Weed management is crucial in meeting the food demands of the growing population. Many traditional techniques—chemical and mechanical—have been employed to date for herbicide control, which has its disadvantages. This article covers a recent review ofthe various sophisticated sensors, UAVs, and machine learning available in the market for precision weed control. The review was published in the journal Chemical and Biological Technologies in Agriculture.
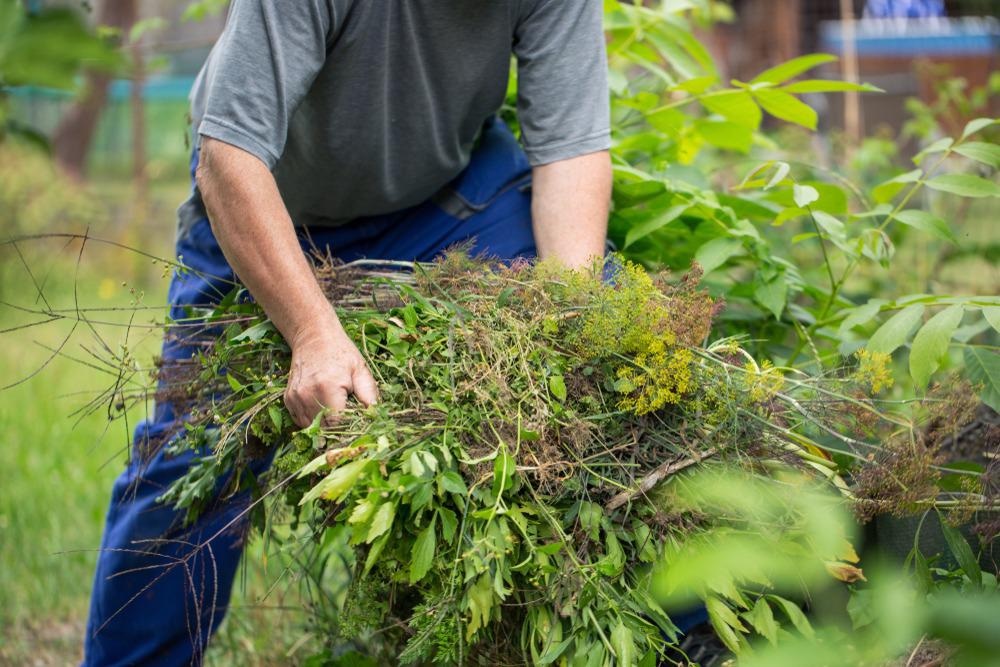
Image Credit: Simon Kadula/Shutterstock.com
Biotic threats like weeds, insects, fungi, bacteria, and viruses affect crop yield and quality. Researches intend to create strategies that decrease the harmful effects of the interspecific competition between crops and weeds, and recent technological advances may further contribute to this scope.
Weed competition results in drastic yield reduction in all major crops. Herbicides, which are the second-most sold pesticide in Europe, are used (Figure 1).
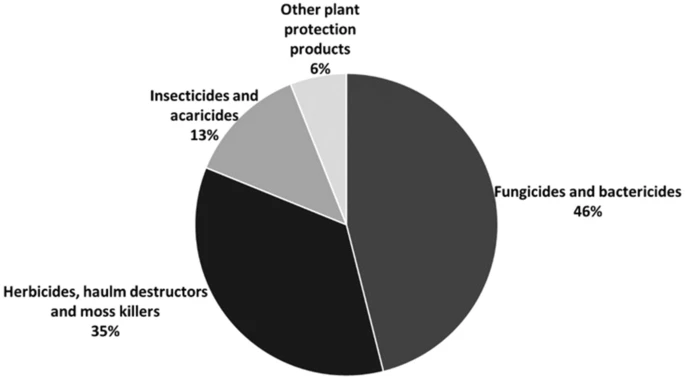
Figure 1. Percentage (of total volume in kilograms) of pesticide sales by category in Europe in 2018. Image Credit: Esposito, et al., 2021.
Weed Management Requires an Integrated Approach
It is anticipated that by 2050, the global population will quadruplicate, and the present production system cannot cope with the predicted increase in food demand. Climate change is also an additional challenge for the human food supply.
Weed management adds to these factors. Even though mechanical and chemical weed control is practiced, they have their own set of disadvantages—mechanical methods are scarcely efficient, and herbicides have a high ecological impact, which hinders them from being effective measures.
One approach that decreases the drawbacks of chemical and mechanical weed control is Integrated Weed Management (IWM). It is a combination of biological, chemical, mechanical, and/or crop management techniques and portrays a model to enhance the efficiency and sustainability of weed control. When compared to conventional methods, IWM integrates numerous agro-ecological aspects.
New Technologies for Site-Specific Weed Management
Precision agriculture depends on technologies that combine information systems, sensors, and informed management to improve crop productivity and minimize environmental impact.
It can be effectively applied to IWM with Unmanned aerial vehicles. Unmanned Vehicles systems are Terrestrial (UTV) or mobile Aerial (UAV) platforms that offer various advantages for the execution and monitoring of farming activities along with providing Site-Specific Weed Management (SSWM) (Figure 2).
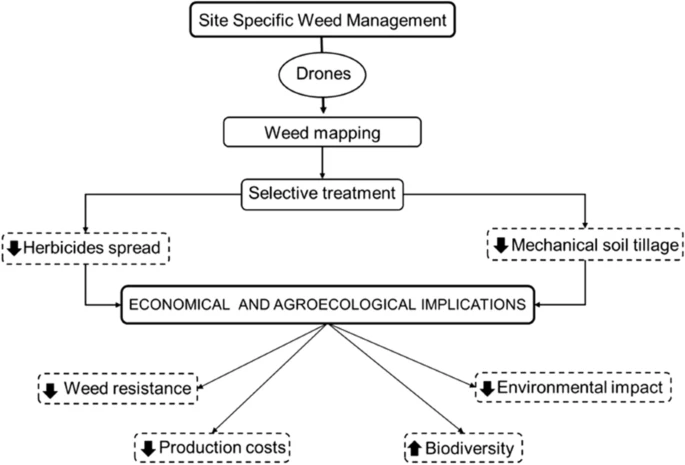
Figure 2. Site-specific weed management (SSWM) scheme realized by drones and its economical and agro-ecological implications. Image Credit: Esposito, et al., 2021.
