Scientists from the Max-Planck-Institut für Eisenforschung (MPIE) examine the status of physics-based modeling and discuss how integrating such methods with artificial intelligence could set the stage for untapped spaces in the design of complicated materials. They reported their findings in the Nature Computational Science journal.
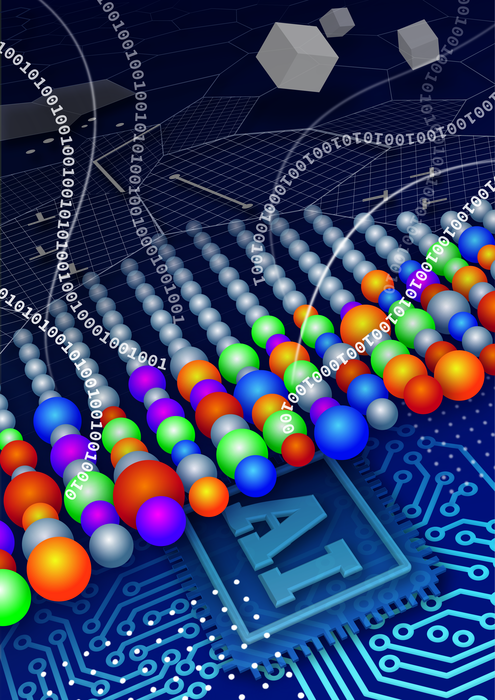
Merging physics-based simulations with artificial intelligence gains increasing importance in materials science, especially for the design of complex materials that meet technological and environmental demands. Image Credit: T. You, Max-Planck-Institut für Eisenforschung GmbH.
Combining Physics-Based Approaches with Artificial Intelligence
To fulfill the requirements of technological and environmental problems, increasingly demanding and multifold material properties have to be taken into account. This is responsible for making alloys highly complicated concerning synthesis, composition, processing, and recycling.
Variations in such parameters involve changes in their microstructure, which directly affects the properties of the materials. There is a need for this complexity to be understood to allow forecasting structures and materials’ properties. In this context, computational materials design methods play a vital role.
Our means of designing new materials rely today exclusively on physics-based simulations and experiments. This approach can experience certain limits when it comes to the quantitative prediction of high-dimensional phase equilibria and particularly to the resulting non-equilibrium microstructures and properties.
Dierk Raabe, Study First Author, Director and Professor, Max-Planck-Institut für Eisenforschung
Raabe added, “Moreover, many microstructure- and property-related models use simplified approximations and rely on a large number of variables. However, the question remains if and how these degrees of freedom are still capable of covering the material’s complexity.”
The study makes a comparison of the physics-based simulations, similar to the molecular dynamics and ab initio simulations, with advanced artificial intelligence and descriptor-based modeling methods.
The use of artificial intelligence (AI) has numerous benefits, even though physics-based simulations are often too expensive to forecast materials with complicated compositions.
AI is capable of automatically extracting thermodynamic and microstructural features from large data sets obtained from electronic, atomistic, and continuum simulations with high predictive power.
Jörg Neugebauer, Study Co-Author, Professor and Director, Max-Planck-Institut für Eisenforschung
Enhancing Machine Learning with Large Data Sets
Since the predictive power of artificial intelligence relies on the availability of large data sets, means to overcome this obstacle are required. One way is to make use of active learning cycles, where machine learning models have been trained with initially small subsets of labeled data.
Furthermore, the predictions of the model are screened by a labeling unit that feeds high-quality data back into the pool of labeled records, and the machine learning model is made to run again. This stepwise method results in a final high-quality data set that has been usable for precise predictions.
Yet, there are still many open questions for the usage of artificial intelligence in materials science: how to tackle noisy and sparse data? How to consider intriguing outliers or “misfits?” How to implement undesirable elemental intrusion from synthesis or recycling?
But, when designing compositionally complicated alloys is concerned, artificial intelligence will play a highly significant role very soon, particularly with the development of algorithms and the accessibility of high-quality material datasets and high-performance computing resources.
The study has been financially supported by the BigMax network of the Max Planck Society.
Journal Reference
Raabe, D., et al. (2023) Accelerating the design of compositionally complex materials via physics-informed artificial intelligence. Nature Computational Science. https://doi.org/10.1038/s43588-023-00412-7.