Reviewed by Mila PereraOct 28 2022
Plankton are central to marine ecology and essential for advanced marine ecological management. Since earlier studies have demonstrated that zooplankton are impervious to long wavelength (i.e., red) light, this light is frequently used to form images of plankton as part of the environmental management process.
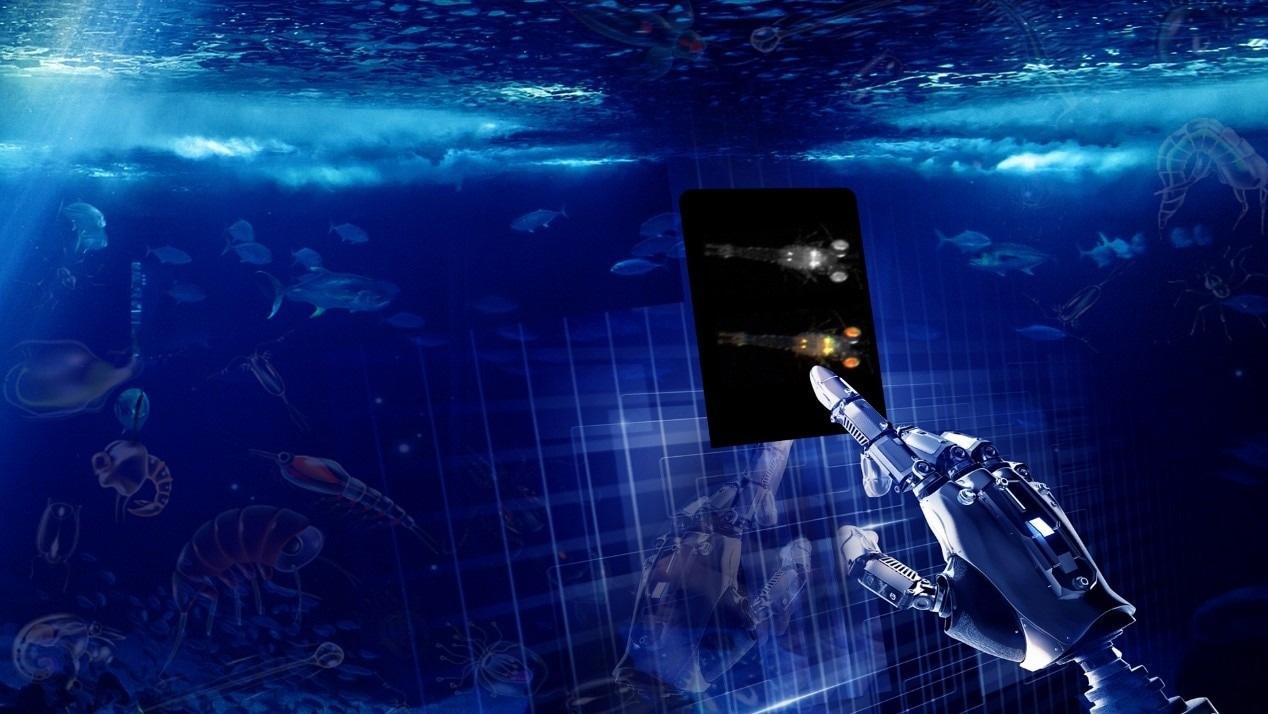
Teaching AI to accurately colorize marine plankton images. Image Credit: LI Jianping
However, red light only yields grayscale images of plankton, thereby losing information about their true color.
To solve this problem, a research group directed by Dr. Jianping Li from the Shenzhen Institute of Advanced Technology (SIAT) of the Chinese Academy of Sciences (CAS) recently formulated an automatic plankton colorization algorithm, the IsPlanktonCLR network, established on deep convolutional neural networks.
The algorithm is taught to change gray images obtained under red lighting into high-fidelity color images. It can automatically and straightforwardly “dye” marine plankton grayscale images captured underwater into their natural colors, and the colorization effect is extremely close to human eye sensitivity.
The research was presented at the European Conference on Computer Vision (ECCV), which was conducted in Israel between October 23rd and 27th, 2022. Scientists from Xiamen University and the Harbin Institute of Technology (Shenzhen) were also a part of this study.
The IsPlanktonCLR network uses a two-path structure with a self-guidance function, a tailored color palette, and a stepwise focusing loss function to accomplish the automatic colorization of plankton grayscale images. It demonstrates superior accuracy and fidelity in color restoration for unusual species and core parts of regular species.
To teach and produce the IsPlanktonCLR algorithm, the researchers put together a dataset comprising thousands of color-gray plankton image pairs. With this dataset, they not only taught the colorization algorithm but also equated its performance with advanced colorization algorithms.
The experimental outcomes demonstrated that the IsPlanktonCLR algorithm delivered excellent results not only concerning human visual perception but also for peak signal-to-noise ratio (PSNR), Fréchet inception distance (FID), structural similarity (SSIM), and other classical metrics commonly used in machine vision.
Furthermore, current colorization algorithms commonly lack quantitative and objective evaluation metrics. Currently, available evaluation metrics not only have trouble mimicking the color sensitivity ability of human vision but also have trouble objectively assessing the color similarity between the ground-truth image and the colored image.
Thus, the team suggested a color similarity assessment index, Color Dissimilarity (CDSIM), which integrates a color histogram, color correlogram, color aggregation vector, and color gradient.
Through the experiments on aquatic plankton and natural scene images, the team confirmed that CDSIM is good at assessing the effect of colorization algorithms but is also ideal for image colorization evaluation in the domain of scientific imaging than other prevailing metrics.
The development of IsPlanktonCLR provides a new artificial intelligence solution for ocean imaging instruments to obtain accurate and real observation results. This provides new technical means for us understanding the ocean.
Dr. Jianping Li, Study Lead Author, Shenzhen Institute of Advanced Technology, Chinese Academy of Sciences