By making use of deep-learning, researchers have successfully built an inference and prediction system of the Indonesian Throughflow (ITF).
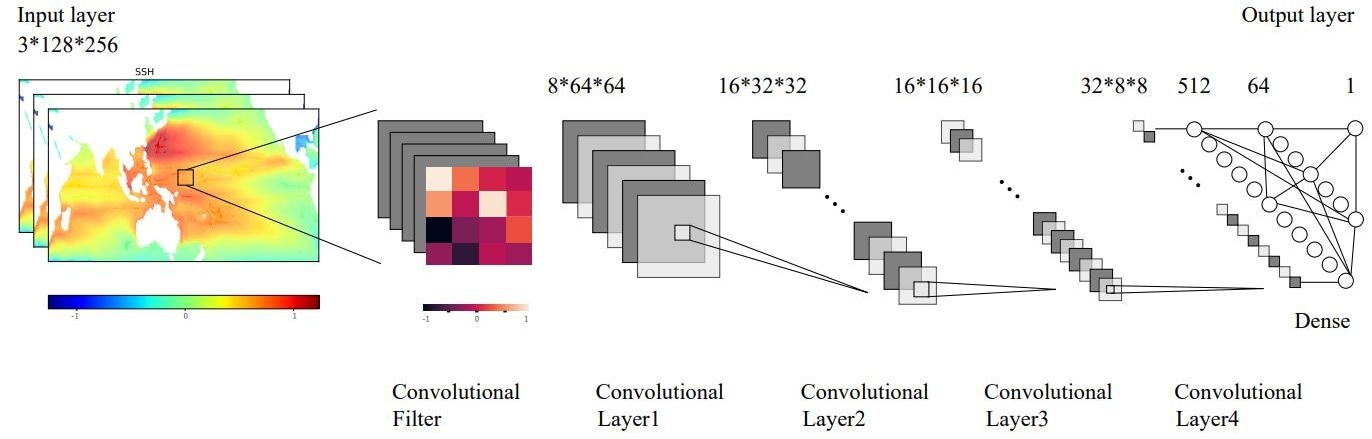
Architecture diagram of deep-learning. Image Credit: Institute of Oceanology of the Chinese Academy of Sciences.
This was done collaboratively by the researchers from the Institute of Oceanology of the Chinese Academy of Sciences (IOCAS) and Nanjing University of Information Science and Technology.
The study was reported in the Frontiers in Marine Science journal on January 16th, 2023.
The only channel linking the tropical ocean basins is the Indonesian seas, and the ITF is a chief ocean dynamic factor for the inter-basin exchange between the Pacific Ocean basin and the Indian Ocean basin.
The ITF plays a crucial role in the material and energy balance of regional and global climate change and the Indo-Pacific Ocean. However, the forecast of ITF primarily depends on numerical simulation systems, which repeatedly have great uncertainty and considerable model biases.
Accordingly, the scientists headed by Professor Shijian Hu propose the concept of integrating satellite observations along with artificial intelligence techniques to build the inference and prediction system of ITF and performed experiments with several deep-learning models.
The chief driving factor of ITF is the Indo-Pacific pressure gradient, so scientists utilized sea surface heights between the Indian and Pacific Ocean basins to deduce and forecast the ITF transport.
The convolutional neural network (CNN) was made to train with the enormous data gathered by the Coupled Model Intercomparison Project Phase 6 model and Simple Ocean Data Assimilation data sets and rebuilt a time series of ITF transport.
The training outcomes displayed that the system relying on the CNN model replicates around 90% of the complete variance of ITF transport. This denotes that the system has the potential to obtain valid inferences about ITF transport.
Furthermore, the scientists integrated the system using satellite data from 1993 to 2021 to gather and build the time series of ITF. Further, it was discovered that the time series was in a fair deal with the internationally celebrated ITF’s field observation data.
They examined the feasibility of forecasting ITF with this AI system, and the outcomes display that the system has the capacity to make a valid prediction with a leading time of almost seven months.
The ITF AI inference and prediction system provides an important tool for conducting research on ocean circulation and climate change in the Indo-Pacific Ocean, which may alleviate the pressure of field ocean observation to some extent.
Shijian Hu, Professor, Institute of Oceanology, Chinese Academy of Sciences
This study was financially supported by the Natural Science Foundation of Shandong Province and the Strategic Pilot Science and Technology Special Project of CAS.
Journal Reference:
Xin, L., et al. (2023) Making use of a deep learning approach to infer and forecast Indonesian Throughflow transport from sea surface height. Frontiers in Marine Science. doi.org/10.3389/fmars.2023.1079286.