Feb 9 2021
At Geisinger, scientists have discovered that a computer algorithm trained with echocardiogram videos of the heart has the ability to predict mortality within a year.
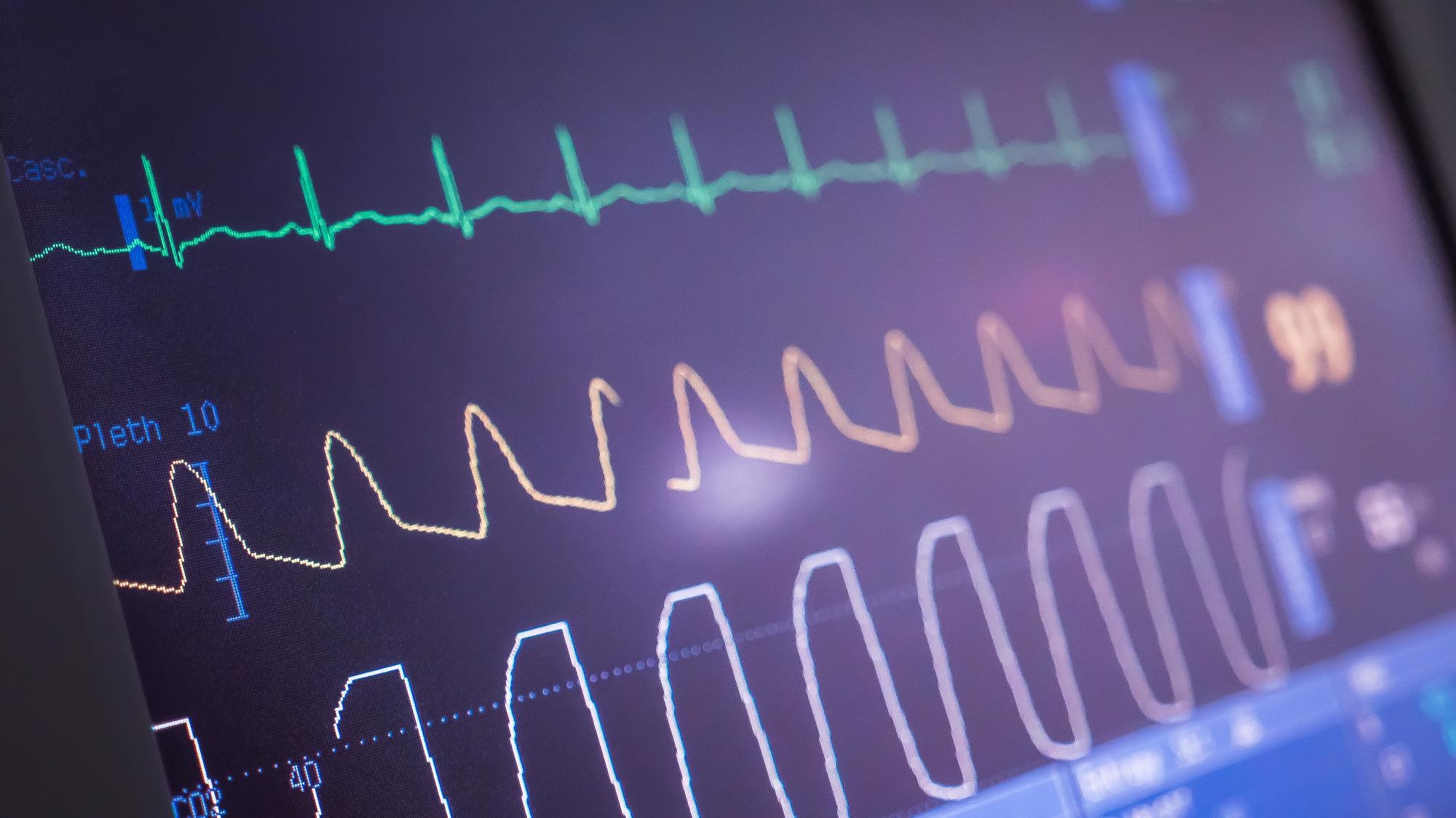
Image Credit: Medijucentras/Shutterstock
The algorithm is an example of the so-called machine learning, or artificial intelligence (AI), and was found to be better than other clinically used predictors, such as the Seattle Heart Failure score and pooled cohort equations. The study findings have been reported in Nature Biomedical Engineering.
We were excited to find that machine learning can leverage unstructured datasets such as medical images and videos to improve on a wide range of clinical prediction models.
Chris Haggerty, PhD, Study Co-Senior Author and Assistant Professor, Department of Translational Data Science and Informatics, Geisinger
Imaging plays a vital role in treatment decisions in a majority of the medical specialties. It has turned out as one of the most data-rich components of the electronic health record (EHR).
For instance, a single ultrasound of the heart returns roughly 3,000 images, and cardiologists have very little time to comprehend these images within the context of numerous other diagnostic data.
This offers considerable opportunity to exploit technology, like machine learning, to handle and analyze this data and eventually offer smart computer assistance to physicians.
As part of the study, the researchers used exclusive computational hardware to train the machine learning model on 812,278 echocardiogram videos gathered from 34,362 Geisinger patients in the past 10 years.
The researchers compared the results of the model with the predictions of cardiologists based on numerous surveys. Another survey demonstrated that when cardiologists are assisted by the model, the accuracy of their prediction increased by 13%.
The study used almost 50 million images, representing one of the most extensive medical image datasets to be ever published.
Our goal is to develop computer algorithms to improve patient care. In this case, we’re excited that our algorithm was able to help cardiologists improve their predictions about patients, since decisions about treatment and interventions are based on these types of clinical predictions.
Alvaro Ulloa Cerna, PhD, Study Author and Senior Data Scientist, Department of Translational Data Science and Informatics, Geisinger
The study was partially supported by funding from the Pennsylvania Department of Health and the Geisinger Health Plan and Clinic.
Journal Reference:
Ulloa Cerna, A. E., et al. (2020) Deep-learning-assisted analysis of echocardiographic videos improves predictions of all-cause mortality. Nature Biomedical Engineering. doi.org/10.1038/s41551-020-00667-9.