An algorithm built with the use of AI could soon be utilized by doctors to detect heart attacks with improved accuracy and speed, as per a new study from the University of Edinburgh, financially supported by British Heart Foundation and the National Institute for Health and Care Research, and published in the journal Nature Medicine.
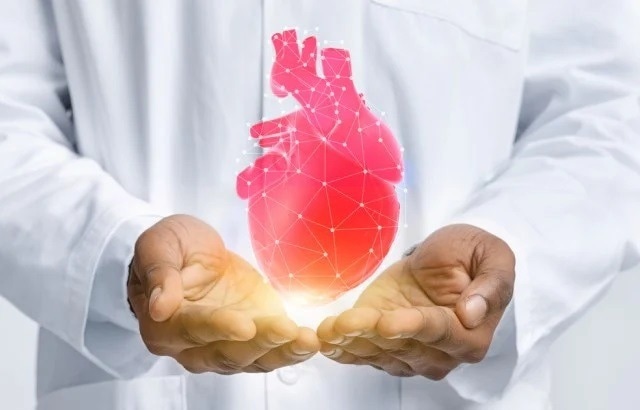
Image Credit: British Heart Foundation
The algorithm’s efficiency, known as CoDE-ACS, was tested on 10,286 patients residing in six countries across the globe. Scientists discovered that, when comparing the existing testing approaches, CoDE-ACS could rule out a heart attack in over double the number of patients, with a precision of 99.6%.
This capacity to rule out a heart attack quicker than ever before has the potential to largely minimize hospital admissions. Now, clinical trials are ongoing in Scotland with aid from the Wellcome Leap to verify if the tool can aid doctors in minimizing pressure on the overcrowded Emergency Departments.
Tackling Inequality
Alongside ruling out heart attacks in patients rapidly, CoDE-ACS also has the potential to aid doctors in determining those whose abnormal troponin levels were the result of a heart attack instead of any other condition.
The AI tool functioned well irrespective of sex, age, or pre-existing health conditions, establishing its likelihood of minimizing inequalities and misdiagnosis throughout the population.
CoDE-ACS could make emergency care more effective and successful by quickly finding patients who are safe to go home and by emphasizing to doctors all those who need to remain in the hospital for more tests.
The existing gold standard for detecting a heart attack is quantifying protein troponin levels in the blood. However, for every patient, the same threshold is employed. This implies that aspects like sex, age, and other health issues, which impact troponin levels are not given consideration, impacting how precise heart attack detections are.
This can result in inequalities in diagnosis. For instance, an earlier BHF-funded study has exhibited that women are 50% more likely to receive an incorrect initial detection. People who are misdiagnosed at first have a 70% increased risk of dying after 30 days. In order to prevent this, the new algorithm is a chance.
AI to Save Lives
CoDE-ACS was built with the use of information from 10,038 patients in Scotland who had reached the hospital with an assumed heart attack. It employs regularly gathered patient data, such as sex, age, ECG results, and medical history, along with troponin levels, to foresee the likelihood that a person has had a heart attack. The finding is a probability score between 0 and 100 for every patient.
For patients with acute chest pain due to a heart attack, early diagnosis and treatment saves lives. Unfortunately, many conditions cause these common symptoms, and the diagnosis is not always straight forward. Harnessing data and artificial intelligence to support clinical decisions has enormous potential to improve care for patients and efficiency in our busy Emergency Departments.
Nicholas Mills, Study Lead Author and Professor, Cardiology, Centre for Cardiovascular Science, University of Edinburgh
Chest pain is one of the most common reasons that people present to Emergency Departments. Every day, doctors around the world face the challenge of separating patients whose pain is due to a heart attack from those whose pain is due to something less serious. CoDE-ACS, developed using cutting edge data science and AI, has the potential to rule-in or rule-out a heart attack more accurately than current approaches. It could be transformational for Emergency Departments, shortening the time needed to make a diagnosis, and much better for patients.
Professor Sir Nilesh Samani, Medical Director, British Heart Foundation
Journal Reference
Doudesis, D., et al. (2023). Machine learning for diagnosis of myocardial infarction using cardiac troponin concentrations. Nature Medicine. https://doi.org/10.1038/s41591-023-02325-4.