Intellegens' machine learning software Alchemite™ is helping to catalyze innovation within key sectors within the chemical, manufacturing and pharmaceutical industries. Following ChemUK 2022, we explore Intellegens' solutions in more detail, and the benefits machine learning can bring to research productivity.
Please could you introduce yourself and tell us about your role within Intellegens?
My name is Dr. Gareth Conduit and I am Co-founder and Chief Scientific Officer at Intellegens, driving the scientific program and leading the development of our machine learning solution.
How did you initially become involved with machine learning technologies, particularly their application to the chemical industry?
The Intellegens software, Alchemite™, originated with methods developed in my research group at the University of Cambridge. We were researching how to extract maximum value from experimental and process data in the design of materials. This led us to develop a novel deep learning methodology that could handle the sparse data by identification and exploitation of property-property correlations.
We first applied the methodology to design alloys, which were later experimentally validated with collaborators Rolls-Royce. Alchemite™ was commercialized with the spin-out of Intellegens, allowing us to work with many other organizations that face similar challenges in designing or optimizing chemicals, formulations, materials, or manufacturing processes.
Who are Intellegens, and what solutions does it provide?
We are a software company based in Cambridge, UK. Our novel machine learning software product, Alchemite™, can extract value from sparse and noisy experimental data where other machine learning methods fail.
Alchemite™ is applied to accelerate innovation in materials, chemicals, manufacturing, and beyond. One key benefit is reducing the amount of experimentation required to achieve an objective, saving time and cost. Another is finding product and process improvements that would otherwise be missed.
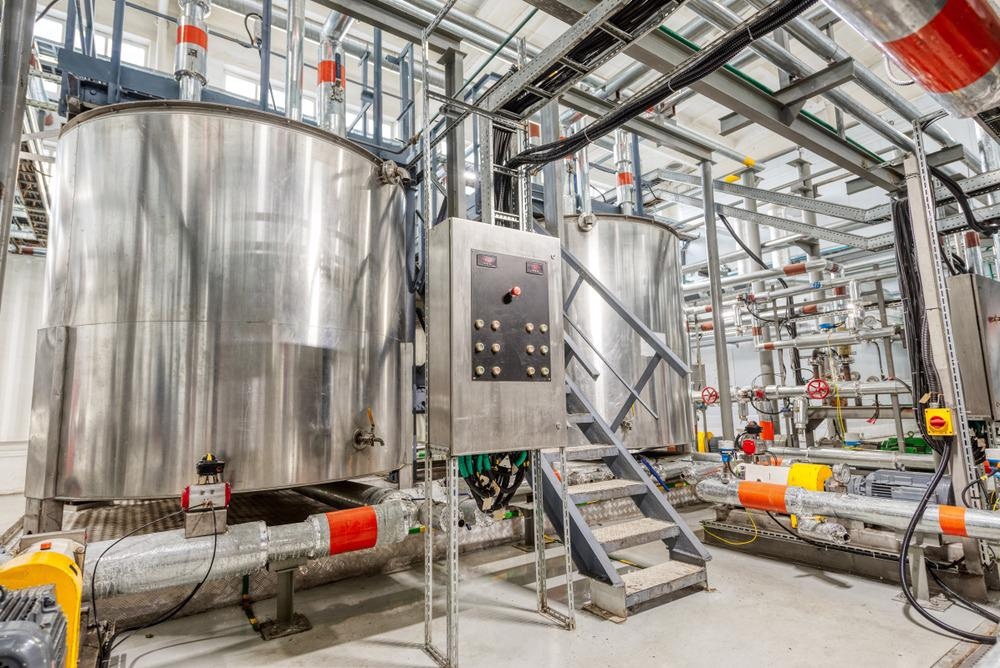
© Nordroden/Shutterstock.com
Could you walk us through an example chemical R&D workflow and where Intellegens’ machine learning software Alchemite could be applied?
