Despite its wide range of uses, there are some problems with traditional atomic force microscopy (AFM) techniques. A high level of skill set and human interference is required for this procedure and is very time-consuming. In this article, the use of artificial intelligence (AI) in AFM and its combined benefits has been described.
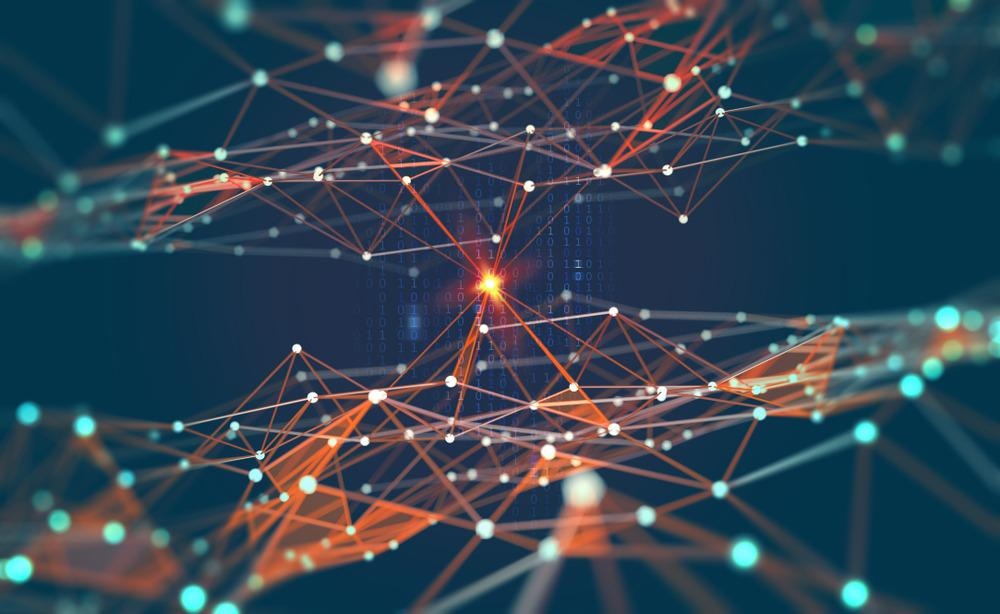
Image Credit: Yurchanka Siarhei/Shutterstock.com
Atomic Force Microscopy
Atomic Force Microscopy (AFM) is a potent technology that permits the imaging of practically any surface along with polymers, ceramics, composites, glass, and biological materials. It is a surface scanning technique that has sub-nanometer scale resolution. Adhesion strength, magnetic forces, and mechanical characteristics are just a few of the various forces that may be measured and localized with an AFM. It uses a cantilever and a sharp tip, around 10 to 20 nm in diameter, to perform AFM.
Limitations of AFM
AFM's single scan picture size is a drawback compared to that of the scanning electron microscope (SEM). A region of a few square millimeters can be imaged in a single pass by the SEM with a depth of field of a few millimeters.
It can only scan an area of 150x150 micrometers, whereas the AFM can only scan an area of 10-20 micrometers in height and 150x150 micrometers in area. Using parallel probes in a manner similar to millipede data storage can increase the size of the scanned region for AFM.
AFM's scanning speed is also a drawback. It has long been known that an AFM cannot scan images as quickly as an SEM, and a typical scan takes several minutes. In contrast, an SEM can scan images in near real-time, although with a lower resolution.
Thermal drift in an AFM picture might make it difficult to accurately measure distances between topographical elements on the image because of the comparatively slow scanning rate. It was hypothesized that numerous fast-acting designs, including what is being referred to as video AFM (acceptable quality pictures are being produced at a video rate: quicker than the usual SEM), might boost microscope scanning productivity. Several approaches have been developed to reduce the distortions in images caused by thermal drift.
Atomic Force Microscopy and Artificial Intelligence
Machine learning and artificial intelligence (AI) have the potential to fundamentally alter the way we live and work, and picture analysis is one of the most promising applications of AI. High-resolution optical imaging and mechanical characterization of many different materials, such as living cells and proteins, may be achieved using AFM.
Single-molecule measurements of protein-protein or receptor-ligand interactions may also be made using AFM in living cells. However, high-resolution imaging and force measurements using AFM and data analytics are time-consuming and require particular skill sets and ongoing supervision. Even though significant progress has been made in the field of AI and machine learning (ML) over the past several years, the potential of AI-AFM techniques in live-cell characterization has been almost entirely ignored.
How Could Artificial Intelligence Systems be Applied?
Image Recognition
Facial recognition for security and self-driving vehicle interpretation are two of the most common uses of image recognition, both of which use supervised machine learning.
The computer will begin to train a neural net where each layer "teaches" the computer a different component of facial recognition through the use of machine learning methods. For example, the first layer may "teach" the ability to recognize edges. Later layers may be able to distinguish between distinct facial features, such as an eye or a nose. All of this culminates in the ultimate objective of being able to identify an individual through images, which may be detected at yet another layer. AFM pictures can also benefit from this technique.
Particle Identification
Customized image analysis is a special strength of machine learning. The complexity of the AFM picture includes several rubbers embedded with nanoparticles that are difficult to differentiate from the underlying material. Particle analysis is a typical need in these photos; however, it is difficult to identify the particles for future analysis. A model is built to detect particles in this group of photos using machine learning.
Future of AI-Based AFM
Interlinking AFM and artificial intelligence will be beneficial for various sectors and industries. For example, biomedicine is one example that can be greatly benefitted from atomic force microscopy. Various improvements and modifications in AFM over the last two decades have made many things possible such as observing biological samples from macroscopic to microscopic level, also from molecular to submolecular resolution can be attained through AFM and AI combination.
ML can be used to tune autonomous instruments to run AFM measurements. Multiple parameters, including gains, scan rate, and setpoint, must be tuned during real-time picture capture when using an AFM equipment
The field of atomic force microscopy is ever-growing. With the continued advances in artificial intelligence, the future of AI-based AFM is likely to become commonplace. AI systems can be faster compared to the present techniques by taking large datasets. Data mining and augmentation techniques can help in this.
Continue reading: AI-Enhanced Innovation.
References and Further Reading
Boyuan Huang, Z. L. (2018). Artificial Intelligent Atomic Force Microscope Enabled by Machine Learning. Nanoscale. https://pubs.rsc.org/en/content/articlelanding/2018/nr/c8nr06734a
JaydeepRade, J. (2021). AI Guided Measurement of Live Cells Using AFM. IFAC-PapersOnLine, 316-321. https://www.sciencedirect.com/science/article/pii/S2405896321022370
Kai-ChihChang, Y.-W. C.-H.-W. (2012). Atomic force microscopy in biology and biomedicine. Tzu Chi Medical Journal, 162-169. http://dx.doi.org/10.1016/j.tcmj.2012.08.002
Singh, R. B. (2014). Atomic Force Microscopy: A Source of Investigation in Biomedicine. International Journal of Electronic and Electrical Engineering, 59-66.
Disclaimer: The views expressed here are those of the author expressed in their private capacity and do not necessarily represent the views of AZoM.com Limited T/A AZoNetwork the owner and operator of this website. This disclaimer forms part of the Terms and conditions of use of this website.