Stains are applied to the samples before pathologists examine them. However, the typical methods used in histopathology for staining tissue samples take a lot of time and call for specialized lab equipment, chemical reagents, and trained personnel.
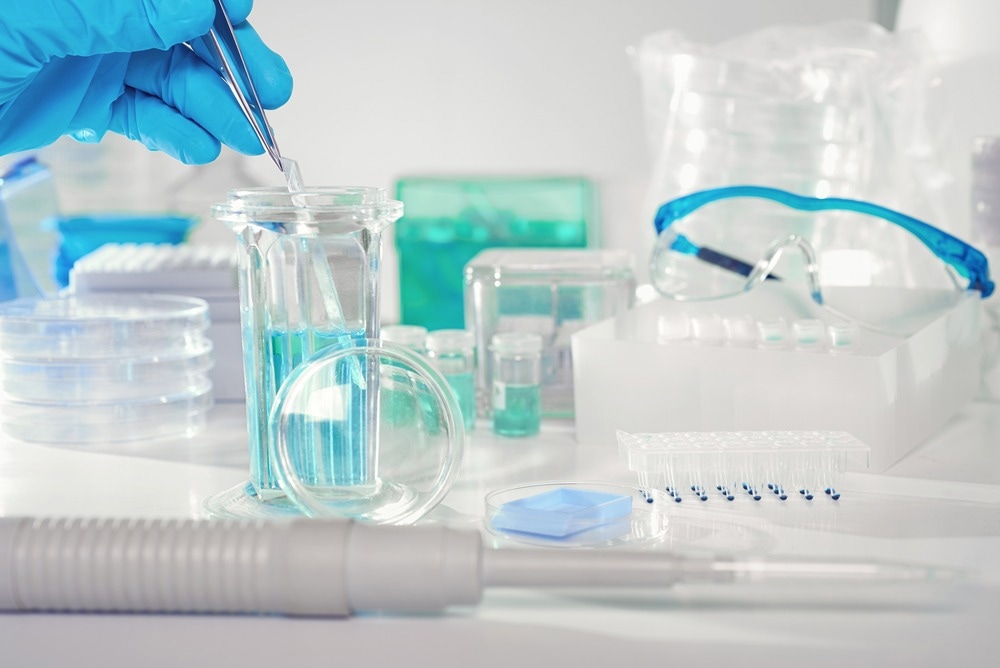
Image Credit: tilialucida/Shutterstock.com
Misdiagnosis could result from differences in tissue staining methods used by various laboratories and histology technicians. Additionally, since each stage of the current histochemical staining techniques has an irreversible effect on the sample, the original tissue sample is not maintained.
Researchers are utilizing AI methods to enhance pathology workflow as artificial intelligence (AI) continues to grow. In a recent study, UCLA researchers employed deep neural networks to virtually dye microscopic images of unlabeled tissue. The study was released on October 27th, 2022, in the journal Intelligent Computing.
Using deep neural networks instead of several time-consuming and tedious histochemical staining procedures, unlabeled tissue segment images have already been stained. However, there are some obstructions.
The study authors stated, “In all the label-free virtual staining methods, the acquisition of in-focus images of the unlabeled tissue sections is essential. In general, focusing is a critical but time-consuming step in scanning optical microscopy.”
The optimal focal plane is found using an iterative search technique, which is time-consuming and may cause photobleaching and photodamage to the samples. The most popular autofocusing approach requires several focus points across the tissue slide region with high focusing precision.
The authors describe a new deep learning-based quick virtual staining approach to address these issues.
“This framework uses an autofocusing neural network (termed Deep-R) to digitally refocus the defocused autofluorescence images. Then a virtual staining network is used to transform the refocused images into virtually stained images,” the authors added.
The new framework presented by the authors uses fewer focal points and lowers the focusing precision for each focus point in comparison to the conventional virtual staining framework to produce coarsely-focused whole slide autofluorescence images of tissue.
The time required for autofocusing and the entire image acquisition procedure can be significantly decreased by this innovative virtual staining framework.
The authors added, “The deep learning-based framework decreases the total image acquisition time needed for virtual staining of a label-free whole slide images (WSI) by ~32%, also resulting in a ~89% decrease in the autofocusing time per tissue slide.”
It is nevertheless possible to produce high-quality staining that nearly resembles the equivalent histochemically stained ground truth images, despite the loss of image sharpness and contrast compared to normal virtual staining frameworks. Additionally, the conventional virtual staining framework can be strengthened by using this framework as an add-on module.
Future expansion opportunities for this fast virtual staining system look promising.
“This fast virtual staining workflow can also be expanded to many other stains, such as Masson’s Trichrome stain, Jones’ silver stain, and immunohistochemical (IHC) stains. Although the virtual staining approach presented here was demonstrated based on the autofluorescence imaging of unlabeled tissue sections, it can also be used to speed up the virtual staining workflow of other label-free microscopy modalities,” added the authors.
Journal Reference
Zhang, Y., et al. (2022) Virtual Staining of Defocused Autofluorescence Images of Unlabeled Tissue Using Deep Neural Networks. Intelligent Computing. doi:10.34133/2022/9818965.