Ultimately, the purpose of volcanology is to predict upcoming volcanic eruptions. As technology advances, the role of sensor and data analysis in volcanic prediction is becoming standard practice amongst geologists. Although experts have uncovered certain trends, these eruptions make reliable forecasts challenging.
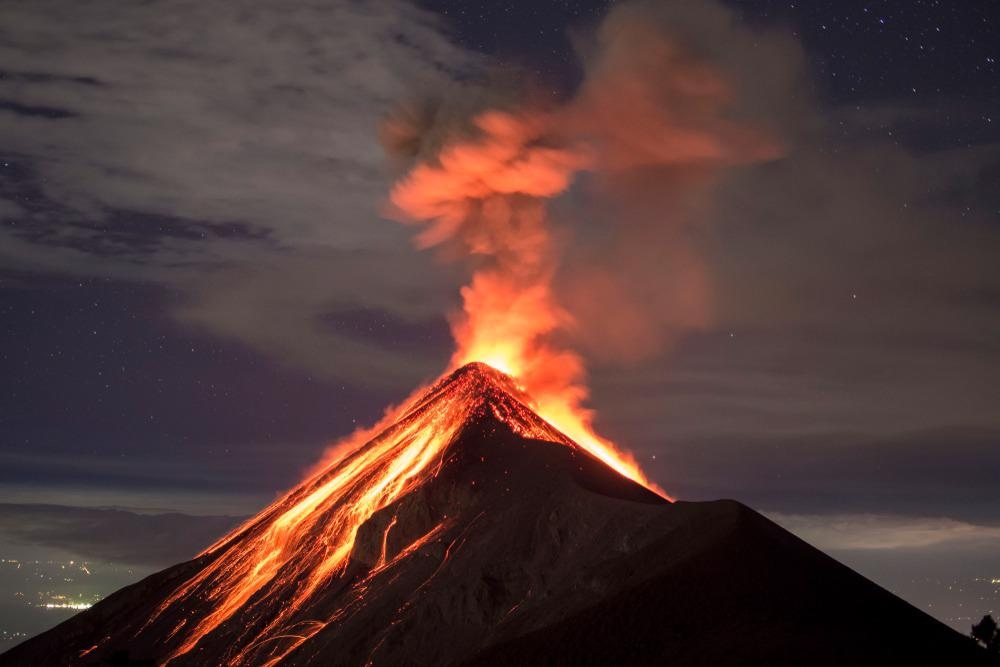
Image Credit: fboudrias/Shutterstock.com
To effectively describe volcanic regimes, a data reduction process that generates just a small number of characteristics is the most critical factor.
Machine Learning and Volcanic Eruptions
Volcanic activity may be predicted with some degree of accuracy using seismic data, one of the most important datasets available. Distinctive event identification and categorization can take a long time, if not impossible, to do manually.
The first step toward predictive approaches can be seen from the Material Failure Forecast Method (FFM), an automated categorization procedure.
Feature vectors should be constructed to convey the maximum information about the source, minimizing the impacts of, for example, route and location. In many circumstances, the characteristics of phenomena might be distinct from the physical model that describes them. When scientists and analysts are unsure of the source of an earthquake signal, machine learning (ML) can provide another source of confidence.
Cluster Analysis (CA) is one of the most commonly utilized unsupervised approaches in volcanic seismology. Seismic data from the volcano Piton de la Fournaise was clustered using spectral analysis.
On Raoul Island, New Zealand, Self-Organizing Maps (SOM) was used in the field of volcano-seismology. The Scilab environment was used to apply hierarchical clustering to findings of SOM tremor research at Ruapehu and Tongariro in New Zealand. Etna volcanic tremors were studied using a similar technique in MATLAB.
Landslide susceptibility and volcanic seismic data were used to determine the end date of an eruption at Telica (Nicaragua) and Nevado del Ruiz (Colombia), respectively, using supervised Logistical Regression (LR) models.
An earthquake prediction technique based on 30 years of data from Indonesian volcanoes was also tested using multinomial Logistic Regression and other approaches.
