AZoRobotics speaks with Professor Naeem Ramzan from the University of the West of Scotland about his development of an innovative artificial intelligence (AI) technology that can accurately identify COVID-19 in just a matter of minutes. This is potentially game-changing research that could make a real difference in the fight against COVID-19.
As infections continue to rise, why is it so important to develop tools that are capable of rapidly detecting COVID-19?
As of the first quarter of 2022, the COVID-19 pandemic kept on affecting the well-being and health of the public. A critical step in the battle against COVID-19 is a reliable and effective detection method for diagnosing infected patients, with the end goal of prompt treatment and care.
The main screening technique used for identifying COVID-19 cases is reverse transcription polymerase chain reaction testing (RT-PCR), which can recognize COVID-19 from respiratory samples gathered through various means, e.g., nasopharyngeal or oropharyngeal swabs.
There was a need to identify a quick and reliable tool that could detect COVID-19 effectively with minimal effort so that treatment could start immediately, something that could prove to be crucial and potentially life-saving when diagnosing severe cases of the virus. Such a tool could also help to relieve strain on hard-pressed Accident and Emergency departments, particularly in countries where PCR tests are not readily available.
Can you provide an overview of how this novel process works and how AI aids in COVID-19 diagnoses?
Radiography assessment is a screening method that can be used as an alternative for COVID-19 detection, where chest radiography imaging, such as computed tomography (CT) or chest X-Ray (CXR), is conducted and analyzed by radiologists to identify visual markers related to COVID-19 viral infection.
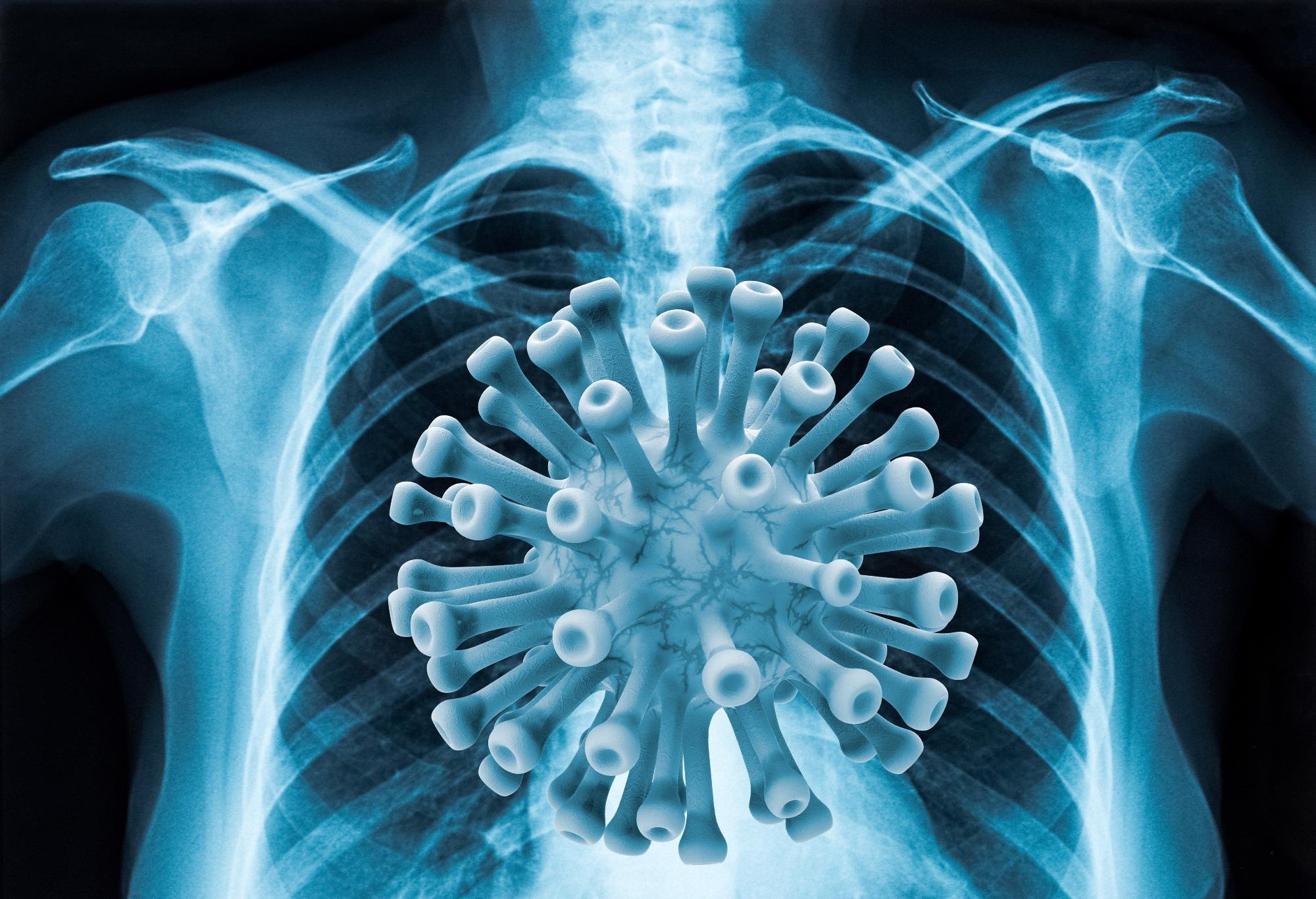
Image Credit: Shutterstock.com/ AGCuesta
Early investigations showed that patients tend to present abnormalities in chest radiography images if they are positive for COVID-19, with some suggesting that radiography assessment could be utilized as a primary tool for diagnosing COVID-19, especially for severe patients.
