Non-destructive testing (NDT) has gained significant traction due to its increasing requirements and applications in manufacturing industries. The diversity of the applications of NDT and the stringent quality control conditions imposed by the relevant industries has made researchers look for accurate, inexpensive, and time-efficient methods.

Image Credit: N_Sakarin/Shutterstock.com
Lately, artificial intelligence (AI) and machine learning (ML) algorithms have proven themselves as effective numerical tools. These algorithms are being widely used in many fields such as control and automation, signal processing, materials engineering, and computational fluid dynamics.
Non-destructive testing is one of the most important industrial applications that can benefit directly from machine learning and artificial intelligence algorithms that have recently evolved.
The conventional NDT methods are susceptible to the effects of human factors, which can lead to different results for the same test, thus, making automation and incorporation of machine learning techniques desirable.
The breakthroughs and achievements in machine learning have recently been introduced in non-destructive testing, and already the results are promising. The machine learning algorithms can analyze, inspect, and examine the integrity of the material structure by interpreting multiple signals or images.
The use of non-destructive testing has become indispensable in the industrial sector. They are being used in manufacturing industries to detect flaws, including cracks, gaps, pores, leaks, and fractures on the surface and inner layers of materials mostly caused by processing, contraction, stresses, and hammering while maintaining durability and originality of the materials after inspection.
Despite the availability of numerous technologies to aid NDT workers in spotting faults, a basic visual inspection still holds a great weightage of all quality checks in the automotive and aerospace industries. A quality visual control depends upon an optimum output of both accuracy and productivity, and incorporating machine learning algorithms in NDT can do that.
This article aims to explore the non-destructive testing method published in mathematics, MDPI, known as a fluorescent penetrant inspection (FPI), and its automation using machine learning algorithms.
Fluorescent Penetrant Inspection (FPI)
FPI is a type of NDT in which a fluorescent dye is applied to the surface of impenetrable material to detect the cracks, leaks, and pores caused by machining, grinding, and processing.
The FPI procedure includes cleaning and prepping the inspection surface, applying the dye penetrant, removing the surplus penetrant, and recording the highlighted surface defects. The main drawback in FPI is the last step, which is evaluation and recording, where an expert operator must work in the dark room to manually carry out the evaluation procedure.
The automated FPI system using appropriate image processing and machine learning algorithms can enhance the reliability of evaluations and recordings. An operator in this procedure can be replaced by mini-drones, robots, and cameras to capture the pictures of a surface at different angles. These pictures can be analyzed using computer vision, image processing, and machine learning algorithms to detect the flaws.
Automated FPI System
The researchers have proposed an automated FPI system that comprises a robot for component handling, a machine vision solution for defect detection, and an intelligent algorithm that allows real-time image processing and extremely robust decision-making.
This system also consists of a human-machine interface that enables the artificial intelligence algorithms to learn from expert personnel and make decisions in ultraviolet light.
The proposed approach also consists of an image multiplication technique to enlarge the available dataset required for training.
FPI Automation Architectures and ML Techniques for Spot Identification
The first architecture presented in mathematics, MDPI is based on a ‘direct spot classifier’. The neural network is used to process the spot indicated in the original image.
The inputs to the neural network are the pixels of the image, while output is the classification of the spot. This architecture can take full advantage of both deep neural networks as well as the multi-layer perceptron.
In the second architecture proposed here, the probabilistically extracted features from each spot are processed with the neural network. This lowers the requirement of the input dataset, but it can identify limited classes of indications.
This architecture is based on the ‘indirect spot classifier’ where deterministic features of the image in the form of area, orientation, major axis, and minor axis are used as an input of the neural network.
Finally, the t-distributed stochastic neighbor embedding (t-DSNE), an ML algorithm was applied for a high-dimensional data visualization. This approach was used to examine and validate the sample distribution at three distinct classification levels.