UAVs Remote Sensing Techniques and Sensors
UAVs are economical, user-friendly, and versatile, making them a common tool in precision agriculture. These systems can be employed for various purposes based on the sensors they carry.
UAVs harbor many advantages like collecting easily deployable data in real-time, surveying areas with a high level of hazard, and collecting data even in unfavorable weather conditions. The sensors available are majorly categorized into three classes based on the spectral length and number they can record including RGB (Red, Green, Blue) or VIS (Visible) sensors, Multispectral sensors, Hyperspectral sensors.
RGB/VIS Sensors
The RGB or VIS sensors are the commonly available commercial cameras (Table 1).
Table 1. RGB cameras and their main specifications. Source: Esposito, et al., 2021.
Camera
model |
Sensor type and resolution [Mpx] |
Sensor
format |
Sensor Size [mm] |
Weight [kg] |
Price (approx.) [€] |
Canon EOS 5d Mark IV |
CMOS 30.4 |
Full Frame |
36.0 × 24.0 |
ca. 1.0 |
ca. 1000 |
Nikon D610 |
CMOS 24.3 |
Full Frame |
36.0 × 24.0 |
ca. 1.250 |
ca. 1000 |
Sony Alpha 7R II |
CMOS 42 |
Full Frame Mirrorless |
35.0 × 24.0 |
ca. 0.6 |
ca. 1200 |
Sony Alpha a6300 |
CMOS 24 |
Small Frame Mirrorless |
23.5 × 15.6 |
ca. 0.8 |
ca. 800 |
Panasonic Lumix DMC GX8 |
CMOS 20 |
Small Frame Mirrorless |
17.3 × 13 |
ca. 0.5 |
ca. 1000 |
Panasonic Lumix DMC GX80 |
DLMOS 16 |
Small Frame Mirrorless |
17.3 × 13 |
ca. 0.5 |
ca. 500 |
DJI Phantom 4 Pro * |
CMOS 20 |
Small Frame |
13.2 × 8.8 |
ca. 1.5 (with UAV) |
ca. 1500 (with UAV) |
DJI Mavic 2 Proa |
CMOS 20 |
Small Frame |
13.2 × 8.8 |
ca. 1.5 (with UAV) |
ca. 1500 (with UAV) |
a* UAV with already supplied camera. Payload not interchangeable
These sensors help calculate vegetation indices like Greenness Index (GI), the Green/Red Vegetation Index (GRVI), and Excessive Greenness (ExG). RGB data can be employed to generate a georeferenced orthomosaic.
Multispectral Sensors
The multispectral sensors are employed for a broader range of calculations of vegetation indices. Table 2 shows the widely used multispectral sensors, specific for UAV systems.
Table 2. Multispectral sensors and their main specifications. Source: Esposito, et al., 2021.
Camera model |
Resolution
[Mpx] |
Spectral
bands |
Ground sample distance [cm/px] |
Weight [kg] |
Price (approx.) [€] |
Micasense RedEdge-M |
1280 × 960
(1.2 Mpx per
EO band) |
Red, Green, Blue, Near-Infrared, Red Edge |
8 (per band) at 120 m AGL |
ca. 0.180 |
ca. 5000 |
Micasense RedEdge-
MX |
1280 × 960
(1.2 Mpx per
EO band) |
Blue, green, red, red edge, near infrared (NIR) |
8 (per band) at 120 m AGL |
ca. 0.231 |
ca. 5000 |
Micasense Altum |
2064 × 1544 (3.2 Mpx per EO band) 160 × 120 thermal infrared |
EO: Blue, green, red, red edge, near-infrared (NIR)
LWIR: thermal infrared 8–14 µm |
5.2 cm per pixel (per EO band) at 120 m AGL—81 cm per pixel (thermal) at 120 m |
ca. 0.405 |
ca. 6000 |
TertaCam MCAW 6 |
1.3 |
6 user selectable narrow bands (450–1000 µm) |
– |
ca. 0.550 |
ca. 17000 |
TetraCam ADC Lite |
3.2 |
Green, Red, Near-Infrared (NIR) |
– |
ca. 0.2 |
ca. 3000 |
TetraCam ADC Micro |
3.2 |
Green, Red, Near-Infrared (NIR) |
– |
ca. 0.09 |
ca. 3000 |
Parrot Sequoia + |
1.2 |
Blue, Green, Red, Red Edge, Near-Infrared (NIR) |
– |
ca. 0.7 |
ca. 5000 |
Multispectral sensors allow an extended range of vegetation indices to be monitored. Multispectral images are also used in machine learning applications.
Hyperspectral Sensors
The hyperspectral sensors record hundreds to thousands of narrow radiometric bands, in infrared and visible ranges. Each hyperspectral sensor can identify only a certain number of bands; hence the aim of the survey must be very clear.
Hyperspectral sensors are expensive when compared to RGB and multispectral sensors and they are bulkier. Table 3 lists some of the widely employed hyperspectral sensors in UAV applications.
Table 3. Hyperspectral sensors and their main characteristics. Source: Esposito, et al., 2021.
Camera
model |
Lens |
Spectral range [µm] |
Spectral bands [number and µm] |
Weight [kg] |
Price (approx.) [€] |
CUBERT |
Snapshot + PAN |
450–995 |
125 (8 µm) |
ca. 0.5 |
ca. 50000 |
Cornirg microHSI 410 SHARK |
CCD/CMOS |
400–1000 |
300 (2 µm) |
ca. 0.7 |
– |
Rikola Ltd. hyperspectral camera |
CMOS |
500–900 |
40 (10 µm) |
ca. 0.6 |
ca. 40000 |
Specim-AISA KESTREL16 |
Push-
broom |
600–1640 |
350 (3 – 8 µm) |
ca. 2.5 |
– |
Headwall Photonics
Micro-hyperspec X-series NIR |
InGaAs |
900–1700 |
62 (12.9 µm) |
ca. 1.1 |
– |
When compared to other sensors, the workflow for radiometric calibration is more complex.
