Dec 11 2020
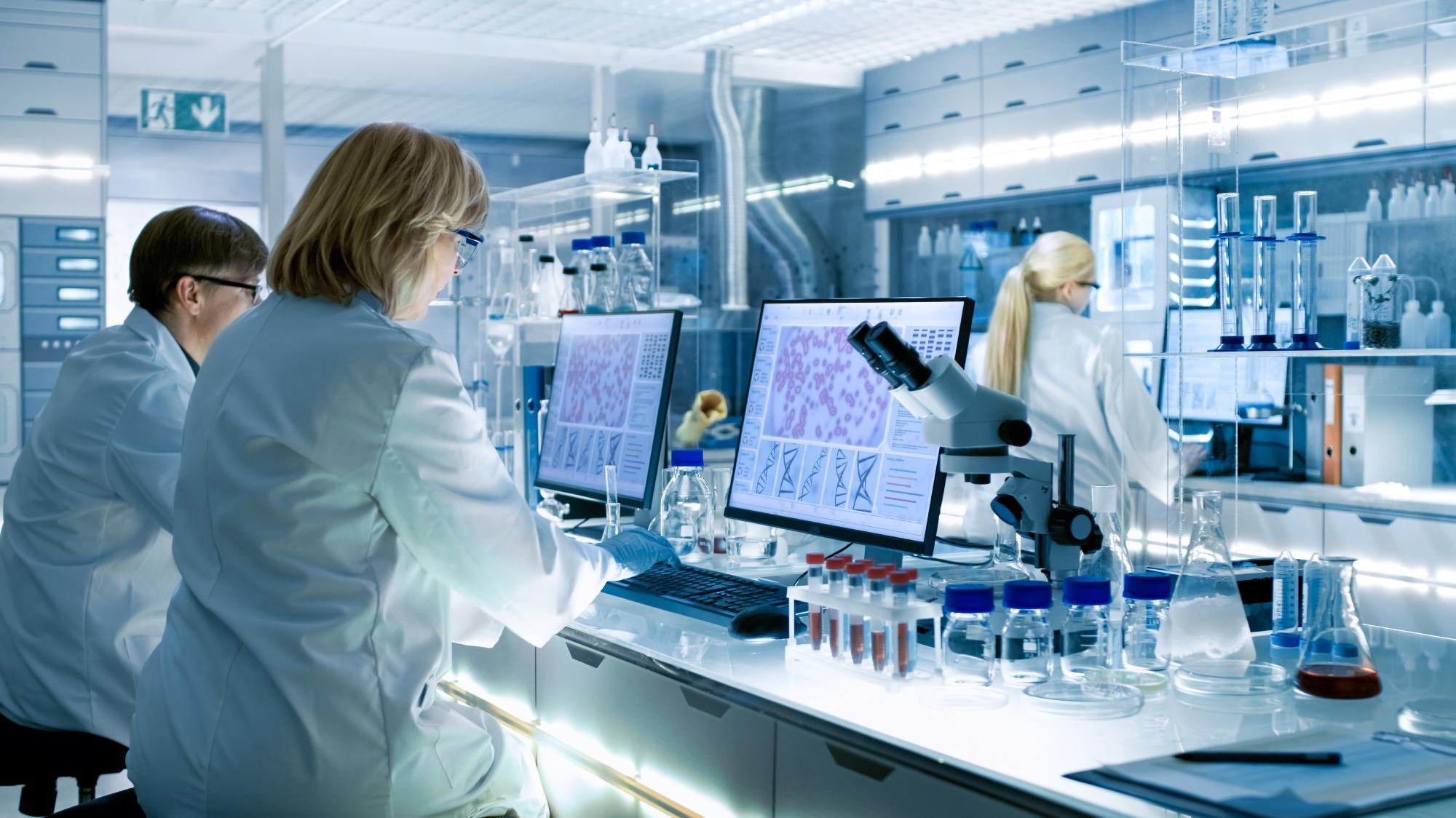
Image Credit: Gorodenkoff/shutterstock
Artificial intelligence could soon be added to the testing toolkits of hospital-based laboratories and doctors at the forefront of the COVID-19 pandemic.
Recent research by collaborators from the University of Vermont and Cedars-Sinai explains the performance of Biocogniv’s new AI-COVID™ software. The researchers discovered high precision in estimating the probability of COVID-19 infection during routine blood tests, which could enable hospitals to reduce the number of patients referred for scarce PCR testing.
Nine months into this pandemic, we now have a better understanding of how to care for patients with COVID-19, but there’s still a big bottleneck in COVID-19 diagnosis with PCR testing.
Timothy Plante, MD, MHS, Study Lead Author and Assistant Professor, University of Vermont
PCR testing is the existing standard diagnostic for COVID-19 and necessitates specific sampling, such as a nasal swab, and exclusive laboratory equipment to perform.
“According to data from over 100 US hospitals, the national average turnaround time for COVID-19 tests ordered in emergency rooms is above 24 hours, far from the targeted one-hour turnaround,” noted Tanya Kanigan, Ph.D., Chief Operating Officer, Biocogniv.
Emergency departments order Complete Blood Count and Complete Metabolic Panels, which are are common laboratory tests and have a fast turnaround time. These tests offer an understanding of the immune system, liver, kidney and electrolytes. The researchers could train a model that investigates variations in these routine tests and assigns a possibility of the patient being COVID-19 negative with high precision.
“AI-COVID takes seconds to generate its informative result once these blood tests return, which can then be incorporated by the laboratory into its own test interpretation,” stated Jennifer Joe, MD, an emergency physician in Boston, Massachusetts, and Biocogniv’s Chief Medical Officer. “In an efficient emergency department that prioritizes these routine blood tests, the door-to-result time could be under an hour.”
According to Victor Tapson, MD, a Cedars-Sinai pulmonary and internal medicine specialist, such assistive tools that enable physicians to rule out probable diagnoses are well known in emergency medicine.
For example, a low D-dimer blood test can help us rule out clots in certain patients, allowing providers to skip expensive, often time-consuming diagnostics such as chest CT scans.
Victor Tapson, Cedars-Sinai Pulmonary and Internal Medicine Specialist
The Biocogniv researchers believe that a viable additional benefit of laboratories adding AI-COVID might be the reduction in time taken for conventional PCR results.
“With the help of AI-COVID, laboratories might relieve some of the testing bottleneck by helping providers better allocate rapid PCR testing for patients who really need it,” added Joe.
The team validated the AI-COVID model using real-world data obtained from Cedars-Sinai and data from demographically and geographically diverse patient encounters from 22 U.S. hospitals, thus realizing an area under the curve (or AUC) of 0.91 out of 1.00.
This enables the model to achieve a high sensitivity of 95% while maintaining moderate specificity of 49%, which is very similar to the performance of other commonly used rule-out tests.
George Hauser, MD, Pathologist and Chief Scientific Officer, Biocogniv
“I’m honored to have such an impressive team of medical scientists from the University of Vermont and Cedars-Sinai as collaborators in validating this timely model. AI has progressed considerably; the time is now to leverage this powerful tool for new healthcare breakthroughs, and we’re glad to direct it to help hospital laboratories and providers combat the current COVID-19 crisis,” concluded Artur Adib, Ph.D., CEO of Biocogniv.
Journal Reference:
Plante, T. B., et al. (2020) Development and External Validation of a Machine Learning Tool to Rule Out COVID-19 Among Adults in the Emergency Department Using Routine Blood Tests: A Large, Multicenter, Real-World Study. Journal of Medical Internet Research. doi.org/10.2196/24048.