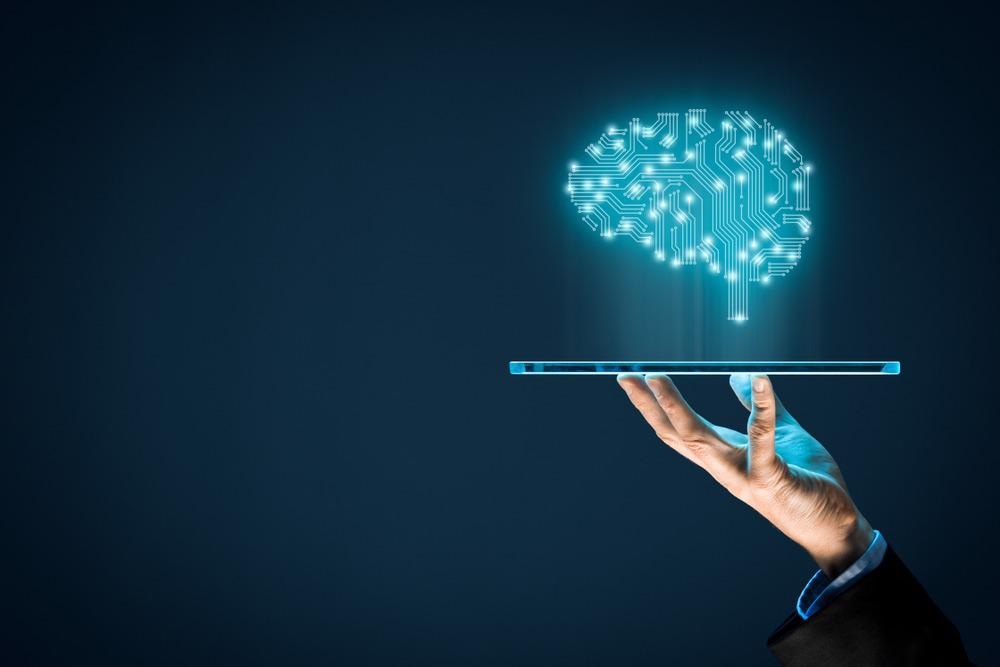
Image Credit: Jirsak/Shutterstock.com
Benefits of an Automated FPI System
The simulations performed by the researchers revealed that the proposed system can detect the fault and classify it based on the training of an ML classifier using the experience of an expert operator.
Incorporating a robotic manipulator and a high-speed decision-making algorithm based on the neural network can significantly increase the FPI efficiency and productivity. The operators can also analyze and validate the results remotely, which can reduce the cost of the process.
Industrial Applications of an Automated FPI System
The aviation industry can benefit from the automated FPI system as it can help them ensure safety, airworthiness, and efficiency by detecting faults before they cause significant damage.
Similarly, the mechanical and material industries can use this system to detect the flaws in their manufactured products and increase their productivity and efficiency at a comparatively lower cost and in less time.
Current Challenges
The drawbacks associated with the proposed automated FPI include the limitation of information in input data due to feature extraction and a low number of available samples inhibiting ML algorithms' learning. The accuracy of the automated FPI system is highly susceptible to the noise level in the original images.
Future of Machine Learning in NDT
The only apparent solution to drawbacks associated with the current NDT methods is machine learning. The ML and AI algorithms can make the NDT process simple, time-efficient, and inexpensive; however, the limitations of the input dataset for training and accuracy of the algorithms need to be addressed.
Continue reading: Mapping Malnutrition and Food Security with Machine Learning
References and Further Reading
Harley, J. B. and Sparkman, D. (2019) ‘Machine learning and NDE: Past, present, and future’, AIP Conference Proceedings, 2102(May). Available at: https://doi.org/10.1063/1.5099819.
Kraljevski, I. et al. (2021) ‘Machine Learning for Anomaly Assessment in Sensor Networks for NDT in Aerospace’, IEEE Sensors Journal, 21(9), pp. 11000–11008. Available at: https://doi.org/10.1109/JSEN.2021.3062941.
Niccolai, A. et al. (2021) ‘Machine learning-based detection technique for ndt in industrial manufacturing’, Mathematics, 9(11), pp. 1–16. Available at: https://doi.org/10.3390/math9111251.
Shipway, N. J. et al. (2021) ‘Using ResNets to perform automated defect detection for Fluorescent Penetrant Inspection, NDT and E International, 119(December 2020), p. 102400. Available at: https://doi.org/10.1016/j.ndteint.2020.102400.
Disclaimer: The views expressed here are those of the author expressed in their private capacity and do not necessarily represent the views of AZoM.com Limited T/A AZoNetwork the owner and operator of this website. This disclaimer forms part of the Terms and conditions of use of this website.