A typical example is what we call Adaptive Design of Experiments, which is used by an organization undertaking a large number of experiments. For example, this could involve trying to understand the factors (ingredients and process steps) influencing the properties of a chemical formulation and, ultimately, to identify formulations with better properties.
A brute force search of formulation space by making every conceivable formulation is usually impossible. Instead, many organizations use statistical methods to narrow down their search. But such conventional ‘Design of Experiments’ (DoE) still requires many experiments with associated high cost and lead-time.
Alchemite™ machine learning uncovers hidden relationships in the data to understand where it would be most productive to gather data to generate a more accurate model. Once performed, the results of these experiments are fed back into the system, making its predictions progressively more accurate. This adaptive approach typically results in projects that achieve their goals with 50-80% fewer experiments.
You recently presented at CHEMUK, discussing the benefits machine learning can bring to chemical processes and formulations. Why should not just companies, but also expert representatives, work to disseminate information at such events? What are the benefits of doing so?
We’re excited by the potential of our work. But, to realize that potential, it really needs to be applied in practice in industrial R&D. We now have a growing group of customers and industrial success stories. However, there are many industrial organizations that aren’t taking advantage of machine learning yet – or, perhaps, that have tried other methods that don’t work so well with real-world industrial data.
Making sure that methods like ours fully meet their potential requires sharing of knowledge and partnerships between academic research, technology providers such as Intellegens, and industrial R&D. Events like CHEMUK are great venues to share this knowledge and make the necessary connections.
You are at the forefront of new machine learning strategies, leading the development of software solutions such as Alchemite™. How did you approach this project in the beginning? Were there any logistical or computational challenges that had to be overcome?
Yes, many! We started out working on customer case studies to understand the problem and opportunity of materials better. On this journey, some of the computational challenges included: developing an algorithm that could handle sparsity and noise in the data in a general way; ensuring accurate quantification of uncertainty in our predictions (because this is important in ensuring the results can be used to steer development decisions); making the methods computationally-efficient so that they run quickly.