However, the greatest bottleneck is the requirement for expert radiologists to decipher the radiography images since the visual pointers can be unobtrusive. That is why we opted for a computer diagnostic framework that can help radiologists precisely decipher radiography images to recognize COVID-19 cases very quickly. It is of critical importance in the fight against the virus.
The critical need to develop solutions to help curb the challenges in the fight against COVID-19, motivated by the availability of chest X-Ray images of COVID-19 cases, led this study to develop Artificial Intelligence (AI) based algorithms, which can effectively detect COVID-19 with the highest accuracy.
The developed AI program is based on a deep convolutional neural network (CNN), an algorithm typically used to analyze visual imagery to make a diagnosis. The state-of-the-art technique utilized X-Ray technology, comparing scans to a database of around 3000 images belonging to patients with COVID-19, healthy individuals and people with viral pneumonia.
Three different versions of the examined CNN architectures were evaluated: (1) a baseline version where the pre-trained CNN models were used as is, changing only the classifier in their output to suit the task at hand, (2) a modified version with two additional fully connected layers between the convolutional base and the classifier, and dropout layers before each fully connected layer, and (3) a modified version with two larger, fully connected layers between the convolutional base and the classifier, and batch normalization and Leaky ReLU layers before each fully connected layer.
How effective is this technology in accurately identifying patients with COVID-19?
The developed solution is very effective. Firstly, it provides very high accuracy, almost 98%, and secondly, it can identify COVID in real-time, so results can be obtained within minutes as compared to PCR which takes more than two hours.
How did you assess the efficacy of your technology, and what were the results?
All the examined approaches were trained and evaluated on a dataset with real chest X-Ray images using a stratified 5-fold cross-validation procedure, while the best performing model was also evaluated on a completely unseen dataset.
Supervised classification experiments demonstrated the efficiency of the proposed approach, reaching a classification accuracy of 98.04% with an F1 score of 98.22%.
Did you come across any challenges during your research, and if so, how did you overcome them?
We came across a few challenges when trying to develop this real-time COVID detection application.
Getting access to COVID positive X-Rays was a challenge as it was difficult to get data due to GDPR issues as we needed quite a large dataset to train our AI algorithms. The real-time implementation was also a challenge as we needed to develop a lightweight application that required fewer resources but still gave highly accurate results.
How does the revolutionary artificial intelligence (AI) program you have developed compare to current PCR tests?
This real-time application was developed to detect COVID-19 by exploiting the AI algorithms. It is lightweight and can be installed on normal PCs or laptops.
