According to a new study, AI might help enhance care for patients who come to the hospital with acute chest pain.
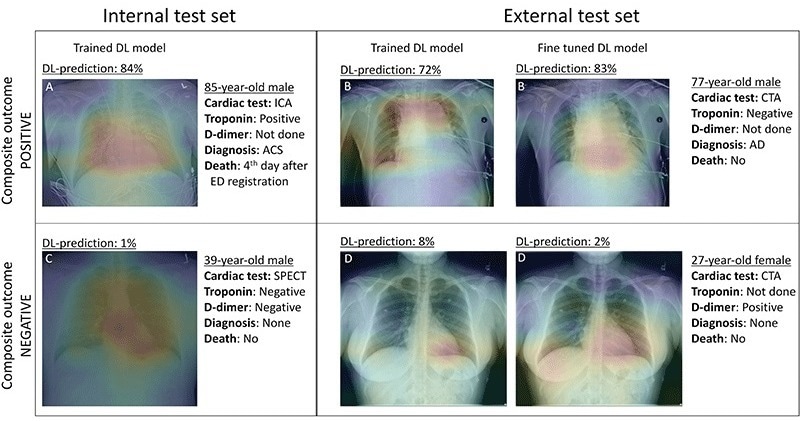
Gradient-weighted class activation maps of representative chest radiographs in (A) an 85-year-old man with acute coronary syndrome (ACS), (B) a 77-year-old man with aortic dissection (AD), (C) a 39-year-old healthy man, and (D) a 27-year-old healthy woman. The maps show which parts of the images influenced deep learning (DL) model predictions for the composite outcome. The color gradient shows the level of activation from that given area, where red indicates the highest activation, blue indicates the lowest activation, and no color indicates no activation. Areas of the heart and lungs contributed most model predictions. Fine-tuning improved the diagnostic accuracy of our DL model and resulted in more relevant areas contributing to predictions. ACS = acute coronary syndrome, CTA = coronary CT angiography, ICA = invasive coronary angiography, SPECT = single-photon emission CT. https://pubs.rsna.org/doi/10.1148/radiol.221926 ®Radiological Society of North America 2023
The study has been reported in the journal Radiology.
To the best of our knowledge, our deep learning AI model is the first to utilize chest X-rays to identify individuals among acute chest pain patients who need immediate medical attention.
Márton Kolossváry, MD, Ph.D., Study Lead Author and Radiology Research Fellow, Massachusetts General Hospital
Acute chest pain might refer to tightness, burning, or other pain in the chest, or severe pain that spreads to one’s neck, back, shoulders, jaw, or arms. It might be followed by shortness of breath.
More than 7 million emergency department visits have been a result of acute chest pain syndrome annually in the United States (U.S.). Hence, this is considered to be one of the most common complaints.
Below 8% of these patients are diagnosed with the three main cardiovascular causes of acute chest pain syndrome, which are acute coronary syndrome, aortic dissection, or pulmonary embolism.
As emergency departments face difficulties with shortages of hospital beds and high patient numbers, efficiently triaging patients at very low risk of such serious conditions is necessary.
Deep learning could be trained to search X-Ray images to find patterns linked to the disease.
Model Maintained Diagnostic Accuracy Across Age, Sex, Ethnicity, and Race
For the study, Dr. Kolossváry and collaborators came up with an open-source deep-learning model to determine patients with acute chest pain syndrome who were at risk for 30-day pulmonary embolism, acute coronary syndrome, all-cause mortality, or aortic dissection depending on a chest X-Ray.
The study utilized electronic health records of patients presenting with acute chest pain syndrome who had a chest X-Ray and extra cardiovascular or pulmonary imaging and/or stress tests at MGH or Brigham and Women’s Hospital in Boston between January 2005 and December 2015. Five thousand seven hundred fifty patients (average age 59, including 3,329 men) were assessed for the study.
The deep-learning model was made to train 23,005 patients from MGH to forecast a 30-day composite endpoint of an acute coronary syndrome, aortic dissection or pulmonary embolism, and all-cause mortality depending on chest X-Ray images.
The deep-learning tool considerably enhanced the forecast of these negative outcomes beyond sex, age, and traditional clinical markers, like d-dimer blood tests. The model retained its diagnostic accuracy across sex, age, race, and ethnicity.
The model could defer extra testing in 14% of patients as compared to 2% while using a model just by integrating sex, age, and biomarker data. This was done using a 99% sensitivity threshold.
Analyzing the initial chest X-Ray of these patients using our automated deep learning model, we were able to provide more accurate predictions regarding patient outcomes as compared to a model that uses age, sex, troponin, or d-dimer information. Our results show that chest X-Rays could be used to help triage chest pain patients in the emergency department.
Márton Kolossváry, MD, PhD, Study Lead Author and Radiology Research Fellow, Massachusetts General Hospital
Dr. Kolossváry stated that, in the future, such an automated model could examine chest X-Rays in the background and help choose those who would profit the most from immediate medical attention. Also, it may help find patients who might be discharged safely from the emergency unit.
Journal Reference:
Kolossváry, M., et al. (2022) Deep Learning Analysis of Chest Radiographs to Triage Patients with Acute Chest Pain Syndrome. Radiology. doi.org/10.1148/radiol.221926.