Applications of UAVs to Weed Management
UAVs are ideal for identifying weed patches as they require shorter monitoring/surveying time and optimal control in the presence of obstacles. They can cover many hectares flying over the field offering photographic material for weed patches identification.
The images are later processed through a convolutional neural network, deep neural network, and object-based image analysis. Three types of cameras are used for weed patches identification: RGB, multispectral and hyperspectral cameras (Table 4).
Table 4. Weed patches identification by different types of camera (multispectral, RGB, hyperspectral). Source: Esposito, et al., 2021.
Crop |
Weed
(common name) |
Weed
(scientific
name) |
Type of camera |
Main
results |
References |
|
Palmer amaranth |
Amaranthus palmeri |
Hyperspectral camera |
Discriminate glyphosate-resistant from glyphosate-sensitive weeds |
[110] |
|
Spotted knapweed
Babysbreath |
Centaurea maculosa
Gypsophila paniculata |
Hyperspectral camera |
Detection invasive species affecting forests, rangelands, and pastures |
[111] |
|
Bunchgrass
Egyptian crowfoot grass
False amaranth
Awnless barnyard grass |
Phalaris minor
Dactyloctenium aegyptium
Digera arvensis
Echinochloa colona |
RGB
camera |
Identify different weeds |
[112] |
|
Ragwort |
Jacobaea vulgaris (Senecio jacobaea) |
Multispectral camera |
Discriminate weeds in pastures |
[113] |
|
Buffel Grass
Spinifex |
Cenchrus ciliaris
Triodia sp. |
RGB
camera |
Discriminate two different weeds |
[114] |
Beta vulgaris
Zea mays
Hordeum vulgare
Lens esculenta
Pisum sativum
Phaseolus vulgaris
Carthamus tinctorius
Cicer arietinum |
Kochia
Marestail
Common lambsquarters |
Bassia scoparia
Conyza canadensis
Chenopodium album |
Hyperspectral camera |
Discriminate glyphosate and dicamba resistant genotypes from sensitive genotypes |
[115] |
Triticum spp.
Triticosecale |
|
|
RGB
camera |
Comparison of cereal genotypes |
[116] |
Beta vulgaris |
Weeds |
|
Multispectral camera |
Discriminate crop vs weeds |
[98] |
Beta vulgaris |
Weeds |
|
Multispectral camera |
Discriminate crop vs weeds |
[85] |
Beta vulgaris |
Thistle |
Cirsium arvense |
Multispectral camera |
Discriminate crop vs weeds |
[117] |
Beta vulgaris |
Thistle
Wild
buckwheat
Ryegrass |
Cirsium arvense
Fallopia convolvulus
Lolium multiflorum |
Multispectral camera |
Discriminate crop vs weeds |
[101] |
Beta vulgaris |
Thistle |
Cirsium arvense |
Multispectral camera |
Discriminate crop vs weeds |
[112] |
Cicer arietinum |
Weeds |
|
Hyperspectral camera |
Discriminate crop vs weeds |
[118] |
Glycine max |
Palmer amaranth
Barnyardgrass
Large crabgrass |
Amaranthus palmeri
Echinochloa crus-galli
Digitaria sanguinalis |
RGB camera
Multispectral camera |
Assessment of crop injury from dicamba |
[102] |
Heliathus annuus |
Pigweed
Mustard
Bindweed
Lambsquarters |
Amaranthus blitoides
Sinapis arvensis
Convolvulus arvensis L
Chenopodium album L |
RGB camera
Multispectral camera |
Discriminate crop vs weeds |
[64] |
Hordeum vulgare |
Thistle
Coltsfoot |
Cirsium arvense
Tussilago farfara |
RGB
camera |
Discriminate crop vs weeds |
[119] |
Hordeum vulgare |
Thistle |
Cirsium arvense |
RGB
camera |
Discriminate crop vs weeds |
[99] |
Hordeum vulgare |
Thistle |
Cirsium arvense |
RGB
camera |
Discriminate crop vs weeds |
[100] |
Lactuca sativa |
Common groundsel
Shepherd's purse
Sow thistle |
Senecio vulgaris
Capsella bursa pastoris
Sonchus spp. |
Multispectral camera |
Discriminate crops vs weeds |
[120] |
Sorghum spp. |
Amaranth
Pigweed
Barnyard grass
Mallow
Nut grass
Fat Hen |
Amaranthus macrocarpus
Portulaca oleracea
Echinochloa crus-galli
E. colona
Malva spp.
Cyperus rotundus
Chenopodium album |
Hyperspectral camera |
Discriminate crop vs weeds |
[121] |
Triticum durum |
Wild oat
Canarygrass
Ryegrass |
Avena sterilis
Phalaris canariensis
Lolium rigidum |
Multispectral camera |
Discriminate crop vs weeds |
[122] |
Triticum durum |
Wild oat
Canarygrass
Ryegrass |
Avena fatua
Phalaris canariensis
Lolium rigidum |
Hyperspectral camera
Multispectral camera |
Discriminate crop vs weeds |
[105] |
Triticum sp. |
Thistle |
Cirsium arvense |
RGB
camera |
Discriminate crop vs weeds |
[99] |
Triticum spp. |
Weeds |
|
Hyperspectral camera |
Discriminate crop vs weeds |
[118] |
Vitis vinifera |
Bermuda
grass |
Cynodon
dactylon |
RGB
camera |
Discriminate crop vs weeds |
[123] |
Zea mays |
Weeds |
|
Multispectral camera |
Discriminate crop vs weeds |
[124] |
Zea mays |
Common lambsquarters
Thistle |
Chenopodium album
Cirsium arvense |
Multispectral camera |
Discriminate monocotyledons (crops) vs dicotyledons (weeds) |
[104] |
Zea mays |
Common lambsquarters
Thistle |
Chenopodium album
Cirsium arvense |
Multispectral camera |
Discriminate crop vs weeds |
[101] |
Zea mays |
Mat amaranth
Johnsongrass |
Amaranthus blitoides
Sorghum halepense |
Multispectral camera |
Discriminate crop vs weeds |
[125] |
These cameras identify weed patches with better precision depending on flying altitude, camera resolution, and UAV used.
