Food safety is critical to our development and wellbeing. Because of the diversity and intricacy of today's food production systems, the highest food safety regulations must be enforced through cutting-edge techniques to avoid health risks for individuals, costly recalls and potential reputational harm for food production companies.
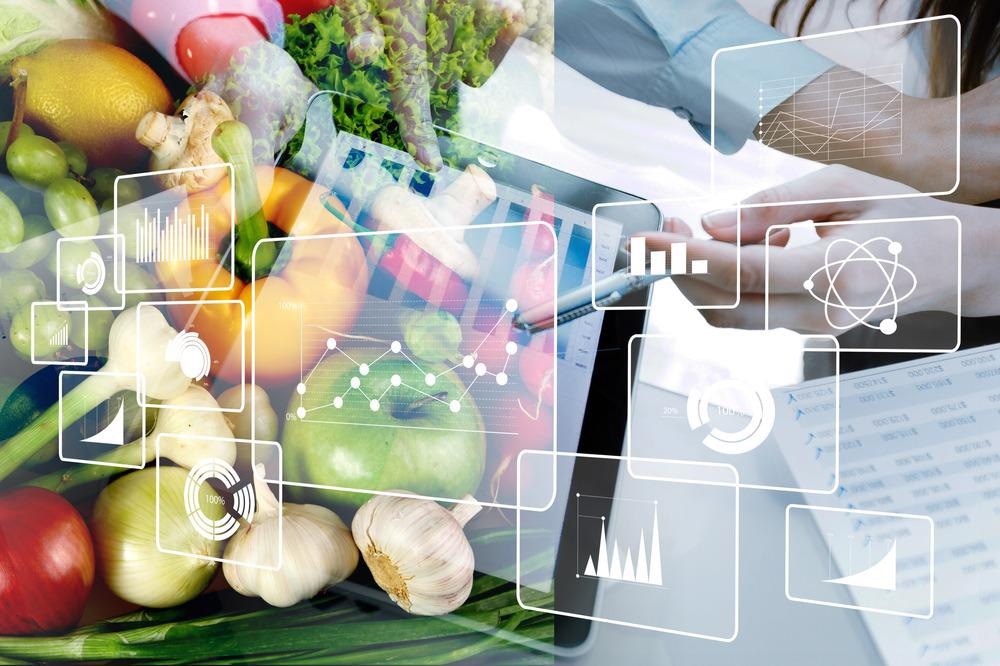
Image Credit: Billion Photos/Shutterstock.com
Artificial intelligence (AI) and machine learning (ML)-based technologies have recently gained a lot of interest in developing new food safety techniques.
Significance of Food Safety
Access to an adequate supply of nutritious food is critical for maintaining a good quality lifestyle. As a result, it is unsurprising that food safety is a top issue in the public health industry. This becomes more obvious when considering WHO statistics, which predict that 600 million people (almost 1 in 10) get severely ill each year as a consequence of consuming tainted food, resulting in around 420,000 fatalities.
Apart from the direct human cost, insufficient food safety has a broader ripple impact, impeding socio-economic progress, particularly in developing countries. Inadequate access to clean food perpetuates a destructive cycle of sickness and hunger, putting a strain on healthcare systems, hindering social and economic growth, and lowering the overall quality of life. As a result, it is critical to design next-generation food safety technologies that reduce food safety hazards. This is where artificial intelligence and machine learning technologies can be especially beneficial.
Data Analytics in Food Safety
The rising digitalization of business and society creates new possibilities for monitoring, analyzing, and improving health care standards. In the food industry, the use of data analysis technology is enabling the creation of a transparent, safer food supply chain from production to consumption. Intelligent analysis of data, information sharing, and cooperation is critical for developing these fast-evolving disciplines for everyone to benefit from a smarter, safer food supply chain.
The food sector generates vast data each day, such as assessments of production and supply facilities, chemical and microbial lab results, and research findings and records kept by government authorities. For instance, IBM Big Data Analytics has been evaluating massive quantities of data on transit temperatures, storage stability, and food consumption and distribution collected for the past several years. This data has been used to advance food safety standards by continuously upgrading supply networks and enabling quicker reactions to food safety problems.
