Reviewed by Mila PereraOct 28 2022
Small leadless implanted electronic devices (LLIEDs) are currently considered a safer option than lead-based cardiac rhythm-management systems, with developments in battery technology, miniaturization, and communication.
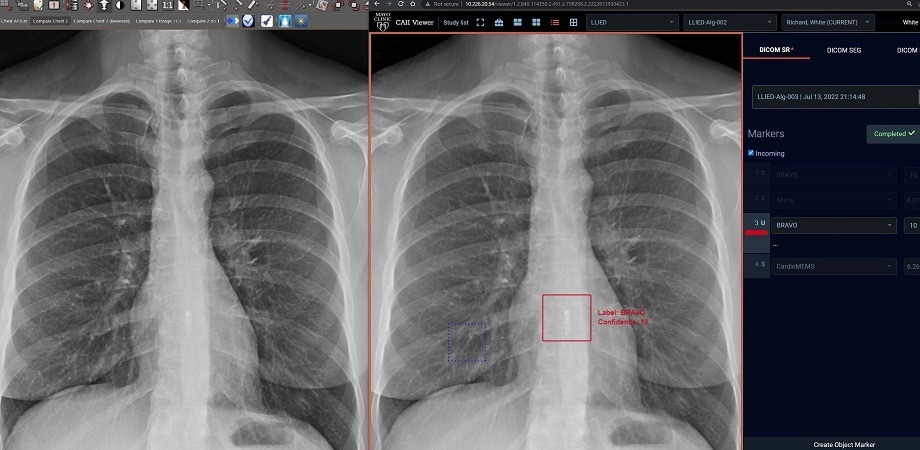
(Left) The standard clinical PACS (Picture archiving and communication system) frontal chest X-ray image, which does not detect/identify LLIED. (Right) ZF GUI/Viewer display of 12-class AI model inference result on the same monitor. The model immediately correctly detected and identified an MRI-unsafe LLIED from the same CXR. Radiologists can achieve positive adjudication with a single click, confirmed by the demarcation of the red boundary around the image. The AI model can, thus, deliver inference results to radiologists for adjudication before clinical use. Image Credit: R. White et al., doi 10.1117/1.JMI.9.5.054504
Intrathoracic LLIEDs not only support cardiac pacing but also facilitate the tracking of cardiovascular and electrophysiologic activity and non-cardiovascular physiology.
Nevertheless, detecting and identifying them (general category, location, specific type, etc.) is important, particularly before circumstances such as magnetic resonance imaging (MRI) scans comprising exposure to electromagnetic and radiofrequency.
In pre-MRI safety screening, existing techniques involving instant interaction between the physician and patient, chest X-Ray (CXR), and electronic medical records (EMR) offer minimal and insufficient data. Thus, they are inadequate for recognizing emerging, rarely used, and much smaller LLIEDs.
Furthermore, the problem is intensified by the small LLIED size, motion-related blurring, suboptimal screening method, and similarities in appearance, and LLIEDs can ultimately be ignored on a CXR during emergencies. Additionally, the inability to convey whether an LLIED is a recorder or a pacemaker can place the patient at substantial risk during an MRI scan.
LLIEDs span a spectrum of categories based on their MRI exposure safety, from being ‘MRI conditional’ to being ‘MRI unsafe.’ Our AI model for recognizing continuously evolving LLIEDs is based on LLIED classification obtained from the identification and labeling of regions of interest from retrospective and/or future organization-wide CXR data.
Richard D. White, Radiologist, Mayo Clinic
Even though both are said to be “MRI conditional,” the pacemaker requires a cardiology device and patient omission before, after, and even during, the MRI examination.
Responding to the requirement for rapid and correct detection of LLIEDs during MRI pre-screening, an artificial intelligence (AI)-based model was created by scientists guided by Richard D. White, a renowned radiologist at Mayo Clinic Florida, USA.
In their latest study reported in the SPIE Journal of Medical Imaging, White’s team evaluated this model's promptness and operational prerequisites with the goal of advancing toward practical applications.
For the pre-deployment evaluation, the researchers used a two-tier cascading methodology containing LLIED detection as tier 1, followed by classification as tier 2. They conducted a five-fold cross-validation during tier 1 to evaluate the sturdiness of the “Original LLIED Model” originally including nine LLIED categories.
To reproduce real-world testing, they additionally applied the two-tier cascading AI model on 150 new CXR images from arbitrarily selected newer patients, already revealing three new LLIED categories.
Furthermore, the researchers integrated some vital technical developments to enable the real-world utilization of their AI model. These included a DICOM-Structured Report (DICOM-SR) to enable end-user inference-result adjudication, a Zero-Footprint (ZF GUI/Viewer) viewing system for imaging, and, most significantly, continuous learning with three new LLIED types to form a 12-class “Updated LLIED Model.”
The researchers then used new extra cases to test this model using the two-tier approach. The tier 1 study produced 100% detection/location sensitivity of LLIEDs for the 9-class as well as the 12-class models, and its durability was additionally verified by the five-fold cross-validation. In tier 2, both models accomplished excellent accuracy in recognizing the type of LLIED (MRI safety category and specific type).
Although no LLIEDs remained undetected in tier 1, there were a few cases of misidentification happening in tier 2 that were attributed to substandard image quality. The AI model did not misidentify any of the “MRI unsafe” or “MRI stringently conditional” LLIEDs.
While the actual value of the AI model can only be assessed in a true real-world clinical setting, these results harbor optimism in favor of deploying the AI model in the near future for assisting pre-screening evaluation by radiologists for patient safety.
Richard D. White, Radiologist, Mayo Clinic
The researchers integrated continuous learning, retraining, and upgraded the AI models based on end-user experience, concentrating on imitating real-world circumstances for testing their model. This is the first study of its kind to report AI-based radiographic detection and identification of LLIEDs.
White and his team hope to make the most of these outcomes and introduce the AI model in an applicable clinical setting. They also anticipate resolving the restrictions of this study by retraining and tweaking the AI model in the near future.
Journal Reference
White, R. D., et al. (2022) Pre-deployment assessment of an AI model to assist radiologists in chest X-Ray detection and identification of lead-less implanted electronic devices for pre-MRI safety screening: realized implementation needs and proposed operational solutions. Journal of Medical Imaging. doi.org/10.1117/1.JMI.9.5.054504.