Conclusion
UAVs and machine learning methods permit the identification of weed patches in a cultivated field with accuracy and can enhance weed management sustainability. Furthermore, weed patch identification by UAVs can facilitate integrated weed management (IWM), reduce the selection pressure.
Also, imaging analysis can help in the study of weed dynamics in the field and their interaction with the crop. Specific algorithms can be trained to manage weeds removal by Autonomous Weeding Robot (AWR), with the help of mechanical means or herbicide spray.
To further expand this approach to real agricultural contexts, novel information on weed population dynamics and their competition with crops is required.
Continue reading: Reducing Water Waste with Robotic Irrigation.
Journal Reference:
Esposito, M., Crimaldi, M., Cirillo, V., Sarghini F., Maggio, A. (2021) Drone and sensor technology for sustainable weed management: a review. Chemical and Biological Technologies in Agriculture, 8(18). Available at: https://doi.org/10.1186/s40538-021-00217-8.
References and Further Reading
- Oerke, E-C (2006) Crop losses to pests. The Journal of Agricultural Science. doi.org/10.1017/S0021859605005708.
- Guglielmini, A. C., et al. (2017) Competitive ability of five common weed species in competition with soybean. International Journal of Pest Management, 63, pp. 30–36 doi.org/10.1080/09670874.2016.1213459.
- Kaur, H., et al. (2019) A REVIEW on different weed management approaches. International Journal of Current Microbiology and Applied Sciences, 8, pp. 28–54. doi.org/10.20546/ijcmas.2019.808.328.
- Korav, S., et al. (2018) Weed pressure on growth and yield of groundnut (Arachis hypogaea L.) in Meghalaya, International Journal of Current Microbiology and Applied Sciences, 7, pp. 2852-2858. doi.org/10.20546/ijcmas.2019.808.328.
- Thompson C, R., et al. (2019) Ciampitti IA, Vara Prasad P, eds.
- Zohaib, A., et al. (2016) Weeds Cause Losses in Field Crops through Allelopathy. Notulae Scientia Biologicae, 8, pp. 47–56. doi.org/10.15835/nsb819752.
- Cirillo, V., et al. (2018) Crop-weed interactions in saline environments. European Journal of Agronomy, 99, pp. 51–61. doi.org/10.1016/j.eja.2018.06.009.
- Hauvermale, A. L., et al. (2019) Phenological Plasticity of Wild and Cultivated Plants. IntechOpen. doi.org/ 10.5772/intechopen.85070.
- Guo, L., et al. (2018) Genomic clues for crop-weed interactions and evolution. Trends in Plant Science, 23, pp. 1102-115. doi.org/10.1016/j.tplants.2018.09.009.
- Kumar, A., et al. (2019) Weed Seed Bank: impacts and management for future crop production. doi.org/10.1007/978-981-32-9783-8_12.
- Smith J, D., et al. (2019) Host range of the invasive tomato pest tuta absoluta meyrick (Lepidoptera: Gelechiidae) on solanaceous crops and weeds in Tanzania. BioOne. doi.org/10.1653/024.101.0417.
- Roshan, P., et al. (2019) Global weed-infecting geminiviruses BT-geminiviruses: impact, challenges and approaches. Berlin: Springer, pp. 103–21.
- Srinivasan, R., et al. (2013) Aphid-Borne Virus Dynamics in the Potato-Weed Pathosystem. Insect Pests Potato.
- Eshed, N (1975) Role of wild grasses in epidemics of powdery mildew. Phytopathology. 65, pp. 57–63.
- Adkins, S., et al. (2014) Biology, ecology and management of the invasive parthenium weed (Parthenium hysterophorus L.). Pest Management Science, 70, pp. 1023–1029. doi.org/10.1653/024.101.0417.
- Tanveer, A., et al. (2015) Interference and management of parthenium: The world’s most important invasive weed. Crop Protection, p. 68. doi.org/10.1016/j.cropro.2014.11.005.
- Khan, M. R & Somvanshi V. S (2017) Rao U. Emerging nematode pest of rice, wheat and onion. Rice Root-Knot Nematode Popular Kheti. 3, pp. 53–55.
- Ersin, A., et al. (2017) The effect of weed competition on apple fruit quality. Notulae Botanicae Horti Agrobotanici Cluj-Napoca, 45, pp. 120–125. doi.org/10.15835/nbha45110556
- Yadav, T., et al. (2018) Assessment of critical period of crop-weed competition in forage cowpea (Vigna unguiculata) and its effect on seed yield and quality. Indian Journal of Agronomy, 63, pp. 124–127.
- Hall C, W., et al. (2013) Vacuum treatment of milk. In: Vacuum Technology Transactions. Pergamon.
- Sabra, F. S & Mahmoud, M. S (2015) Utilization of herbicidal treatments to overcome weed problems in utilization of herbicidal treatments to overcome weed problems in peppermint (Mentha piperita L.) cultivation under Egyptian conditions. Asian Journal of Agriculture and Food Sciences, 3, pp. 1–7.
- Mantle, P (2020) Comparative ergot alkaloid elaboration by selected plectenchymatic mycelia of Claviceps purpurea through sequential cycles of axenic culture and plant parasitism. Biology. 9, p. 41. doi.org/10.3390%2Fbiology9030041.
- Kumar, R., et al. (2016) Lantana camara: an alien weed, its impact on animal health and strategies to control. Journal of Experimental Biology and Agricultural Sciences, 4, pp. 321–337. doi.org/10.18006/2016.4(3S).321.337.