Use of AI-Based Systems in Food Safety Applications
In recent times, AI-based systems have been widely deployed in food safety applications. Predicting the quality of food is critical for maintaining good food safety standards. Historically, food quality prediction relied on computer modeling of quality attributes such as aerobic plate cell number, lipid accumulation, and color intensity. Recently, various efforts to accomplish this goal have been reported using machine learning and artificial intelligence systems.
In a recent article, Lam et al.(2020) devised a system for estimating food quality based on deep learning that employs a wireless frequency-powered detector mote to forecast changes in food conditions. The radio frequency power at 915 MHz was employed to supply energy and detect the gas and moisture conditions in this study. To forecast the meal quality, gathered information on gas composition and moisture were employed in the deep learning network.
It was determined that pork and fish quality changes could be forecasted with 97.9 percent and 90.6 percent accuracy, respectively. As related technology advances, precision will inevitably exceed 99 percent. This indicates that virus development may be accurately predicted using environmental variables such as temperature, moisture, and oxygen concentration.
AI-Systems in Food Safety Industry around the World
As the popularity of AI-based food safety systems has grown, various large-scale data collecting and analytical systems have been created. For example, a prominent restaurant chain in the United States (The Cheesecake Factory) gathers massive amounts of data on transit temperatures, storage stability, and food shortages, which IBM Big Data Analytics evaluates. The tainted food can be swiftly returned from all locations when an issue arises.
Similarly, Wal-Mart Stores Inc. employs a Sustainable Paperless Auditing and Record-Keeping (SPARK) technology that transfers data (such as food temperature) to a web-based archiving program automatically. Within a month, health officials assessed the core cooking temperatures of rotisserie chickens ten times, private researchers 100 times, and SPARK 1.4 million times. Thus, a large amount of data is collected via SPARK that may be used to rapidly detect undercooked chicken.
An intriguing case study conducted by Aiforia used a deep neural network to build an artificial intelligence model (AI-model) to identify the multi-class separation of skin from Atlantic salmon. The AI model was created to provide accurate spatial assessments of all the succeeding skin layers and then applied to samples obtained from commercially produced Atlantic salmon.
The AI-model results indicated adaptive skin behavior, showing spatial changes in skin tissues linked to time in the water, developmental stage, and operating situations.
Future Directions
The method by which food safety experts assess risks and make choices will change as the food sector receives access to better data. New operational issues can emerge, especially in the areas of business disruption, perceived accountability, and data exchanges. Companies that are early adopters will lead the path for determining the viability and return on investment of applying these food safety solutions.
Several industry-wide campaigns may include (i) investing in food researchers and staff members to become data-savvy, (ii) specifying how AI and ML algorithms are used in regulatory systems, and (iii) forming inclusive alliances with strategic and operational stakeholders to enhance global food safety programs.
Continue reading: Automation in the Food Analysis Industry
References and Further Reading
Aiforia.com. (2022) Food safety case study: salmon skin analysis by AI. [online] Available at: https://www.aiforia.com/blog/food-safety-case-study
Imandar, T. (2021). Big data for Food Safety and Quality Assurance. [online] Available at: https://www.linkedin.com/pulse/big-data-food-safety-quality-assurance-tanveer-inamdar-/
Kim, S.-S., & Kim, S. (2022). Impact and prospect of the fourth industrial revolution in food safety: Mini-review. Food Science and Biotechnology. Available at: https://doi.org/10.1007/s10068-022-01047-6
Lam, M. B., Nguyen, T. -H., & Chung, W. -Y. (2020). Deep Learning-Based Food Quality Estimation Using Radio Frequency-Powered Sensor Mote. IEEE Access, 8, 88360-88371. Available at: https://ieeexplore.ieee.org/abstract/document/9089003
Sveen, L., Timmerhaus, G., Johansen, L.-H., & Ytteborg, E. (2021). Deep neural network analysis - a paradigm shift for histological examination of health and welfare of farmed fish. Aquaculture. Available at: https://doi.org/10.1016/j.aquaculture.2020.736024
Disclaimer: The views expressed here are those of the author expressed in their private capacity and do not necessarily represent the views of AZoM.com Limited T/A AZoNetwork the owner and operator of this website. This disclaimer forms part of the Terms and conditions of use of this website.