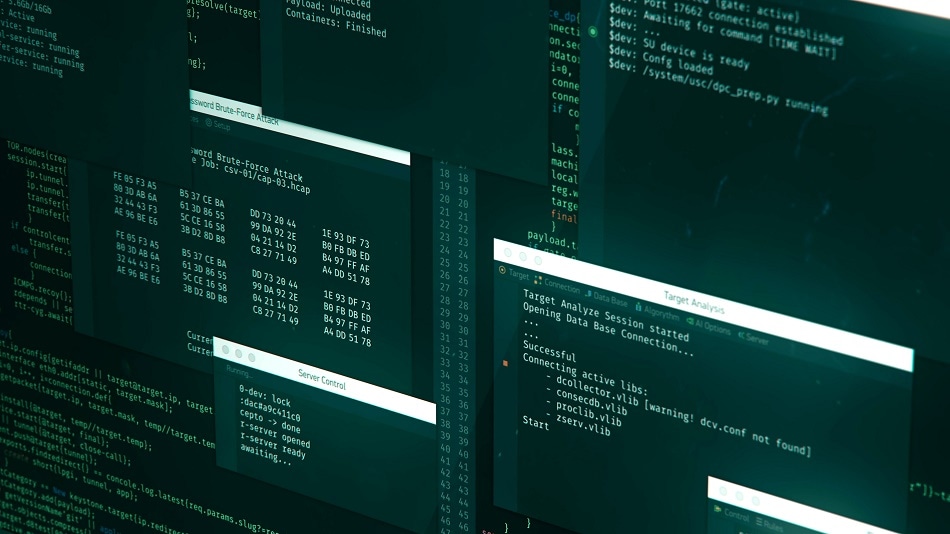
Image credit: SynthEx / Shutterstock.com
Researchers at MIT and the University of California at San Diego (UCSD) have developed a new machine-learning system finds “serial hijackers” of internet IP addresses.
IP Address Hijacking Has Been A Problem Since The 90s
Back in the late 90s the US Senate was warned about the threat to security that hijacking IP addresses posed to the country. A group of hackers warned that it was possible for them to take down the internet through this kind of cyber-hacking. Today, IP address hijacking remains a concern, with hackers administering spam, malware and even conducting Bitcoin theft through this method.
How IP Hijacking Threatens Security
IP hijacking is an efficient way for hackers to gain access to traffic from public internet or on private business networks. Hackers corrupt routing tables of the Border Gateway Protocol (BGP), where routing information is exchanged so that it can reach its intended destination.
Hijackers convince nearby networks to go through their network in order to reach a specific IP address, a process which is surprisingly easy to achieve, and in doing this, hackers gain control of groups of IP addresses. Once traffic has been redirected to the hackers’ own networks, they have the capability to not only intercept and view data, but to even modify it.
As a result, hackers have a means to send spam and malware and even steal Bitcoin. For this reason, combating hacker’s ability to hijack IP addresses has remained a focus for researchers aiming to improve internet security, and this month, a breakthrough has been made at MIT that could potentially detect these kind of cybercriminals, stopping them before they’ve committed these attacks.
Developments From MIT Using Machine Learning
Current methods to detect IP hijacks track hackers once they are already in the process of corrupting the BGP. What the team at MIT has achieved is developing a method that can prevent these attacks from even beginning in the first place through predicting these incidents by identifying the hackers.
MIT researchers have used machine learning to develop a new system which can recognize traits shared by ‘serial hijackers’, hackers who frequently hijack IP addresses. They demonstrated that the system is successful at identifying hacker networks, in testing the system they were able to identify 800 suspicious networks, and in analyzing them they found that some of them had even been active for years.
This development has the potential to significantly change the face of cyber security. In the past network operators have only had the opportunity to address these kind of cybercriminals in a reactive way, which hasn’t been efficient at stopping hijacks from occurring. Now operators have the opportunity to proactively defend their networks.
Future Directions
While the model offers a promising way to enhance cyber security, experts have stated that work still needs to be done to develop it further. Currently, human supervision is required in order for the system to work effectively. There is still the possibility for false positives to be churned out by the system, which may falsely identify safe behaviors as those appearing to signify cyber attacks.
For this reason humans are still required to clarify whether or not identifications of suspicious activity are authentic or not. Given that around 20% of identifications made by the system were false positives, there is still some work to be done to improve the system’s specificity and success at identifying authentic attack situations. Researchers are hopeful that they will be able to achieve this, and therefore minimize the requirement for human intervention.
One way the system is already causing a shift in the way cyber attacks are prevented is that it gives network operators the chance to move away from investigating specific attacks, allowing them to investigate global Internet routing across years, helping them to identify ‘serial hijackers’.
Disclaimer: The views expressed here are those of the author expressed in their private capacity and do not necessarily represent the views of AZoM.com Limited T/A AZoNetwork the owner and operator of this website. This disclaimer forms part of the Terms and conditions of use of this website.