- Denisow-Pietrzyk, M., et al. (2019) Asteraceae species as potential environmental factors of allergy. Environmental Science and Pollution Research, 26, pp. 6290–6300. doi.org/10.1007/s11356-019-04146-w.
- Allan, S & Adkins, S. W (2018) Impact of parthenium weed on human and animal health. Parthenium Weed: Biology, Ecology and Management, 7, pp. 7–105.
- Korres, N. E., et al., (2019) New directions for integrated weed management: modern technologies, tools and knowledge discovery. Advances in Agronomy, 155, pp. 243–319. doi.org/10.1016/bs.agron.2019.01.006.
- Partel, V., et al. (2019) Development and evaluation of a low-cost and smart technology for precision weed management utilizing artificial intelligence. Computers and Electronics in Agriculture, 157, pp. 339–350. doi.org/10.1016/j.compag.2018.12.048.
- Hunter, J. E., et al. (2020) Integration of remote-weed mapping and an autonomous spraying unmanned aerial vehicle for site-specific weed management. Pest Management Science, 76, pp. 1386–1392. doi.org/10.1002/ps.5651.
- Soltani, N., et al. (2016) Potential Corn yield losses from weeds in North America. Weed Technology, 30, pp. 979–984. doi.org/10.1614/WT-D-16-00046.1.
- Llewellyn, R., et al. (2016) Impact of Weeds on Australian Grain Production: the cost of weeds to Australian grain growers and the adoption of weed management and tillage practices Report for GRDC. CSIRO.
- Appleby, A.P., et al. (2001) Weed control. In Ullmann’s Encyclopedia of Industrial Chemistry, (Ed.). Available at: doi.org/10.1002/14356007.a28_165.
- Swanton, C. J., et al. (2015) Experimental methods for crop-weed competition studies. Weed Science, 63, pp. 2–11. doi.org/10.1614/WS-D-13-00062.1.
- EUSROSTAT. Statistics Eurostat. Available at: https://ec.europa.eu/eurostat/databrowser/view/aei_fm_salpest09/default/table?lang=en.
- Alexandratos, N & Bruinsma, J (2012) World agriculture towards 2030/2050: the 2012 revision.
- Ray, D. K., et al. (2013) Yield trends are insufficient to double global crop production by 2050. PLoS ONE, 8, p. e66428. doi.org/10.1371/journal.pone.0066428.
- Mall, R. K. (2017) 2—effect of climate change on agricultural crops. In: Dubey, S. K., Pandey, A., Sangwan RSBT-CD in B and B, Eds. Elsevier. p. 23–46.
- Westwood, J. H., et al. (2018) Weed management in 2050: perspectives on the future of weed science. Weed Science, 66, pp. 275–285. doi.org/10.1017/wsc.2017.78.
- Dong, W., et al. (2017) Impact of no tillage vs. conventional tillage on the soil bacterial community structure in a winter wheat cropping succession in northern China. European Journal of Soil Biology, 80, pp. 35–42. doi.org/10.1016/j.ejsobi.2017.03.001.
- Duke, S & Heap, I (2017) Evolution of weed resistance to herbicides: what have we learned after 70 years. Biology, Physiology and Molecular Biology of Weeds, pp. 63–86. doi.org/10.1201/9781315121031-6.
- Hicks, H. L., et al. (2018) The factors driving evolved herbicide resistance at a national scale. Nature Ecology & Evolution, 2, pp. 529–536. doi.org/10.1038/s41559-018-0470-1.
- Büchi, L. (2018) Importance of cover crops in alleviating negative effects of reduced soil tillage and promoting soil fertility in a winter wheat cropping system. Agriculture, Ecosystems & Environment, 256, pp. 92–104. doi.org/10.1016/j.agee.2018.01.005.
- Jackson, L., et al. (2003) Responses of soil microbial processes and community structure to tillage events and implications for soil quality. Geoderma, 114, pp. 305–317. doi.org/10.1016/S0016-7061(03)00046-6.
- Muola, A., et al. (2020) Risk in the circular food economy: glyphosate-based herbicide residues in manure fertilizers decrease crop yield. Science of The Total Environment, 750, p. 141422. doi.org/10.1016/j.scitotenv.2020.141422.
- Riemens, M. M., et al. (2007) Effect of stale seedbed preparations and subsequent weed control in lettuce (cv. Iceboll) on weed densities. Weed Research, 47, pp. 149–156. doi.org/10.1111/j.1365-3180.2007.00554.x.
- Bajwa, A. A., et al. (2017) Weed management using crop competition in Australia. Crop Protection, 95, pp. 8–13. doi.org/10.1016/j.cropro.2016.08.021.
- Swanton, C J & Weise, S F (1991) Integrated weed management: the rationale and approach. Weed Technology, 5, pp. 657–663. doi.org/10.1017/S0890037X00027512.
- Young, S. L., et al. (2017) Moving integrated weed management from low level to a truly integrated and highly specific weed management system using advanced technologies. Weed Research, 57, pp. 1–5. doi.org/10.1111/wre.12234.
- Bonny, S (2016) Genetically modified herbicide-tolerant crops, weeds, and herbicides: overview and impact. Environmental Management, 57, pp. 31–48. doi.org/10.1007/s00267-015-0589-7.
- Rose, M. T., et al. (2016) Impact of Herbicides on Soil Biology and Function. In: Sparks, D. L., Ed. Advances in Agronomy. Academic Press, pp. 133–220.
- Shannon, D., et al. (2018) An introduction to precision agriculture. Precision Agriculture Basics. Available at: doi.org/10.2134/precisionagbasics.2016.0084.
- Mahlein, A K (2016) Plant disease detection by imaging sensors—parallels and specific demands for precision agriculture and plant phenotyping. Plant Disease, 100, pp. 241–251. doi.org/10.1094/PDIS-03-15-0340-FE.
- Nawar, S., et al. (2017) Delineation of soil management zones for variable-rate fertilization: a review. Advances in Agronomy, 143, pp. 175–245. doi.org/10.1016/bs.agron.2017.01.003.
