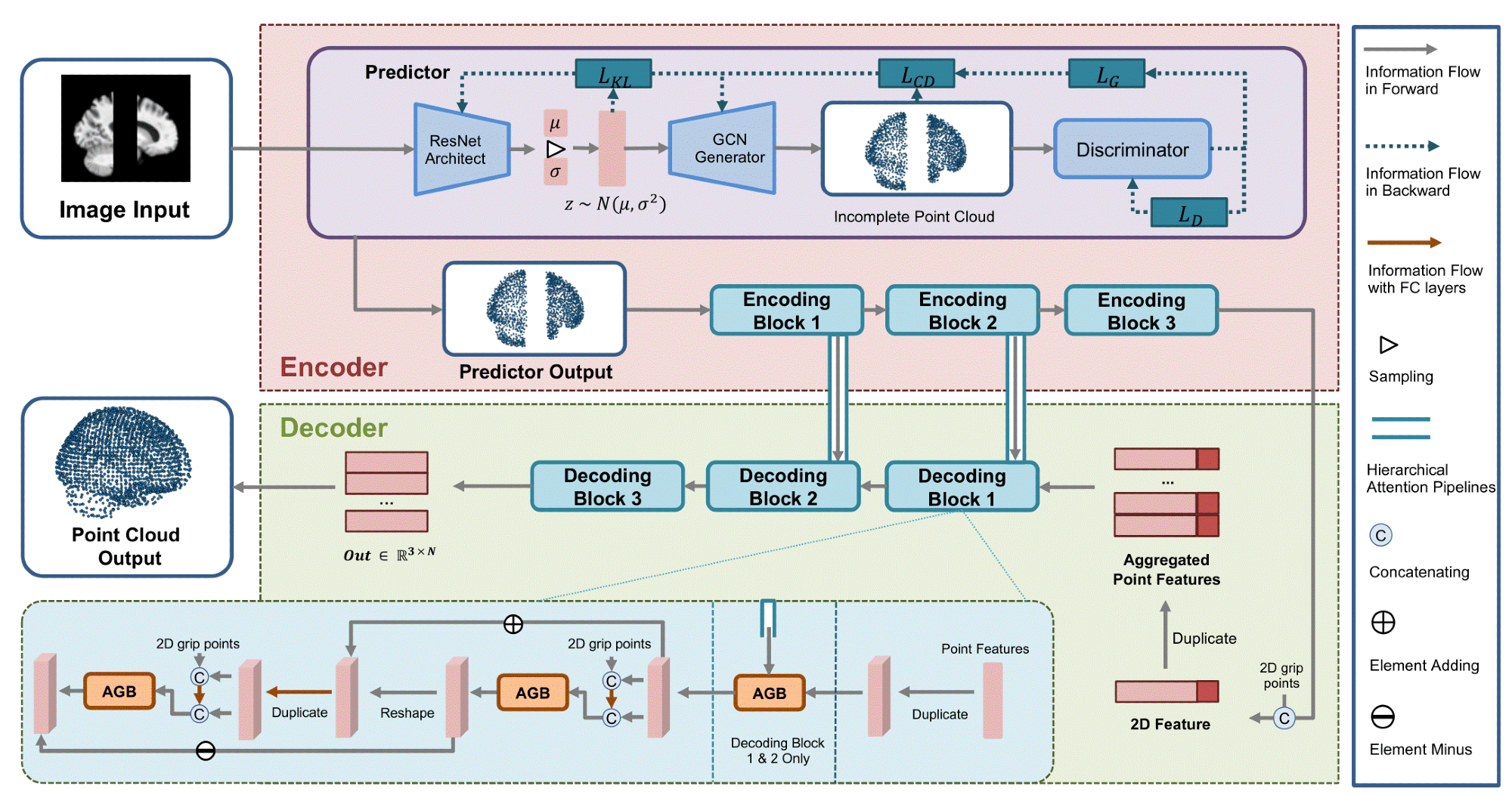
Architecture of HSPN. Image Credit: Shenzhen Institute of Advanced Technology
Three-dimensional (3D) brain point cloud generation plays a vital role in defeating the visual limitations of such surgeries.
In the field of 3D brain point cloud generation, one of the applications of generative AI is present. By making use of deep learning algorithms, the 3D point cloud of the patient’s brain could be produced for reconstruction at the time of minimally invasive surgery. But, a few traditional present point cloud reconstruction techniques still have restrictions when applied instantly to real surgical scenarios.
Currently, a research group headed by Professor Shuqiang Wang from the Shenzhen Institute of Advanced Technology (SIAT) of the Chinese Academy of Sciences has suggested a hierarchical shape-perception network (HSPN) for improved 3D brain reconstruction.
The study was reported in the journal IEEE Transactions on Neural Networks and Learning Systems on May 11th, 2023.
The suggested framework of HSPN comprises an encoder, which has a corresponding decoder, a predictor, and several encoding blocks. The predictor is built to produce point clouds that precisely explain the unfinished images and further complete such point clouds with high quality.
Taking the unified information flow into account for communicating with adjacent modules, the scientists built a hierarchical attention pipeline to send local attention features between decoding and encoding blocks.
At the same time, they came up with attention gate blocks (AGBs) in encoding and decoding blocks to effectively whole local geometric features of incomplete point clouds shifted by internal features and hierarchical attention pipelines of rebuild point clouds.
The employment of AGB can considerably increase the elaborate expression potential while decreasing generation error and improving stability.
3D shape perception and completion can be achieved spontaneously using the suggested HSPN. Extensive outcomes quantified by Chamfer distance and Point Cloud-to-Point Cloud (PC-to-PC) error illustrated that the planned HSPN surpassed other competitive techniques concerning qualitative displays, quantitative experiments, and classification evaluation.
The proposed method has a significantly short inference time, which enables effective real-time feedback of local image properties. This feedback can guide doctors to find diagnostically valuable surgical locations.
Shuqiang Wang, Shenzhen Institute of Advanced Technology, Chinese Academy of Sciences
Journal Reference
Hu, B., et al. (2023) 3-D Brain Reconstruction by Hierarchical Shape-Perception Network From a Single Incomplete Image. IEEE Transactions on Neural Networks and Learning Systems. https://doi.org/10.1109/TNNLS.2023.3266819.