Genome editing technologies provide huge opportunities for the treatment of genetic diseases. Procedures such as the extensively used CRISPR/Cas9 gene scissors directly deal with the reason for disease in DNA.
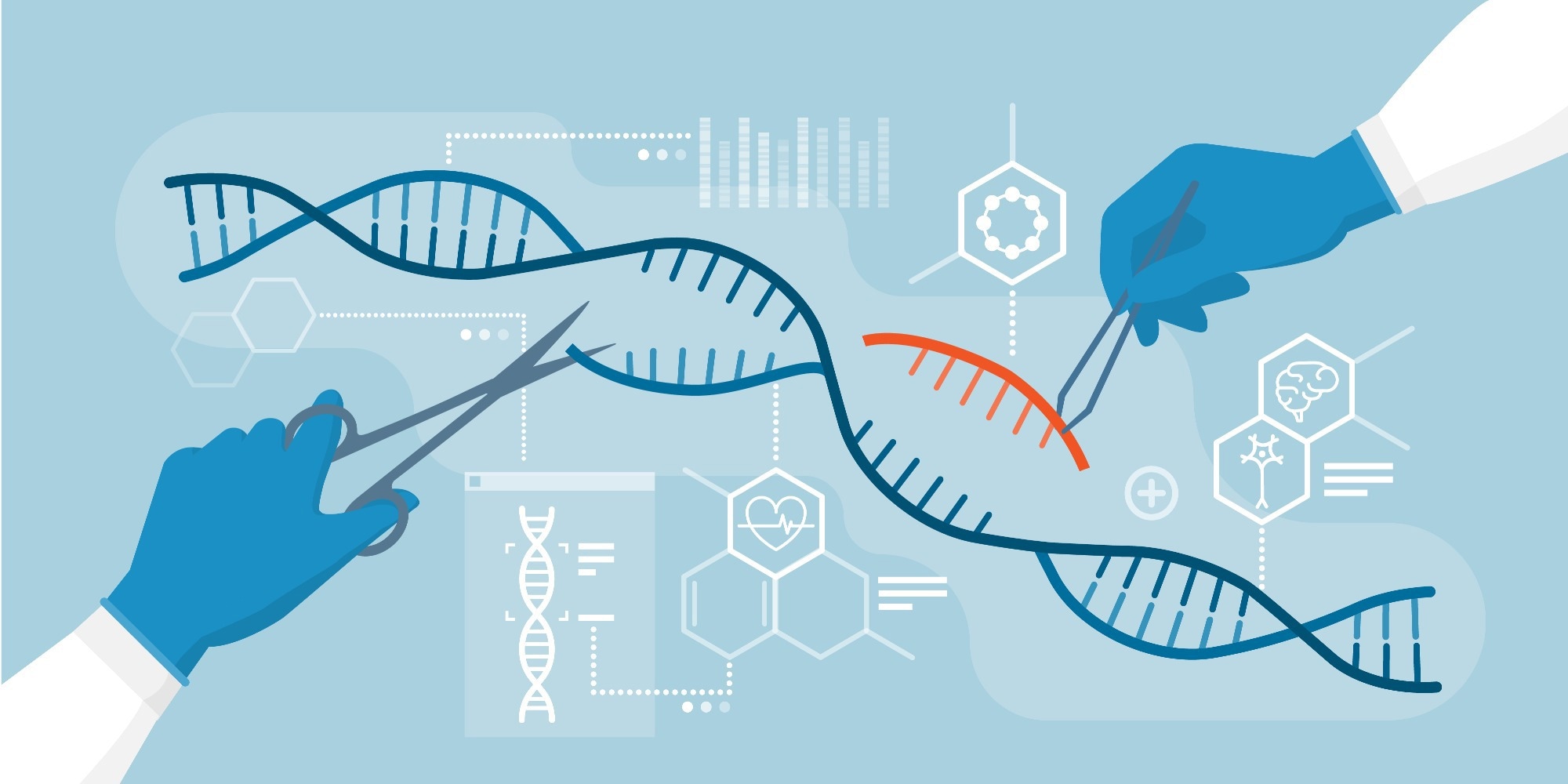
Image Credit: elenabsl/Shutterstock.com
The scissors are employed in the lab to carry out targeted alterations to the genetic matter in cell lines and model organisms and to analyze biological systems.
The advanced development of this standard CRISPR/Cas9 technique is known as prime editing. In contrast to the traditional gene scissors that form a break in the two strands of the DNA molecule, prime editing cuts and rectifies DNA on just one. The prime editing guide RNA (pegRNA) accurately targets the applicable site in the genome and offers the new genetic data, which is then transcribed by a “translation enzyme” and integrated into the DNA.
Finding the Most Efficient DNA Repair Options
Prime editing promises to be an operational technique for repairing disease-triggering mutations in patients’ genomes. However, regarding its successful application, it is crucial to minimize unplanned side effects like mistakes in DNA correction or modification of DNA in other areas of the genome.
According to preliminary studies, prime editing results in a considerably lower number of unplanned modifications than traditional CRISPR/Cas9 methods.
However, scientists still have to spend a substantial amount of time improving the pegRNA for direct targeting in the genome.
There are over 200 repair possibilities per mutation. In theory, we would have to test every single design option each time to find the most efficient and accurate pegRNA.
Gerald Schwank, Professor, Institute of Pharmacology and Toxicology, University of Zurich
Analyzing a Large Data Set with AI
Schwank and his research team were keen to discover a simpler solution. Along with Michael Krauthammer, UZH professor at the Department of Quantitative Biomedicine, they formulated a technique that can predict the efficiency of pegRNAs. By examining more than 100,000 different pegRNAs in human cells, they could produce a detailed prime editing data set.
This allowed them to determine which properties of a pegRNA—such as the sequence of DNA building blocks, the length of the DNA sequence, or the shape of the DNA molecule—positively or negatively impact the prime editing procedure.
Next, the team prepared an artificial intelligence (AI)-based algorithm to identify patterns in the pegRNA of significance for efficiency. Established on these patterns, the trained tool can forecast both the effectiveness and precision of genome editing with a specific pegRNA.
In other words, the algorithm can determine the most efficient pegRNA for correcting a particular mutation.
Michael Krauthammer, Professor, Department of Quantitative Biomedicine, University of Zurich
The tool has already been tested for its effectiveness in mice and human cells and is freely available to scientists.
Long-Term Goal: Repairing Hereditary Diseases
Additional pre-clinical studies are still required before the new prime editing tool can be administered to humans. However, the scientists are self-assured that, in the near future, it will be feasible to employ prime editing to treat the DNA mutations of common genetic diseases like cystic fibrosis, sickle cell anemia, or metabolic diseases.
Journal Reference
Mathis, M., et al. (2023) Predicting prime editing efficiency and product purity by deep learning. Nature Biotechnology. doi.org/ 10.1038/s41587-022-01613-7.