- Lama, G. F. C., et al. (2020) Evaluation of flow resistance models based on field experiments in a partly vegetated reclamation channel. Geosciences, 10, p. 47. doi.org/10.3390/geosciences10020047.
- Gautam, P. V., et al. (2019) Mechatronics application in precision sowing: a review. International Journal of Current Microbiology and Applied Sciences, 8, pp. 1793–1807. doi.org/10.20546/ijcmas.2019.804.208.
- Chlingaryan, A., et al. (2018) Machine learning approaches for crop yield prediction and nitrogen status estimation in precision agriculture: a review. Computers and Electronics in Agriculture, 151, pp. 61–69. doi.org/10.1016/j.compag.2018.05.012.
- Murray, S C (2017) Optical sensors advancing precision in agricultural production. Photonics Spectra.
- Dyshekov, A. I., et al. (2020) Principles of functioning of the autonomous device for weed control for precision agriculture. IOP Conference Series: Materials Science and Engineering. Available at: https://iopscience.iop.org/article/10.1088/1757-899X/747/1/012108.
- Duhan, J. S., et al. (2017) Nanotechnology: the new perspective in precision agriculture. Biotechnology Reports, 15, pp. 11–23. doi.org/10.1016/j.btre.2017.03.002.
- Mogili, U R & Deepak B B V L (2018) Review on application of drone systems in precision agriculture. Procedia Computer Science, 133, pp. 502–509. doi.org/10.1016/j.procs.2018.07.063.
- Raj, R., et al. (2020) Precision agriculture and unmanned aerial vehicles (UAVs) BT unmanned aerial vehicle: applications in agriculture and environment. In: Avtar, R., Watanabe, T., Eds. Cham: Springer International Publishing.
- Krishna KR (2018) Agricultural drones: a peaceful pursuit. Taylor Francis.
- John, K. N., et al. (2020) Weed mapping technologies in discerning and managing weed infestation levels of farming systems. Research on Crops, 21, pp. 93–98. doi.org/10.31830/2348-7542.2020.015.
- Lottes, P., et al. (2017) UAV-based crop and weed classification for smart farming. Proceedings of International Conference on Robotics and Automation. doi.org/10.1109/ICRA.2017.7989347.
- López-Granados, F., et al. (2016) Early season weed mapping in sunflower using UAV technology: variability of herbicide treatment maps against weed thresholds. Precision Agriculture, 17, pp. 183–199. doi.org/10.1007/s11119-015-9415-8.
- Jiménez-Brenes, F. M., et al. (2019) Automatic UAV-based detection of Cynodon dactylon for site-specific vineyard management. PLoS ONE. doi.org/10.1371/journal.pone.0218132.
- Daponte, P., et al. A review on the use of drones for precision agriculture. IOP Conference Series: Earth and Environmental Science, 275, p. 012022. Available at: https://iopscience.iop.org/article/10.1088/1755-1315/275/1/012022.
- Radoglou-Grammatikis, P., et al. (2020) A compilation of UAV applications for precision agriculture. Computer Networks, 172, p. 107148. doi.org/10.1016/j.comnet.2020.107148.
- Mancini, A., et al. (2019) Satellite and UAV data for Precision Agriculture Applications. In: 2019 International Conference on Unmanned Aircraft Systems (ICUAS). IEEE.
- Brook, A., et al. (2020) A smart multiple spatial and temporal resolution system to support precision agriculture from satellite images: proof of concept on Aglianico vineyard. Remote Sensing of Environment, 240, p. 111679. doi.org/10.1016/j.rse.2020.111679.
- Watts, A. C., et al. (2012) Unmanned aircraft systems in remote sensing and scientific research: classification and considerations of use. Remote Sensing, 4, pp. 1671–1692. doi.org/10.3390/rs4061671.
- Manfreda, S., et al. (2018) On the use of unmanned aerial systems for environmental monitoring. Remote Sensing, 10, p. 641. doi.org/10.3390/rs10040641.
- Anderson, K & Gaston, K J (2013) Lightweight unmanned aerial vehicles will revolutionize spatial ecology. Frontiers in Ecology and the Environment, 11, pp. 138–146. doi.org/10.1890/120150.
- Whitehead, K & Hugenholtz, C H (2014) Remote sensing of the environment with small unmanned aircraft systems (UASs), part 1: a review of progress and challenges. Journal of Unmanned Vehicle Systems, 02, pp. 69–85. doi.org/10.1139/juvs-2014-0006.
- Torres-Sánchez, J., et al. (2014) Multi-temporal mapping of the vegetation fraction in early-season wheat fields using images from UAV. Computers and Electronics in Agriculture, 103, pp. 104–113. doi.org/10.1016/j.compag.2014.02.009.
- Jannoura, R., et al. (2015) Monitoring of crop biomass using true colour aerial photographs taken from a remote controlled hexacopter. Biosystems Engineering, 129, pp. 341–351. doi.org/10.1016/j.biosystemseng.2014.11.007.
- Motohka, T., et al. (2010) Applicability of green-red vegetation index for remote sensing of vegetation phenology. Remote Sensing, 2, pp. 2369–2387. doi.org/10.3390/rs2102369.
- Xue J & Su B (2017) Significant remote sensing vegetation indices: a review of developments and applications. Journal of Sensors. doi.org/10.1155/2017/1353691.
- Crimaldi, M., et al. (2020) Neural network algorithms for real time plant diseases detection using UAVs. In: Coppola, A., Di Renzo, G. C., Altieri, G., D’Antonio, P., Eds. Innovative Biosystems Engineering for Sustainable Agriculture, Forestry and Food Production, p. 827–835. doi.org/10.1007/978-3-030-39299-4_89.
- Pflanz, M., et al. (2018) Drone based weed monitoring with an image feature classifier. Julius-Kühn-Archiv, 458, pp. 379–384.
- Etienne, A & Saraswat, D (2019) Machine learning approaches to automate weed detection by UAV based sensors. In: Autonomous air and ground sensing systems for agricultural optimization and phenotyping IV. International Society for Optics and Photonics, p. 110080R.
