Only a few known inorganic crystals have been computationally predicted or theoretically proven thus far. Researchers have presented a way for using artificial intelligence to find novel and highly efficient materials for sustainable energy technologies.
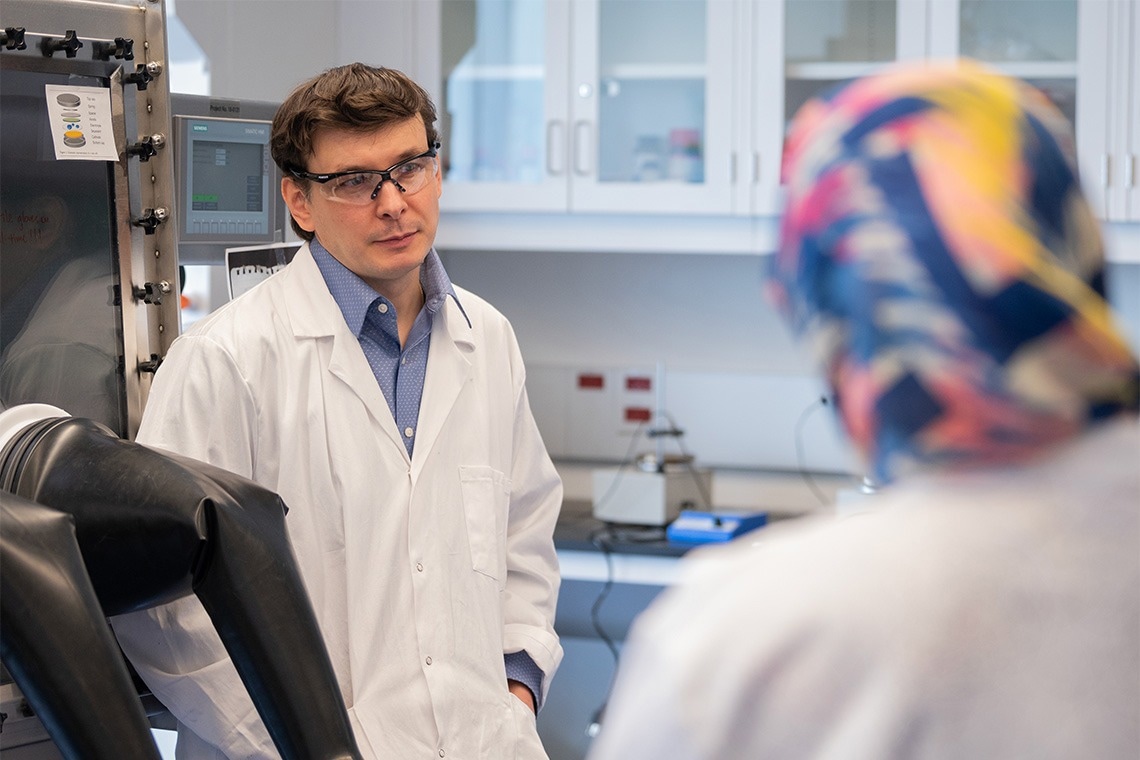
Alex Voznyy, an assistant professor at U of T Scarborough, right, speaks with a colleague (photo by Dylan Toombs)
A new study published in the journal Patterns discussed the use of machine learning to substantially reduce the time required to discover novel materials with specified qualities.
Discovering New Materials
The development of novel materials is essential for a variety of applications, including energy storage, electronic devices, catalysts, and biomedical materials, to name a few.
The properties of materials determine their suitability for a specific application, and the discovery of materials with novel properties is essential for the advancement of materials science.
Ab initio methods like Density Functional Theory (DFT) have been widely used to predict the properties of new materials, providing a powerful tool for materials discovery and design.
DFT calculates the electronic structure of materials by considering the interactions between electrons and the nucleus, providing insights into the stability, reactivity, and electronic properties of materials.
However, the high computational cost associated with DFT presents a significant challenge to the widespread use of this method for large-scale screening of new materials.
The computational demands of DFT increase with the size of the system, making it challenging to screen large numbers of materials in a reasonable amount of time.
To overcome this challenge, researchers are exploring alternative methods that can provide the accuracy of DFT while reducing computational costs.
How Can Machine Learning and AI Help?
Machine learning (ML) methods paired with high-throughput computations have been demonstrated to produce accurate forecasts of new material characteristics with increased speed and efficiency.
Alex Voznyy, an author of the research paper, said, “We want to be able to predict new materials faster and more efficiently so we can start physically creating these materials sooner and with greater certainty that they will work.”
Machine-learning models are becoming more efficient at determining the characteristics of inorganic crystals, including band gaps, formation energy, and bulk moduli. Nonetheless, screening for novel materials with required attributes requires knowledge of their equilibrium structures.
To extract ground-state structural details, an ML-based optimizer may be utilized; however, the model must be capable of distinguishing between high-energy and ground-state structures.
The ML model's predictive accuracy decreases when non-optimized structures are used. However, DFT must be used to generate relaxed structures, which defeats the purpose of employing machine learning in the first place.
Ideally, a force field with low computational cost would initially be used to relax the structure. The structure would then be input into the machine learning model, generating accurate predictions. A force field of this kind could potentially be based on machine learning.
To train such a model, data including deformed structures and, preferably, even the forces on the atoms would be required. Unfortunately, available public databases only provide the formation energies for DFT-optimized structures. By definition, the forces are already zero in this case.
Highlights of the Study
The team showed that utilizing publicly accessible data values for elasticity, which summarized the energy computations for several strained variants of the crystals, was adequate for training an energy predictor for strained structures based on machine learning.
The ML model learned the response to global strain and was capable of generalizing to local strains, thereby generating a force field that could be utilized to build a geometry optimizer.
All this was accomplished without the need for any extra DFT computations to create augmented training data.
A robust machine learning model and optimal equilibrium structures were necessary to analyze and estimate the stability of inorganic crystals appropriately.
The team generated uniaxial and hydrostatic strain responses from around 8000 materials at various strain levels.
The strained structural data augmentation approach presented in the research improved formation energy estimates for strained as well as undistorted materials.
The researchers also demonstrated that including global strains enhanced the ML model's comprehension of local strains.
They used this ML-based model as a geometry optimizer with a significant increase in prediction accuracy for features like band gaps and formation energy.
The strain-aware model enabled the development of an efficient optimizer. This method may be used to increase the performance of various inorganic crystal optimizers.
Opportunities for Further Improvements
Further research might try to enhance the model's accuracy by increasing the size as well as the complexity of the dataset.
Only about 5% of the Materials Project's accessible materials include acceptable elastic data, restricting the augmentation's potential overall impact. The data augmentation approach will improve with the availability of more data.
Furthermore, the model's complexity may be increased by including additional forms of deformations, including shear stresses that change the unit cell (and bond) angles.
Reference
Dinic, F., Wang, Z. et al. (2023). Strain data augmentation enables machine learning of inorganic crystal geometry optimization. Patterns. Available at: https://doi.org/10.1016/j.patter.2022.100663
Disclaimer: The views expressed here are those of the author expressed in their private capacity and do not necessarily represent the views of AZoM.com Limited T/A AZoNetwork the owner and operator of this website. This disclaimer forms part of the Terms and conditions of use of this website.