Forming a branch of computer science and artificial intelligence (AI), machine learning enables a software system to gradually improve its accuracy in predicting outputs using algorithms and historical data. In a way, the system emulates the human ability to learn by improving its predictive accuracy over time.
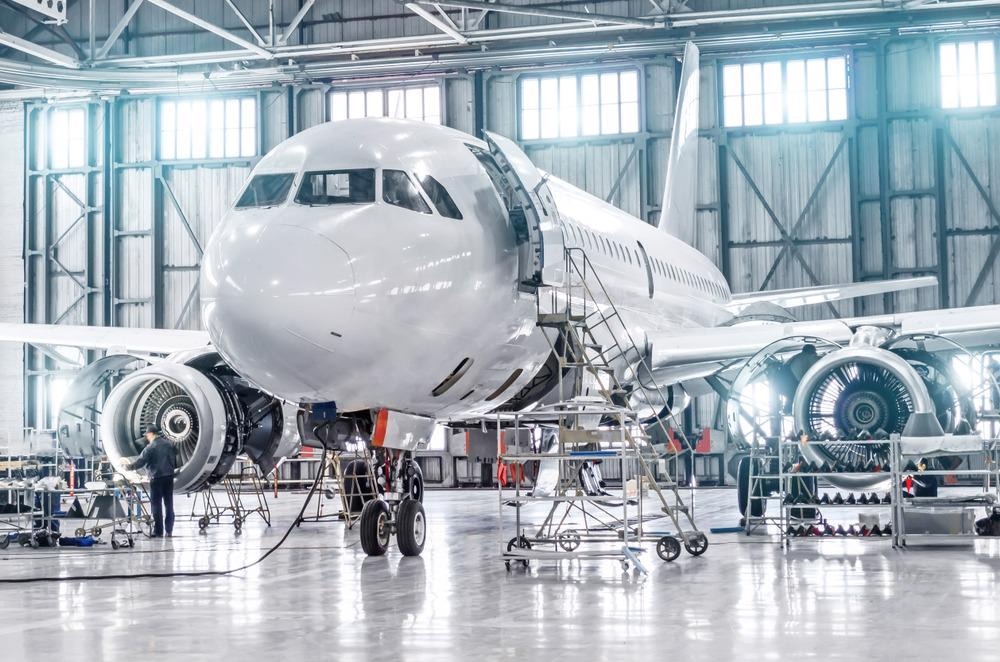
Image Credit: aapsky/Shutterstock.com
Machine learning is quickly reshaping the world of science. Its applications are endless, and it can be utilized in almost every industrial setting to usher in a new era of growth and technology.
One such example is the aerospace industry, where many high-dimensional, multi-objective, multi-constraint problems can be addressed in optimizing aircraft design, analysis, and manufacturing processes using data-driven techniques.
Safety-critical applications such as testing new combinations of elements for environmental barrier coatings in the aerospace industry can be revolutionized using machine learning.
Environmental Barrier Coatings
Critical parts in aircraft jet engines and the components responsible for the structural integrity of hypersonic jets, rockets, and space launch vehicles are sealed and protected from harsh environmental, thermal, and atmospheric conditions using environmental barrier coatings (EBCs).
In aero-engines designed to run at relatively high temperatures, silicon-based non-oxide ceramic matrix composites (CMCs) are used due to better retention of mechanical properties at high temperature operating conditions. However, even these CMCs, used for components such as rotor blades, can suffer from some degree of deformation and spallation in the presence of high temperature water and oxygen molecules if left unprotected.
It is here that EBCs can be utilized. Rare-earth silicates, currently one of the most popular choices for EBCs, improve ceramics' mechanical and thermal properties for operation at high temperatures, protect parts from severe corrosion, oxidation, thermal fatigue, and damage due to stresses imposed by supersonic flight. They achieve this due to their stability at high temperatures, anti-corrosive and oxidation-resistant properties.