- Wang, S., et al. (2018) A deep-learning-based low-altitude remote sensing algorithm for weed classification in ecological irrigation area. In: International Conference on Intelligent Technologies and Applications. Springer, Singapore. pp. 451–460.
- Bah, M. D., et al. (2018) Deep learning with unsupervised data labeling for weed detection in line crops in UAV images. Remote Sensing, 10, p. 1690. doi.org/10.3390/rs10111690.
- Hughes, D P & Salathe, M (2016) An open access repository of images on plant health to enable the development of mobile disease diagnostics. ArXiv151108060 Cs. Available at: http://arxiv.org/abs/1511.08060.
- Singh, D., et al. (2020) PlantDoc: a dataset for visual plant disease detection. In: Proceedings of the 7th ACM IKDD CoDS and 25th COMAD, pp. 249–253.
- Sa, I., et al. (2018) WeedMap: a large-scale semantic weed mapping framework using aerial multispectral imaging and deep neural network for precision farming. Remote Sensing, 10, pp. 1–25. doi.org/10.3390/rs10091423.
- Wspanialy, P., et al. (2020) An image labeling tool and agricultural dataset for deep learning. ArXiv200403351 Cs Eess. Available at: http://arxiv.org/abs/2004.03351.
- Micasense Inc. (2020) Automatic Calibration Panel Detection (QR Mode). MicaSense Knowledge Base. Available at: https://support.micasense.com/hc/en-us/articles/360018618774.
- Micasense Inc. (2020) Best practices: Collecting Data with MicaSense Sensors. MicaSense Knowledge Base. Available at: https://support.micasense.com/hc/en-us/articles/224893167.
- Laliberte, A. S., et al. (2011) Multispectral remote sensing from unmanned aircraft: image processing workflows and applications for rangeland environments. Remote Sensing, 3, pp. 2529–2551. doi.org/10.3390/rs3112529.
- Barrero, O & Perdomo, SA (2018) RGB and multispectral UAV image fusion for Gramineae weed detection in rice fields. Precision Agriculture, 19, pp. 809–822. doi.org/10.1007/s11119-017-9558-x.
- Candiago, S., et al. (2015) Evaluating multispectral images and vegetation indices for precision farming applications from UAV images. Remote Sensing, 7, p. 4026. doi.org/10.3390/rs70404026.
- Mazzia, V., et al. (2020) UAV and machine learning based refinement of a satellite-driven vegetation index for precision agriculture. Sensors, 20, p. 2530. doi.org/10.3390/s20092530.
- Brook, A & Ben-Dor, E (2015) Supervised Vicarious Calibration (SVC) of multi-source hyperspectral remote-sensing data. Remote Sensing, 7, pp. 6196–6223. doi.org/10.3390/rs70506196.
- Adao, T., et al. (2017) Hyperspectral imaging: a review on UAV-based sensors, data processing and applications for agriculture and forestry. Remote Sensing, 9, p. 1110. doi.org/10.3390/rs9111110.
- Hassanein, M & El-Sheimy, N (2018) An efficient weed detection procedure using low-cost UAV imagery system for precision agriculture applications. The International Archives of the Photogrammetry, Remote Sensing and Spatial Information Sciences, 42, pp. 181–187. doi.org/10.5194/isprs-archives-XLII-1-181-2018.
- Tsouros, D. C., et al. (2019) A review on UAV-based applications for precision agriculture. Information, 10, p. 349. doi.org/10.3390/info10110349.
- Maes, W H & Steppe, K (2019) Perspectives for remote sensing with unmanned aerial vehicles in precision agriculture. Trends in Plant Science, 24, pp. 152–164. doi.org/10.1016/j.tplants.2018.11.007.
- Fawakherji, M., et al. (2019) UAV Image based crop and weed distribution estimation on embedded GPU boards BT computer analysis of images and patterns. Berlin: Springer.
- Rasmussen, J., et al. (2019) Pre-harvest weed mapping of Cirsium arvense in wheat and barley with off-the-shelf UAVs. Precision Agriculture, 20, pp. 983–999. doi.org/10.1007/s11119-018-09625-7.
- Franco, C., et al. (2018) Automatic detection of thistle-weeds in cereal crops from aerial RGB images. Information Processing and Management of Uncertainty in Knowledge-Based Systems. Applications, pp. 441–452. doi.org/10.1007/978-3-319-91479-4_37.
- Louargant, M., et al. (2018) Unsupervised classification algorithm for early weed detection in row-crops by combining spatial and spectral information. Remote Sensing, 10, pp. 1–18. doi.org/10.3390/rs10050761.
- Huang, Y., et al. (2018) UAV low-altitude remote sensing for precision weed management. Weed Technology, 32, pp. 2–6. doi.org/10.1017/wet.2017.89.
- Hansen, K. D., et al. (2013) An autonomous robotic system for mapping weeds in fields. IFAC Proceedings Volumes, 8, pp, 217–224. doi.org/10.3182/20130626-3-AU-2035.00055.
- Louargant, M., et al. (2017) Weed detection by UAV: simulation of the impact of spectral mixing in multispectral images. Precision Agriculture, pp. 1–20. doi.org/10.1007/s11119-017-9528-3.
- López-Granados, F., et al. (2006) Using remote sensing for identification of late-season grass weed patches in wheat. Weed Science, 54, pp. 346–353. doi.org/10.1614/WS-05-54.2.346.
- Sanders, J. T., et al. (2019) Weed species differentiation using spectral reflectance land image classification. Proceedings Volume 11007, Advanced Environmental, Chemical, and Biological Sensing Technologies XV. Available at: doi.org/10.1117/12.2519306.
- Davis, S., et al. (2018) A Meta-analysis of Canada Thistle (Cirsium arvense) management. Weed Science, 66, pp. 548–557. doi.org/10.1017/wsc.2018.6.
- Alshallash KS (2018) Germination of weed species (Avena fatua, Bromus catharticus, Chenopodium album and Phalaris minor) with implications for their dispersal and control. Annals of Agricultural Sciences, 63, pp. 91–97. doi.org/10.1016/j.aoas.2018.05.003.
