The gut microbiome consists of a complicated population of varied bacterial species that are necessary to human health.
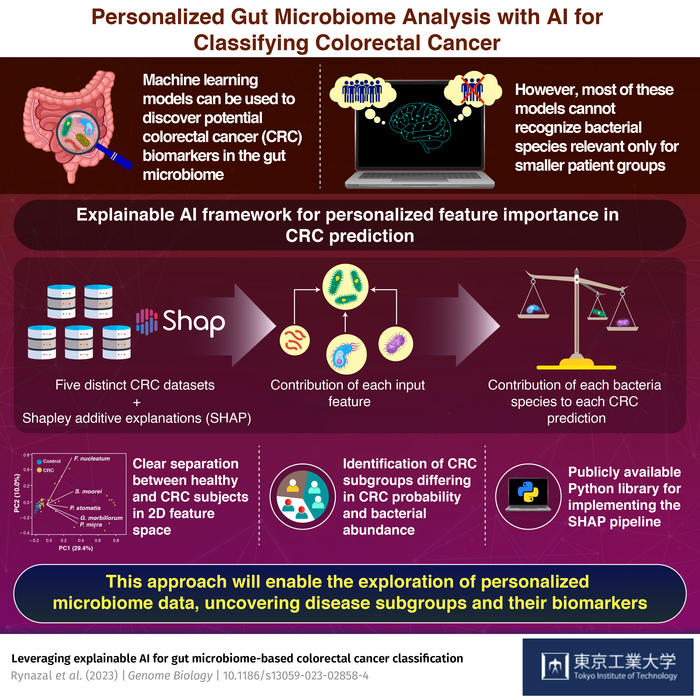
Image Credit: Tokyo Tech
In the past few years, researchers across numerous fields have discovered that variations in the gut microbiome could be linked to an extensive range of diseases, notably colorectal cancer (CRC).
Several studies performed have shown that a greater abundance bacteria like Parvimonas micra and Fusobacterium nucleatum, is normally linked to the progression of CRC.
Depending on such findings, scientists have come up with several artificial intelligence (AI) models to aid them in examining which bacterial species are beneficial as CRC biomarkers.
But, the majority of such models depend on what is called “global explanations,” implying that they can just consider the entirety of the input data to make forecasting. Consequently, such models are incapable of determining bacterial species that could be appropriate CRC biomarkers available for smaller and less-representative groups of patients.
Against this backdrop, a research group from the Tokyo Institute of Technology (Tokyo Tech), Japan, decided to adopt a different method that had the potential to fulfill this limitation. As defined in their paper, which was recently reported in the journal Genome Biology, an explainable AI framework was employed by the research group that offers local, instead of global, explanations for its CRC predictions.
Local explanation techniques make it possible to discover the most contributing bacteria for each individual CRC patient, enabling us to examine inter-individual differences between subjects within a disease group.
Takuji Yamada, Study Main Author and Associate Professor, Tokyo Institute of Technology
A framework known as “Shapley additive explanations” (SHAP) was utilized by the team, which was sourced from a concept in game theory named the Shapley value.
In simple terms, the Shapley value lets one gain insights into how a payout must be distributed amongst the players of a group or coalition. In their study, the team made use of SHAP to assess the contribution of every bacterial species to each separate CRC prediction.
By making use of this together with data achieved from five CRC datasets, the researchers discovered the fact that projecting the SHAP values into a two-dimensional (2D) space allowed them to get an obvious separation between healthy and CRC subjects.
Grouping this 2D information led to four subgroups of CRC subjects, each changing the CRC probability and the linked bacteria. Besides, the team discovered that subjects in the CRC subgroups having the greatest CRC probability always had an enhanced population of bacteria that is normally linked with CRC.
Most strikingly, the outcomes were persistent throughout the five datasets, demonstrating the extensive applicability of this technique.
The team expects their method to make solid contributions to the gut microbiome research community since they have such promising outcomes.
Considering the increasing use of machine learning in microbiome-disease association studies, our novel method could be beneficial for a more personalized microbiome data exploration as well as help uncover potential disease subgroups along with their potential associated biomarkers.
Takuji Yamada, Study Main Author and Associate Professor, Tokyo Institute of Technology
Furthermore, the technique is also relevant to other diseases with familiar links to the gut microbiome, like Chron’s disease, ulcerative colitis, and diabetes.
The researchers are hoping that the explainable AI will help disclose more such secrets of the gut microbiome in the immediate future.
Journal Reference
Rynazal, R., et al. (2023) Leveraging explainable AI for gut microbiome-based colorectal cancer classification. Genome Biology. https://doi.org/10.1186/s13059-023-02858-4.