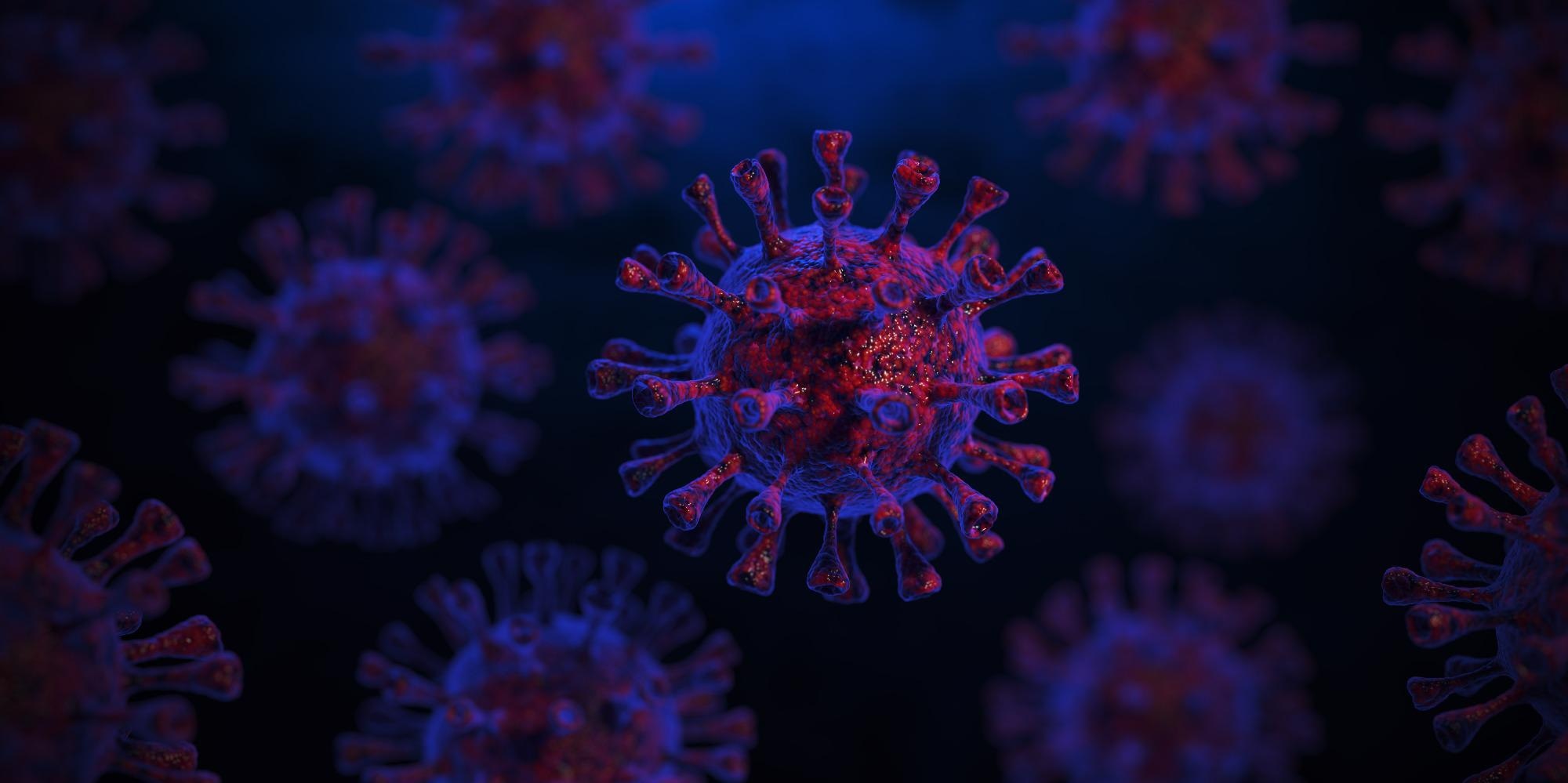
Image Credit: Shutterstock.com/ SWKStock
The application has been designed in such a way that no additional equipment is needed. Patient X-Rays simply need to be uploaded to the application via USB or web services, and the deep convolutional neural network algorithm analyses the image and gives back an output result indicating if it is COVID-19 positive or not.
The developed program has higher accuracy than PCR and produces results within a minute, whereas PCR takes a couple of hours.
How will the rapid detection of COVID-19 aided by this technology benefit the medical sector, specifically emergency departments?
The required medical and clinical resources for COVID-19 diagnosis on a global scale are a major challenge. Several countries are unable to carry out large numbers of COVID-19 tests, especially in remote locations, because of limited or no diagnosis tools available.
In addition, in many cases, there is a need to detect the virus far more quickly than a PCR test, which typically takes around 2-hours. However, our designed method can detect COVID in just 10-15 seconds, or in just under a minute for the most tricky cases.
Aside from COVID diagnoses, can this technology be used for anything else, i.e., helping to determine what treatment may be needed?
Apart from being used to detect COVID-19, this technology can also help to detect viral pneumonia. It can also be used to detect other lungs diseases like cancerous cells, but we need to retrain our model to add other modalities
What impact will such a novel technology have on the medical sector and society as a whole?
Several countries are unable to carry out large numbers of COVID tests because of limited diagnosis tools, but this technique utilizes easily accessible technology to quickly detect the virus.
COVID-19 symptoms are not visible in X-Rays during the early stages of infection, so it is important to note that the technology cannot fully replace PCR tests. However, it can still play an important role in curtailing the virus’s spread, especially when PCR tests are not readily available.