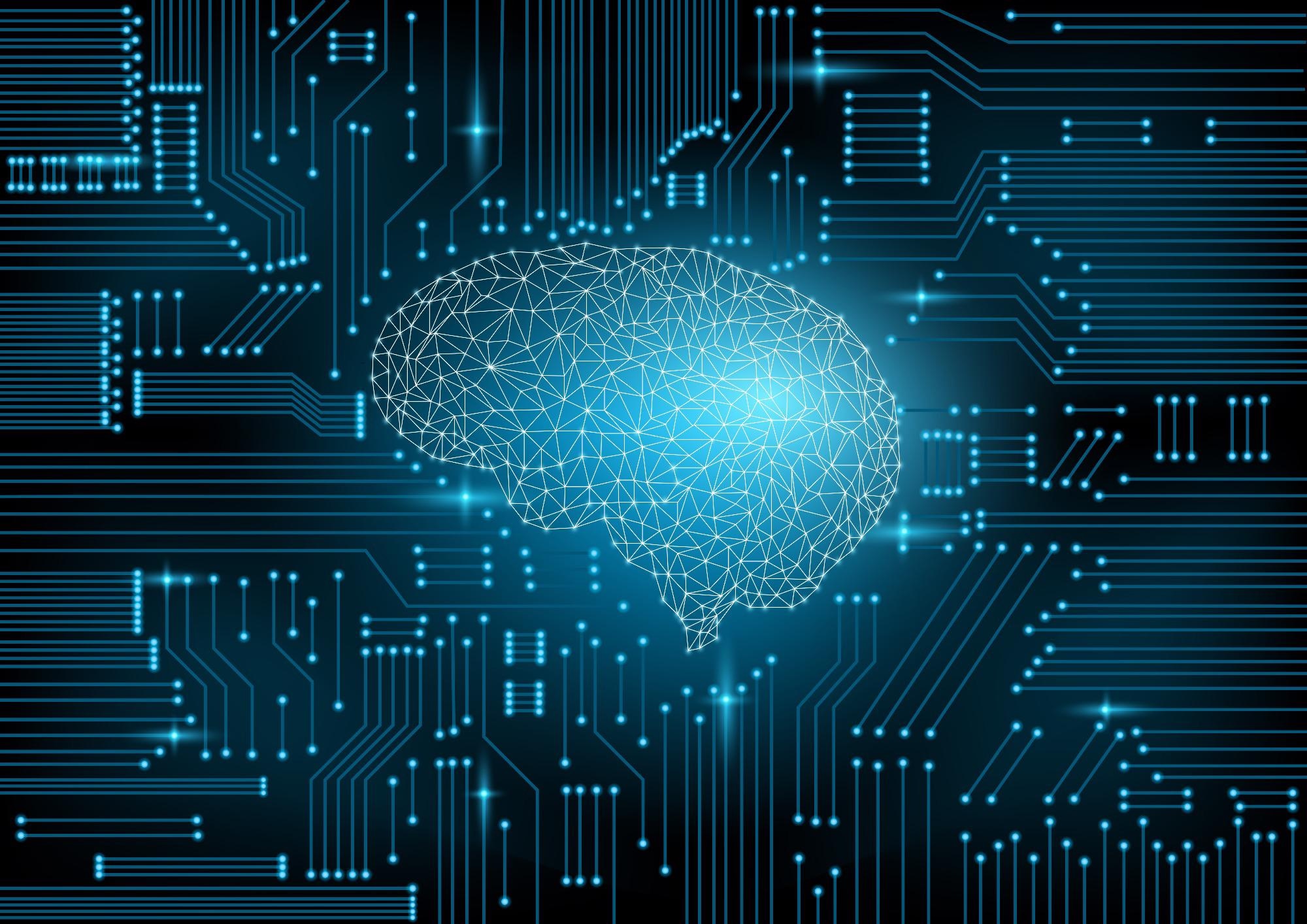
© CNStock/Shutterstock.com
We have seen how machine learning and artificial intelligence can boost the productivity of research, though their everyday use in industry feels relatively new. In your opinion, what are the main barriers that limit the real-world application of this technology?
Firstly, one major barrier is ensuring the methods work well on real-world datasets without requiring ‘massaging’ or pre-processing of the data. Secondly, delivering access to the algorithms within an intuitive user interface that can be used by engineers who are experts in the application domain (in our case, materials or chemistry), but not in data science.
How do you expect machine learning technologies to influence the advancement of the chemical production sector in the years to come? Are there any areas, in particular, that would benefit from its integration?
Key impacts can come from improvement in understanding and setting of chemical plant production processing variables that are difficult to otherwise model. This enables better performance and finding efficiencies that can reduce environmental impact and time in development and experimentation, thus freeing up resources and getting products to market faster.
Throughout your experience, what has been the most memorable project you have been involved with?
Memorable projects are those with real-world positive commercial impact. I, therefore, really enjoyed the early collaborations with Rolls Royce, where we first identified the opportunity to exploit sparse data and proceeded to design and experimentally verify alloys. More recently, I was excited by a collaboration with International Flavors & Fragrances, where we used machine learning to replace subjective human survey results for the attractiveness of perfumes.
About Dr. Gareth Conduit
Dr. Gareth Conduit focuses on applying machine learning to solve real-world problems, with examples spanning materials science to drug discovery. He is a Royal Society University Research Fellow at the University of Cambridge.
At Intellegens, as CSO, Dr. Conduit lead the development of the Alchemite™ software and of new machine learning products to complement Intellegens’ offering.
Disclaimer: The views expressed here are those of the interviewee and do not necessarily represent the views of AZoM.com Limited (T/A) AZoNetwork, the owner and operator of this website. This disclaimer forms part of the Terms and Conditions of use of this website.