There are some limitations of rare-earth silicates, however. They can cause some degree of deterioration in the performance of the jet engines. It is a tradeoff that allows for better protection of components from harsh environmental conditions, at the cost of some performance.
Still, this tradeoff can be bypassed by using alternative combinations of elements for next-generation EBCs. As with any technology, innovations and breakthroughs would naturally be required if higher performance metrics are to be reached.
Testing the Viability of Rare-Earth Phosphates for Use in EBCs using Machine Learning
Researchers at Rensselaer Polytechnic Institute aimed to unravel the complexities of using a completely different material for EBCs, to identify possible candidacy of multi-component rare-earth phosphates for making the next generation of EBCs instead of rare-earth silicates. Their investigations were recently published in AAIA Journal.
The advantage of using rare-earth phosphates is that they will open an entirely new world of possibilities. Suppose the right composition and microstructure can be determined. In that case, they will inevitably lead to unprecedented gains in the performance of jet engines at high temperatures by protecting the necessary components without being a deterrent to performance as much.
Although switching to rare-earth phosphates for use as EBCs would be ideal, the process is costly, with many researchers taking a trial and error approach to investigations.
One potential answer to these problems is machine learning. Empirical trial and error are considered to be impractical where the optimum composition of a material, as well as its microstructure and properties, must be determined from a pool of endless possibilities of combinations.
The team behind the project at Rensselaer, however, aimed to use a unique methodology to resolve this by pairing physics-based modeling techniques with machine learning. Essentially, sophisticated computer algorithms will be used to simulate different combinations and compositions of multi-component rare-earth phosphates in various possible configurations. This approach would allow for the efficient prediction of the optimum framework and design of next-generation EBCs that would reshape the future of high speed and space flight.
There are many advantages of using machine learning models that are prepared using collections of data obtained from high output multiscale simulations. The time and costs associated with designing and optimizing the framework, microstructure and composition of rare-earth phosphates for use in EBCs are greatly reduced.
The main challenge that may be faced in this approach will undoubtedly be the creation of an advanced computer algorithm capable of carrying out complex multiscale simulations and processing all the data obtained to predict the best composition and framework for the new EBCs.
Prospects
Innovation is the key to progress, and even on a small scale, it can prove to be transformative. With support from the National Science Foundation and collaboration with companies like General Electric Global Research, the Rensselaer team's effort of designing next-generation EBCs has traction and may transform the future of aerospace transportation systems.
Continue reading: Detecting Radiation-Induced Defects with a New Machine Learning Platform.
References and Further Reading
Brunton, S. L., Nathan Kutz, J., Manohar, K., Aravkin, A. Y., Morgansen, K., Klemisch, J., & ... & McDonald, D. (2021) Data-driven aerospace engineering: reframing the industry with machine learning. AIAA Journal, 2820-2847. Available at: https://arc.aiaa.org/doi/full/10.2514/1.J060131
Gallagher, J. H. (2021) Machine Learning Tests Abilities of Rare-Earth Phosphates Given Atmospheric Extremes. Available at: https://news.rpi.edu/content/2021/09/29/machine-learning-tests-abilities-rare-earth-phosphates-given-atmospheric-extremes
Gandhi, A. S (2020) Materials Aspects of Thermal Barrier Coatings. Handbook of Advanced Ceramics and Composites: Defense, Security, Aerospace and Energy Applications, pp. 1443-1463. Available at: https://link.springer.com/
Xu, Y., Hu, X., Xu, F., & Li, K. (2017) Rare earth silicate environmental barrier coatings: present status and prospective. Ceramics International, pp. 5847-5855. Available at: http://dx.doi.org/10.1016/j.ceramint.2017.01.153
Disclaimer: The views expressed here are those of the author expressed in their private capacity and do not necessarily represent the views of AZoM.com Limited T/A AZoNetwork the owner and operator of this website. This disclaimer forms part of the Terms and conditions of use of this website.