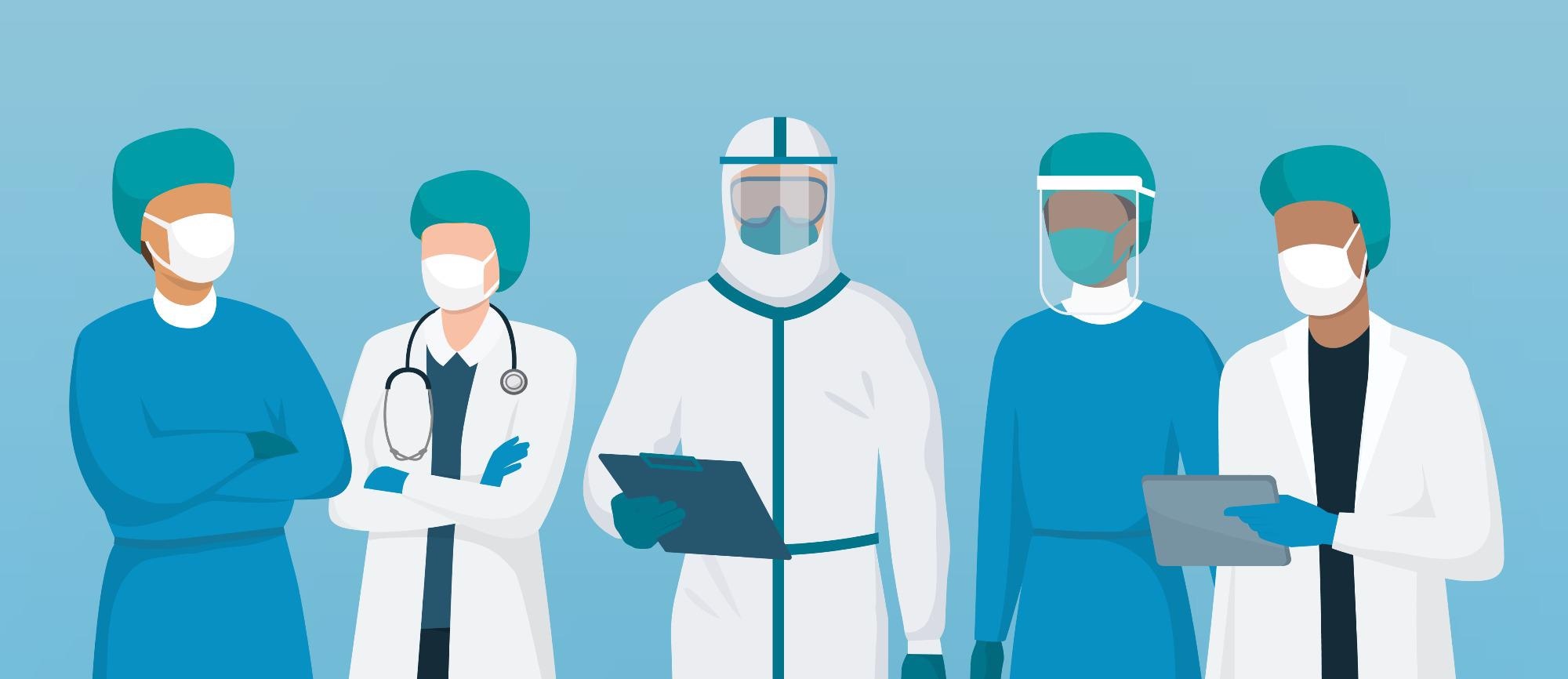
Image Credit: Shutterstock.com/ elenabsl
It could prove to be crucial and potentially life-saving when diagnosing severe cases of the virus, helping determine what treatment may be required.
What are the next steps for your research?
First, we plan to expand the study, integrating a bigger database of X-Ray images obtained by various models of X-Ray machines. This will enable us to assess the eligibility of the method in a clinical setting.
We are also planning to test this technology in Pakistan as part of an EU-funded SAFE RH project to see what impact it can have in a real-world setting.
About Professor Naeem Ramzan
Professor Naeem Ramzan (S’04, M’08, SM’13) is a professor and Director of the Affective and Human Computing for Smart Environment (AHCSE) Research Centre at the University of the West of Scotland (UWS), UK. He received a M.Sc. degree in telecommunication from the University of Brest, France, in 2004 and a Ph.D. degree in electronics engineering from Queen Mary University of London, London, U.K., in 2008. He was a senior research fellow and lecturer at Queen Mary University of London from 2008 to 2012.
His research interests are cross-disciplinary & industry-focused and include AI/machine learning, Affective Computing and Multimedia Processing, analysis and communication, video quality evaluation, Brain-inspired multi-modal cognitive technology, Big Data analytics, Affective computing, IoT/smart environments, Natural multi-modal human computer interaction, eHealth/connected Health.
Apart from the research work at UWS, he led a team of young and enthusiastic lecturers to develop a highly innovative portfolio of post-graduate studies, including MSc Advanced Computing, MSc Big Data, MSc IoT, and MSc eHealth.
Disclaimer: The views expressed here are those of the interviewee and do not necessarily represent the views of AZoM.com Limited (T/A) AZoNetwork, the owner and operator of this website. This disclaimer forms part of the Terms and Conditions of use of this website.