- De Castro, A. I., et al. (2018) An automatic random forest-OBIA algorithm for early weed mapping between and within crop rows using UAV imagery. Remote Sensing, 10, p. 285. doi.org/10.3390/rs10020285.
- Reddy, K. N., et al. (2014) Glyphosate-resistant and glyphosate-susceptible Palmer amaranth (Amaranthus palmeri S. Wats.): hyperspectral reflectance properties of plants and potential for classification. Pest Management Science, 70, 1910–1917. doi.org/10.1002/ps.3755.
- Lass, L. W., et al. (2005) A review of remote sensing of invasive weeds and example of the early detection of spotted knapweed (Centaurea maculosa) and babysbreath (Gypsophila paniculata) with a hyperspectral sensor. Weed Science, 53, pp. 242–251. doi.org/10.1614/WS-04-044R2.
- Tiwari, O., et al. (2019) An experimental set up for utilizing convolutional neural network in automated weed detection. Proceedings of 2019 International Conference on Internet of Things: Smart Innovation and Usages (IoT-SIU), pp. 1–6. doi.org/10.1109/IoT-SIU.2019.8777646.
- Tay, J. Y. L., et al. (2018) Reaching new heights: can drones replace current methods to study plant population dynamics? Plant Ecology, 219, pp. 1139–1150. doi.org/10.1007/s11258-018-0865-8.
- Sandino, J & Gonzalez, F (2018) A Novel Approach for invasive weeds and vegetation surveys using UAS and artificial intelligence. 2018 23rd International Conference on Methods & Models in Automation & Robotics (MMAR). doi.org/10.1109/MMAR.2018.8485874.
- Scherrer, B., et al. (2019) Hyperspectral imaging and neural networks to classify herbicide-resistant weeds. Journal of Applied Remote Sensing, 13, p. 1. https://doi.org/10.1117/1.JRS.13.044516.
- Svensgaard, J., et al. (2019) Can reproducible comparisons of cereal genotypes be generated in field experiments based on UAV imagery using RGB cameras? European Journal of Agronomy, 106, pp. 49–57. doi.org/10.1016/j.eja.2019.03.006.
- Garcia-Ruiz, F. J., et al. (2015) Sugar beet (Beta vulgaris L.) and thistle (Cirsium arvensis L.) discrimination based on field spectral data. Biosystems Engineering, 139, pp. 1–15. doi.org/10.1016/j.biosystemseng.2015.07.012.
- Shapira, U., et al. (2010) Weeds detection by ground-level hyperspectral data. ISPRS Archive Vol. XXXVIII, 38, pp. 27–33.
- Rasmussen, J., et al. (2013) Potential uses of small unmanned aircraft systems (UAS) in weed research. Weed Research, 53, pp. 242–248. doi.org/10.1111/wre.12026.
- Slaughter, D. C., et al. (2008) Multispectral machine vision identification of lettuce and weed seedlings for automated weed control. Weed Technology, 22, pp. 378–384. doi.org/10.1614/WT-07-104.1.
- Norasma, N., et al. (2013) Spectral discrimination of weeds using hyperspectral radiometry. In: 5th Asian Conference on Precision Agriculture.
- López-Granados, F., et al. (2008) Multispectral classification of grass weeds and wheat (Triticum durum) using linear and nonparametric functional discriminant analysis and neural networks. Weed Research, 48, pp. 28–37. doi.org/10.1111/j.1365-3180.2008.00598.x.
- de Castro, A. I., et al. (2017) Mapping Cynodon dactylon in vineyards using UAV images for site-specific weed control. Advances in Animal Biosciences, 8, pp. 267–271. doi.org/10.1017/S2040470017000826.
- Fawakherji, M., et al. (2019) UAV image based crop and weed distribution estimation on embedded GPU boards. Communications in Computer and Information Science, 1089, pp. 100–108. doi.org/10.1007/978-3-030-29930-9_10.
- Peña, J. M., et al. (2013) Weed mapping in early-season maize fields using object-based analysis of unmanned aerial vehicle (UAV) images. PLoS ONE, 8, pp. 1–11. doi.org/10.1371/journal.pone.0077151.
- Pantazi, X.-E., et al. (2016) Active learning system for weed species recognition based on hyperspectral sensing. Biosystems Engineering, 146, pp. 193–202. doi.org/10.1016/j.biosystemseng.2016.01.014.
- Gibson, D. J., et al. (2017) Can weeds enhance profitability? Integrating ecological concepts to address crop-weed competition and yield quality. Journal of Ecology, 105, pp. 900–904. doi.org/10.1111/1365-2745.12785.
- Millar, K. D. L., et al. (2011) Evaluation of physiological parameters for the prediction of seed yield and quality for soybean (Glycine max) plants grown in the presence of weed competition. Plant Biosystems, 145, pp. 1–11. doi.org/10.1080/11263504.2010.514129.
- Adeux, G., et al. (2019) Mitigating crop yield losses through weed diversity. Nature Sustainability, 2, pp. 1018–1026. doi.org/10.1038/s41893-019-0415-y.
- Millar, K., et al. (2007) Impact of interspecific competition on seed development and quality of five soybean cultivars. Australian Journal of Experimental Agriculture, 47, pp. 1455–1459. doi.org/10.1071/EA07034.
- Talaviya, T., et al. (2020) Implementation of artificial intelligence in agriculture for optimisation of irrigation and application of pesticides and herbicides. Artificial Intelligence in Agriculture, 4, pp. 58–73. doi.org/10.1016/j.aiia.2020.04.002.
- Lanz, B., et al. (2018) The expansion of modern agriculture and global biodiversity decline: an integrated assessment. Ecological Economics, 144, pp. 260–277. doi.org/10.1016/j.ecolecon.2017.07.018.
- Erisman, J. W., et al. (2016) Agriculture and biodiversity: a better balance benefits both. AIMS Agriculture and Food, 1, pp. 157–174. doi.org/10.3934/agrfood.2016.2.157.