Methodology and Procession of Data
The majority of the approaches currently utilized attempt to categorize discrete seismic events, meaning they are defined by a specific start and finish time. As a result, detection and categorization are often discrete steps.
Hidden Markov Models (HMMs) capable of processing continuous input, such as continuous HMMs, may extract and categorize data in a single possible real-time step. HMMs are finite-state computers that represent sequential patterns in which time direction is critical. This is typical of seismic data from volcanoes. P waves, for example, always arrive first. Many publications have utilized classifiers based on HMMs to classify volcano seismic data. Some volcano observatories, like Colima and Popocatepetl in Mexico, utilize HMM regularly.
Other commonalities among the applications described in the literature include data captured at a single station to derive feature vectors. Classification techniques for multi-station networks are rare.
At Piton de la Fournaise, a Random Forest (RF) system was put into use, where tremor observations were classified, and the fundamental frequencies associated with various eruptions were identified using a multi-station technique at the same volcano.
Developments of Machine Learning in Volcanology and Future Aspects
Machine Learning approaches will have an ever-increasing influence on how the research and modeling of volcanoes are undertaken, how they are monitored, and how their danger is assessed.
Monitoring equipment on volcanoes is rising, but this increases the amount of information that must be analyzed in real-time. This is especially true during eruptions when the information is most critical.
ML can offer the right tools to go through extensive data and detect minor trends that might suggest instability, preferably well before eruptions.
Generalization is an important consideration as ML models need to be built that can be used on a variety of volcanoes, for example, when historical data is unavailable for training specialized models. Easy installation methods and integration into current monitoring systems should also be made available to encourage the widespread usage of ML technologies at volcanic observatories. Open-source software is one option that could be considered. One of the most critical things observatories can do to advance machine learning and data science is to supply high-quality training data for academics, developers, and data scientists.
Developing credible training sets in many domains of volcanology should take advantage of readily available open access data, both from the ground and from satellites. "Scientific rivalry" between research organizations employing different ML algorithms will be made simpler by the availability of "standard" training datasets that can be downloaded by anybody.
Continue reading: Providing Hope in Natural Disasters with Rescue Robots
References and Further Reading
A.Messina, H. (2011). Pattern recognition of volcanic tremor data on Mt. Etna (Italy) with KKAnalysis—A software program for unsupervised classification. Computers and Geosciences, 953-961. https://doi.org/10.1016/j.cageo.2011.03.015
Cortés, G., Carniel, R., Ángeles Mendoza, M. and Lesage, P., (2019) Standardization of Noisy Volcanoseismic Waveforms as a Key Step toward Station‐Independent, Robust Automatic Recognition. Seismological Research Letters, 90(2A), pp.581-590. https://doi.org/10.1785/0220180334
Ramis, R., Garcia, A., Marrero, J., De la Cruz-Reyna, S., Carniel, R. and Vila, J., (2018). Volcanic and volcano-tectonic activity forecasting: a review on seismic approaches. Annals of Geophysics, 61(Vol 61 (2018). https://doi.org/10.4401/ag-7655
Biswajeet Pradhana, S. L. (2010). Landslide susceptibility assessment and factor effect analysis: backpropagation artificial neural networks and their comparison with frequency ratio and bivariate logistic regression modelling. Environment Modelling and Software, 747-759. https://doi.org/10.1016/j.envsoft.2009.10.016
Ren, C. (2020). Machine Learning Reveals the Seismic Signature of Eruptive Behavior at Piton de la Fournaise Volcano. Geophysical Research Letters. https://agupubs.onlinelibrary.wiley.com/doi/10.1029/2019GL085523
Carniel, R. and Raquel Guzmán, S., (2021) Machine Learning in Volcanology: A Review. Updates in Volcanology - Transdisciplinary Nature of Volcano Science. https://www.intechopen.com/chapters/73667
Disclaimer: The views expressed here are those of the author expressed in their private capacity and do not necessarily represent the views of AZoM.com Limited T/A AZoNetwork the owner and operator of this website. This disclaimer forms part of the Terms and conditions of use of this website.